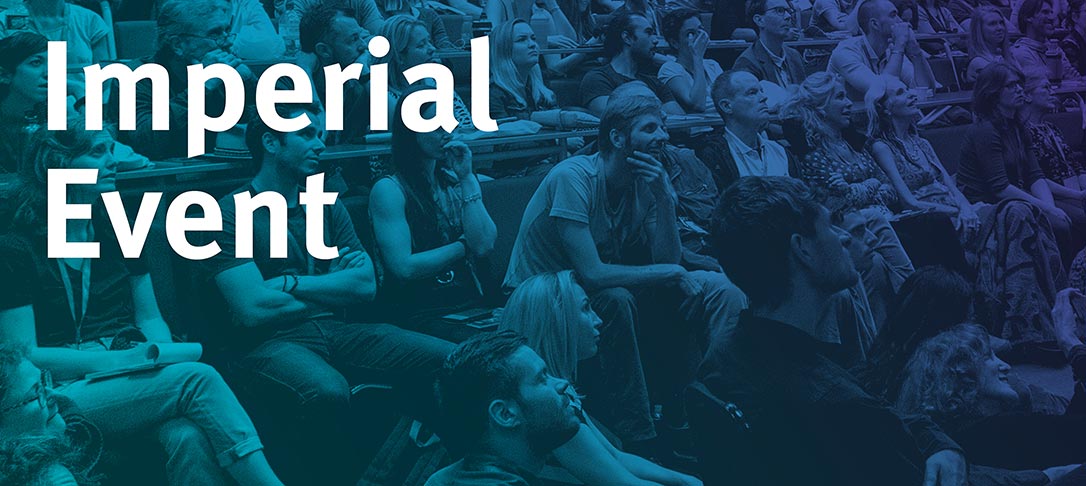
14:00 – 15:00 Marco Avella Medina:
Title: Differentially private inference via noisy optimization
Abstract: We propose a general optimization-based framework for computing differentially private M-estimators and a new method for the construction of differentially private confidence regions. Firstly, we show that robust statistics can be used in conjunction with noisy gradient descent and noisy Newton methods in order to obtain optimal private estimators with global linear or quadratic convergence, respectively. We establish global convergence guarantees, under both local strong convexity and self-concordance, showing that our private estimators converge with high probability to a neighborhood of the non-private M-estimators. The radius of this neighborhood is nearly optimal in the sense it corresponds to the statistical minimax cost of differential privacy up to a logarithmic term. Secondly, we tackle the problem of parametric inference by constructing differentially private estimators of the asymptotic variance of our private M-estimators. This naturally leads to the use of approximate pivotal statistics for the construction of confidence regions and hypothesis testing. We demonstrate the effectiveness of a bias correction that leads to enhanced small-sample empirical performance in simulations.
This is joint work with Casey Bradshaw and Po-Ling Loh.
15:30 – 16:30 Philip Ernst:
Title: New frontiers in statistical inference for stochastic processes
Abstract: I will first speak about how we recently resolved a longstanding open question in applied probability. Consider the standard empirical correlation $\rho_n$, which is defined for two related series of data of length $n$ using the standard Pearson correlation statistic. This empirical correlation is known as Yule’s “nonsense correlation” in honor of the British statistician G. Udny Yule, who in 1926 described the phenomenon by which empirical correlation fails to gauge independence of data series for random walks and for other time series. For the case of two independent and identically distributed random walks independent from each other, Yule empirically observed that the distribution of the empirical correlation is not concentrated around 0; rather, it is “volatile” in the sense that its distribution is heavily dispersed and is frequently large in absolute value. This well-documented effect was ignored by many scientists over the decades, up to the present day, even sparking recent controversies in climate-change attribution. Since the 1960s, some probabilists have wanted to eliminate any possible ambiguity about the issue by computing the variance of the continuous-time version $\rho$ of Yule’s nonsense correlation, based on the paths of two independent Wiener processes. The problem would remain open until we finally closed it in our work entitled “Yule’s `nonsense correlation’ solved!” (The Annals of Statistics, 2017).
I will then turn to speaking about our subsequent success in explicitly calculating all moments of $\rho$ for two independent Wiener processes. Our solution leads to the first approximation to the density of Yule’s nonsense correlation. We are also able to explicitly compute higher moments of Yule’s nonsense correlation when the two independent Wiener processes are replaced by two correlated Wiener processes, two independent Ornstein-Uhlenbeck processes, and two independent Brownian bridges. We then consider extending the definition of $\rho$ to the time interval $[0,T]$ for any $T>0$ and prove a Central Limit Theorem for the case of two independent Ornstein-Uhlenbeck processes. All of these aforementioned results appear in our 2022 preprint entitled “Yule’s `nonsense correlation’ solved: Part II” (under review, The Annals of Statistics, and available via https://www.stat.rice.edu/~pe6/YuleII.pdf).
Time permitting, I will then discuss our present work in building asymptotically exact and powerful tests of independence for pairs of independent Ornstein-Uhlenbeck processes and for other stationary Gaussian processes. Many of the methods of proof are drawn from Wiener chaos analysis, a simplified way of implementing the so-called Malliavin calculus for random variables depending in a polynomial way on finite or infinite dimensional Gaussian vectors such as Wiener processes. I also hope to speak about some initial leads in building tests of independence for pairs of nonstationary processes, in particular for processes with long memory such as the so-called fractional Brownian motion.
I will conclude with some concrete applications of our work to the study of weather and climate extremes. In particular, one pressing question of interest to our collaboration with the U.S. Office of Naval Research (ONR) and Royal Navy as follows: can the frequency of open ocean “freak wave” events observed by sea-faring vessels be correlated to and/or predicted and/or forecasted by sea-level rise and/or coastal wind extremes? This answer to this question should help inform the evaluation of risk exposure for Navy installations and vessels, particularly those based on the Atlantic seaboard; indeed, some naval installations may already be experiencing the recurrent effects of Atlantic storms, and are sure to be affected by major climate disruptions.
*I acknowledge, with thanks, the support of this research by the Office of Naval Research (ONR) award N00014-21-1-2672 (2021-2024) and a Royal Society Wolfson Fellowship (2022-2027).
*The first paper mentioned is joint work with Larry Shepp (deceased) and Abraham J. Wyner (The University of Pennsylvania). The second paper mentioned is joint work with L.C.G. Rogers (University of Cambridge) and my former postdoctoral fellow, Quan Zhou (Texas A&M University). Some of the present work is joint with Frederi Viens (Rice University).
Refreshments available between 15:00 – 15:30