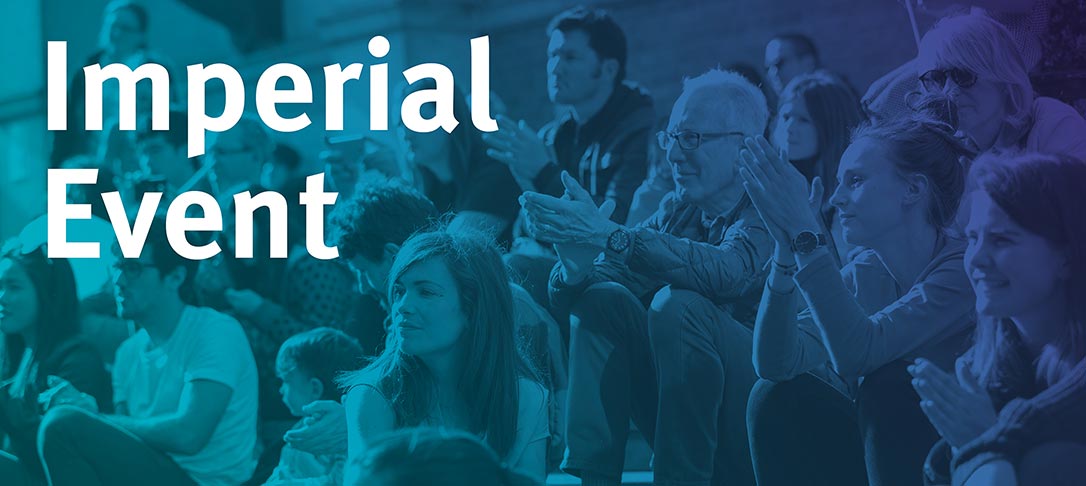
14:00 – 15:00 Helen Ogden
Title: Flexible models for longitudinal data
Abstract: I will discuss models for longitudinal data, where the data consists of noisy measurements taken at several different time points for each individual, and the aim is to model how each individual’s underlying response varies over time. If we assume linear variation of the responses over time, we could use a linear mixed model for this task. In this talk, I will discuss more flexible modelling approaches, which allow the variation of the response over time to be any smooth curve. There is a strong link with models for functional data, and I will describe previous work on adapting methods designed for functional data (where measurements are typically taken very frequently) to longitudinal data (with typically only a few measurements on each individual). I will describe some shortcomings of existing approaches in some examples, and describe new methodology to solve these problems.
15:30 – 16:30 Daniel Garcia Rasines
Title: Information splitting for selective inference
Abstract: In contemporary statistical applications, selection of the formal inferential problem is typically done after some level of interaction with the data. Usually, an initial exploratory analysis is used to identify interesting aspects of the population under study, and then the same dataset is used to learn about them. Such “data snooping” invalidates classical inferential procedures. Many approaches have been proposed to ensure inferential validity in these problems. In this talk, we will present a simple alternative to data splitting based on randomisation, which allows for higher selection and inferential power than the former and is applicable with an arbitrary selection rule. We provide theoretical and empirical comparisons of both methods, as well as a Central Limit Theorem for misspecified models.
Refreshments available between 15:00 – 15:30