The research focus is on developing state-of-the-art machine learning algorithms (including Deep learning, Reinforcement learning, and Unsupervised learning) for intelligent robot behaviour. We aim to advance the decisional autonomy of robots, improve their perception, their understanding of the world, and their prediction capabilities. The ultimate goal is to create algorithms that allow robots to autonomously learn from their environment in an open-ended and continuous way (i.e. lifelong learning).
We have several robots that we use to study cognition:
Robot DE NIRO - Design Engineering's Natural Interaction Robot.
SLIDER - a novel bipedal walking robot without knees.
ResQbot - an autonomous casualty extraction robot.
Lab members researching robot cognition: Fabio Pardo, Nemanja Rakicevic, Arash Tavakoli, Vitaly Levdik, Digby Chappell, Francesco Cursi.
Robot learns the skill of archery
Learning Symbolic Representations of Actions from Human Demonstrations
Interactive robot learning of visuospatial skills
Thruster Failure Recovery on Autonomous Underwater Vehicle
Publications
- Fabian Falck, Sagar Doshi, Marion Tormento, Gor Nersisyan, Nico Smuts, John Lingi, Kim Rants, Roni Permana Saputra, Ke Wang, Petar Kormushev, "Robot DE NIRO: A Human-Centered, Autonomous, Mobile Research Platform for Cognitively-Enhanced Manipulation", In Frontiers in Robotics and AI, 2020.
- Roni Permana Saputra, Nemanja Rakicevic, Petar Kormushev, "Sim-to-Real Learning for Casualty Detection from Ground Projected Point Cloud Data", In Proc. IEEE/RSJ Intl Conf. on Intelligent Robots and Systems (IROS 2019), Macau, China, 2019.
- Nemanja Rakicevic, Petar Kormushev, "Active Learning via Informed Search in Movement Parameter Space for Efficient Robot Task Learning and Transfer", In Autonomous Robots, Springer, 2019.
- Roni Permana Saputra, Petar Kormushev, "Casualty Detection from 3D Point Cloud Data for Autonomous Ground Mobile Rescue Robots", In Proc. 2018 IEEE International Symposium on Safety, Security, and Rescue Robotics (SSRR 2018), Philadelphia, USA, 2018.
- Roni Permana Saputra, Petar Kormushev, "Casualty Detection for Mobile Rescue Robots via Ground-Projected Point Clouds", In Proc. 19th International Conference Towards Autonomous Robotic Systems (TAROS 2018), Bristol, UK, 2018.
- Nemanja Rakicevic, Petar Kormushev, "Efficient Robot Task Learning and Transfer via Informed Search in Movement Parameter Space", In NIPS 2017 Workshop on Acting and Interacting in the Real World: Challenges in Robot Learning, 31st Conference on Neural Information Processing Systems (NIPS), California, USA, 2017.
Contact us
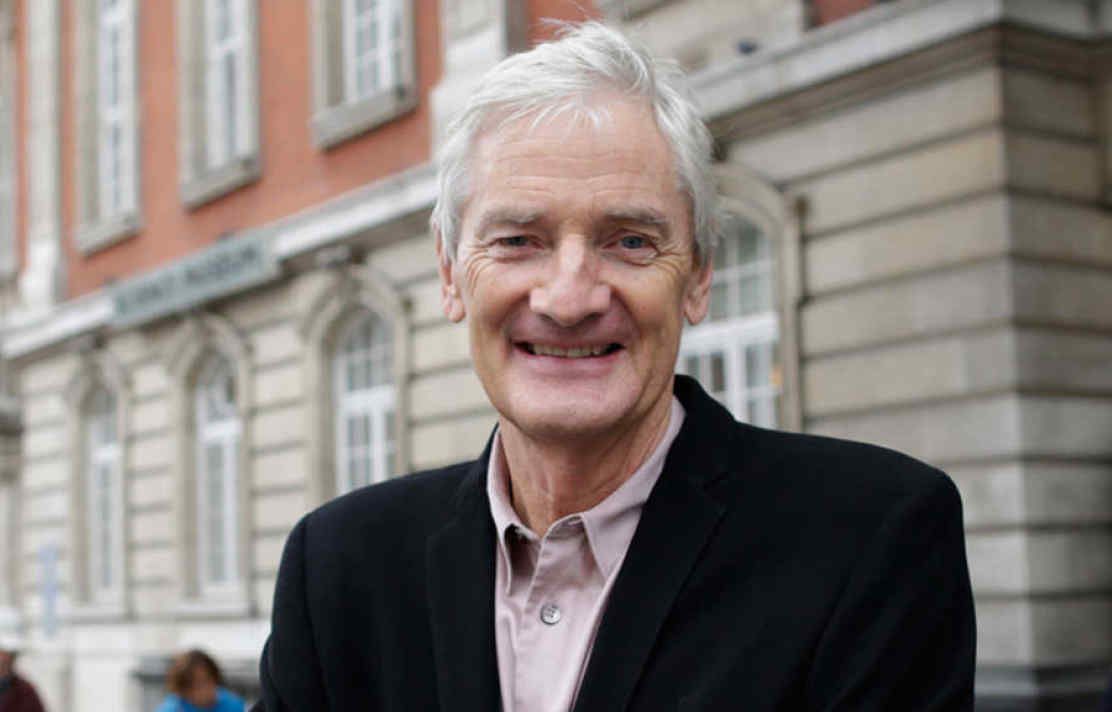