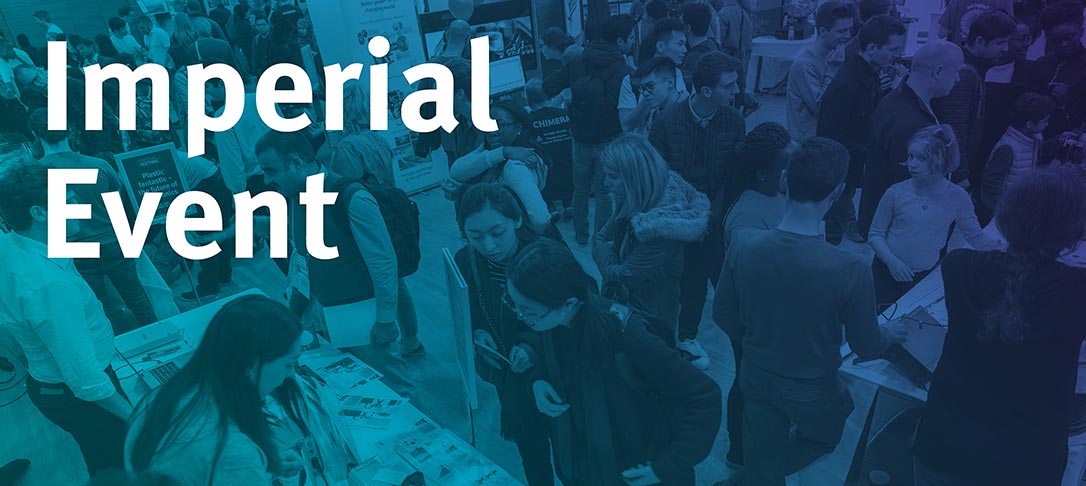
Title: Statistical Inference in Preferential Attachment Models and its connection to urn models
Abstract: After gaining its traction due to its ability to model power laws, the preferential attachment (PA) network is a popular way among probabilists and physicists to model the social networks, the collaboration networks and etc. The PA network model is an evolving network model where new nodes keep coming in. Each incoming new node establishes only one connection with one of the existing node. The probability to pick the node to connect to is proportional to preferences based on a PA function, mapping the natural numbers “degrees” to a positive real number “preference”. The PA function is assumed apriori non-decreasing, which means the nodes with high degrees are more likely to get new connections, i.e., “the rich get richer”. To solve the inverse problem of estimating the PA function statistically without relying on the historical evolution of the network, we propose a history-free maximum likelihood estimator (MLE). However, the lack of straightforward martingales in the history-free likelihood puts us in the blindfold in proving the central limit result for the said estimator. We draw an equivalence between the infinite dimensional evolution of PA networks (“something new”) and the finite dimensional urn model (“something old”), to overcome the impossibility of modeling the PA networks’ evolution with the existing operator theory, and to eventually prove the central limit theorem for the MLE. This is joint work with Aad van der Vaart.