

Last year four projects on machine learning were awarded a seed fund by the DSI. Ruth Misener and Marc Deisenroth talk about outcomes and future plans
To mark the launch of the new Machine Learning Lab in May 2018, the Data Science Institute awarded 4 seed funds of the overall value of £100k for pilot projects in the field of probabilistic modelling.
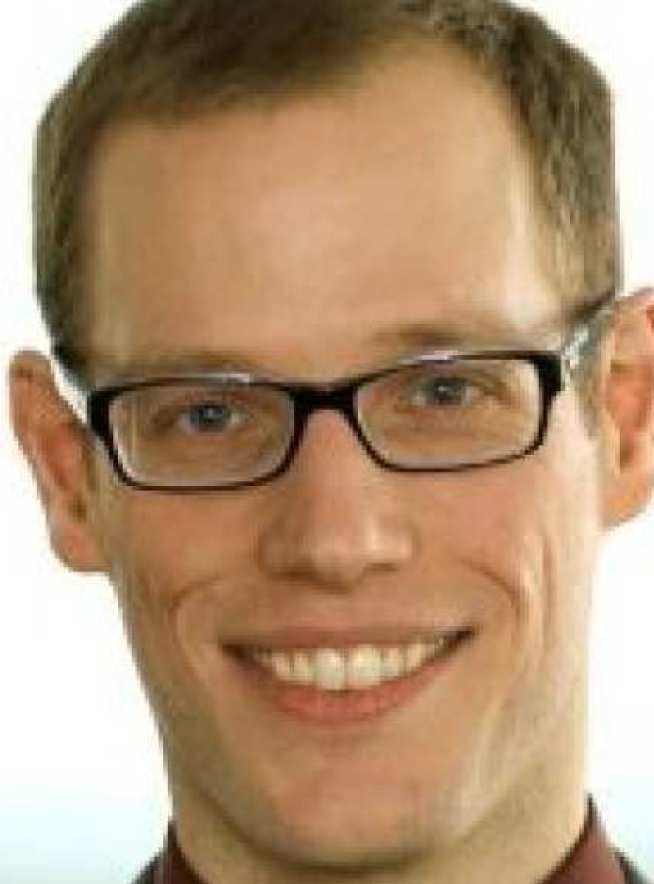
Marc Deisenroth, from the Department of Computing, got funding for a project on “Distributional robust adversarial training for natural language processing”
Thanks to the funding, they developed, in the words of Dr Deisenroth “a novel approach for high-dimensional optimization of black-box functions under the constraints of data efficiency”. This work is included in a publication accepted at Optimization Letters and a follow-up submitted to UAI, the Conference on Uncertainty in Artificial Intelligence in 2019. The team is also working on an open-source software package.
Ruth Misener, also from the Department of Computing, was awarded for her proposal to develop a methodology that “designs and controls an online experiment to identify the best model in a noisy environment incorporating measurement error and uncertain parameters”. She added that during the project they actually ended up considering “a wider range of uncertainty types than we originally thought”, incorporating uncertainty in states, uncertainty in controls, model parameter uncertainty, measurement noise, process noise, and a black-box transition function”.
The results of this work will be included in a manuscript to be submitted in June 2019. The team also developed an extension to their Python package for design of experiments for model discrimination that will be released when the paper will be submitted. Results will also be presented to the American Institute of Chemical Engineers (AIChE) Annual Meeting in November 2019.

Based on the findings of this DSI seed funding, they have applied in January 2019 to a joint EPSRC Prosperity Partnership application with pharmaceutical company Eli Lilly.
Dr Misener adds that “mostly this grant has allowed us to strengthen existing collaborations, e.g. with Bayer and Eli Lilly. This led to a grant submission. But our open source software has also inspired interest from academic contacts who are testing GPdoemd for their applications”.
Publications:
- Riccardo Moriconi, K. S. Sesh Kumar, Marc P. Deisenroth, High-dimensional Bayesian optimization with projections using quantile Gaussian processes, Optimization Letters, 2019
Article text (excluding photos or graphics) © Imperial College London.
Photos and graphics subject to third party copyright used with permission or © Imperial College London.
Reporter

Anna Cupani
Faculty of Engineering

Contact details
Email: press.office@imperial.ac.uk
Show all stories by this author