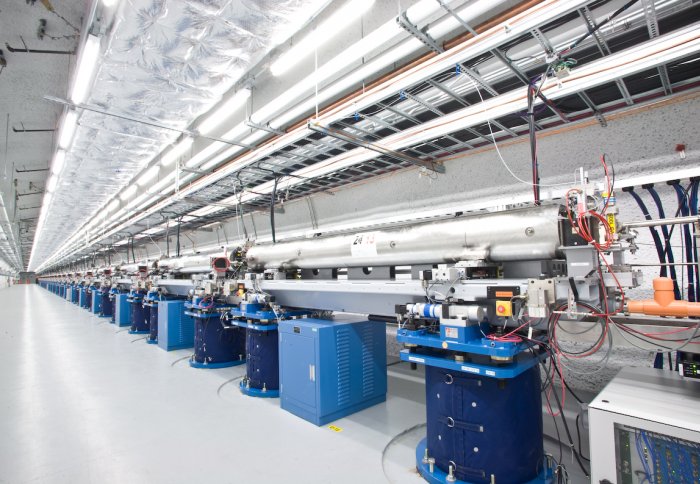
View of the Linac Coherent Light Source. Photo: SLAC National Accelerator Laboratory

Chemical reactions could be probed in even greater detail using a method invented by Imperial researchers that better characterises ultrafast x-rays.
X-rays can be used to investigate the structures of, and reactions between, molecules on very small scales and at high speed. To do this, scientists use free electron lasers (FELs) to create a train of x-ray pulses.
This allows researchers to probe some of the fundamental processes in chemistry and biology – such as the mechanisms of photosynthesis and the reactions of amino acids, which are the building blocks of life.
[Our method] will allow useful data to be gathered up to a thousand times faster.
– Alvaro Sanchez-Gonzalez
However, FELs are inherently unstable, meaning the properties of the resulting x-rays can vary from one pulse to the next. This can lead to inaccuracies in the measurements made using those x-rays.
There are methods to measure the actual properties of produced x-rays, but they can interfere with the experiment, and many will not be able to keep up with the very fast pulse rates of the next generation of x-ray FELs, such as the European XFEL in Hamburg (currently in testing) and the Linac Coherent Light Source II (LCLS-II) in the US.
Now, a research team led by physicists at Imperial College London have used an artificial intelligence technique known as machine learning to accurately predict the properties of x-rays. These predictions are based on certain measurements of the FEL, which can be performed fast enough to match the speed of x-rays.
A thousand times more data
The results of the study, involving 18 research institutions from the UK, Germany, Sweden, the US and Japan, are published today in Nature Communications.
Lead author of the new study Alvaro Sanchez-Gonzalez from the Department of Physics at Imperial said: “For current instruments, which generate about a hundred pulses per second, the slow nature of x-ray characterisation means that sometimes up to a half of the data is unusable.
"This problem will only be compounded in next-generation instruments, such as the European XFEL or LCLS-II, designed to generate hundreds of thousands of pulses per second.
“Our method effectively resolves the problem, and should work on the new instruments as well as the older ones we tested it on. This will allow useful data to be gathered up to a thousand times faster.”
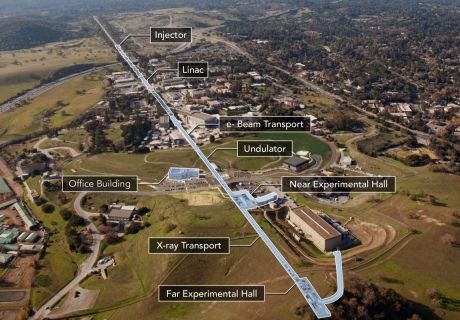
The setup of LCLS-I. Photo: SLAC National Accelerator Laboratory
The speed of the technique means chemical reactions could be explored in greater detail, as changes in the molecules could be observed on shorter timescales, down to single femtoseconds (one quadrillionth of a second).
Lead researcher Professor Jon Marangos from the Department of Physics at Imperial said: “These rapid-fire experiments will allow us to observe interactions that usually happen too fast for us to capture.
“They will also allow researchers to build up ‘molecular movies’ of these ultrafast process, for example to see how atoms and even the faster electrons move during a chemical reaction”
Predicting x-ray properties
The researchers knew that there were hundreds of variables in the FEL that could potentially be used to predict the x-ray properties, but it would take a long time to manually check each of these. So the team – which included final year MSci undergraduate students Paul Micaelli and Charles Olivier at Imperial – created a machine learning programme to do the work for them.
Machine learning involves software designed to trawl large datasets for patterns, build models, and then test predictions based on those models, improving as they go along. They used data from the SLAC National Accelerator Laboratory at Stanford University, US, to train some of these models to automatically find key variables and correlations that could be used to predict the x-ray properties with high accuracy.
The team hope their method could be installed directly into x-ray FEL instruments, allowing researchers around the world who access them to benefit from the greater data pool without applying the programme separately themselves.
-
'Accurate prediction of X-ray pulse properties from a free-electron laser using machine learning' by A. Sanchez-Gonzalez et al. is Published in Nature Communications.
Article text (excluding photos or graphics) available under an Attribution-NonCommercial-ShareAlike Creative Commons license.
Photos and graphics subject to third party copyright used with permission or © Imperial College London.
Reporter
Hayley Dunning
Communications Division

Contact details
Tel: +44 (0)20 7594 2412
Email: h.dunning@imperial.ac.uk
Show all stories by this author
Leave a comment
Your comment may be published, displaying your name as you provide it, unless you request otherwise. Your contact details will never be published.