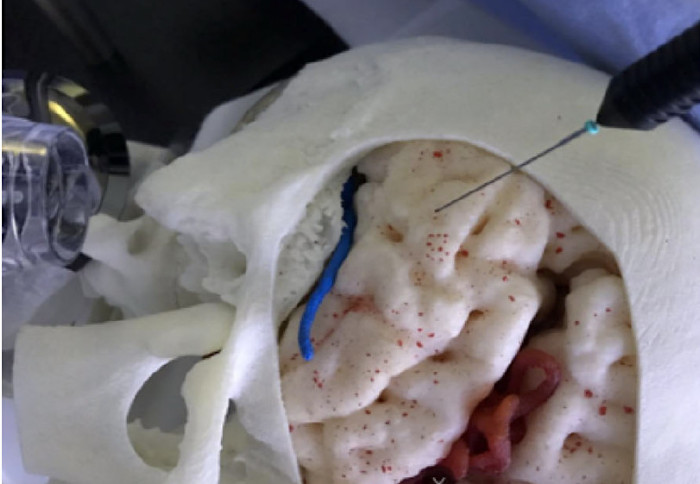

Our Hamlyn researchers proposed a transfer learning scheme for training a deep neural networks model, aiming to aid Robot-Assisted Microsurgery.
Micro-surgical robotic technologies have developed rapidly over the past few decades, which significantly improves the accuracy and dexterity in microsurgery.
Robot-Assisted Microsurgery (RAMS) can reduce blood loss and lead to fewer complications, shorter operating time, and lower treatment costs. Nevertheless, to realise the full potential of RAMS, microsurgical training is critical for surgical trainees to master the skills required to operate on patients.
However, the current assessment of microsurgical skills for RAMS still relies primarily on subjective observations and expert opinions. Subjective methods require an expert surgeon to score the performance of the trainee subjectively. The grading process of which is thus time-consuming, expensive and inconsistent due to the inherent biases in human interpretations.
In view of this, a general and automated evaluation method is desirable. Deep neural networks can be used for skill assessment through raw kinematic data, which has the advantages of being objective and efficient.
Yet, one of the major issues of deep learning for the analysis of surgical skills is that it requires a large database to train the desired model, which might result in a time-consuming training process.
A cross-domain transfer learning scheme for microsurgical skill assessment
Our researchers at the Hamlyn Centre proposed a transfer learning scheme for training a deep neural networks model with limited RAMS datasets for microsurgical skill assessment.
An in-house Microsurgical Robot Research Platform Database (MRRPD) was built with data collected from a microsurgical robot research platform (MRRP). It was used to verify the proposed cross-domain transfer learning for RAMS skill level assessment.
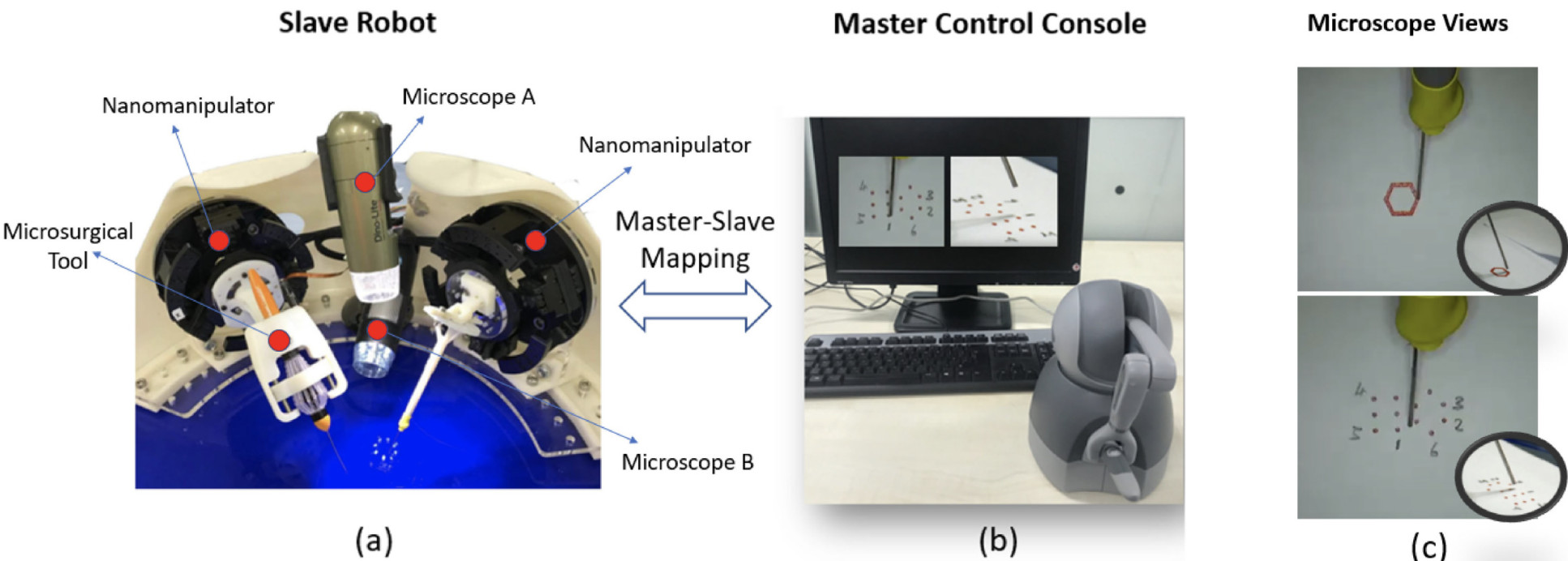
In order to carry out the implementation of transfer learning, our research team first reorganised the data that was collected via a publicly available database JIGSAWS (JHU-ISI Gesture and Skill Assessment Working Set) from eight right-handed subjects with three different skill levels by performing three surgical tasks (suturing, needle passing and knot tying) using the da Vinci surgical system.
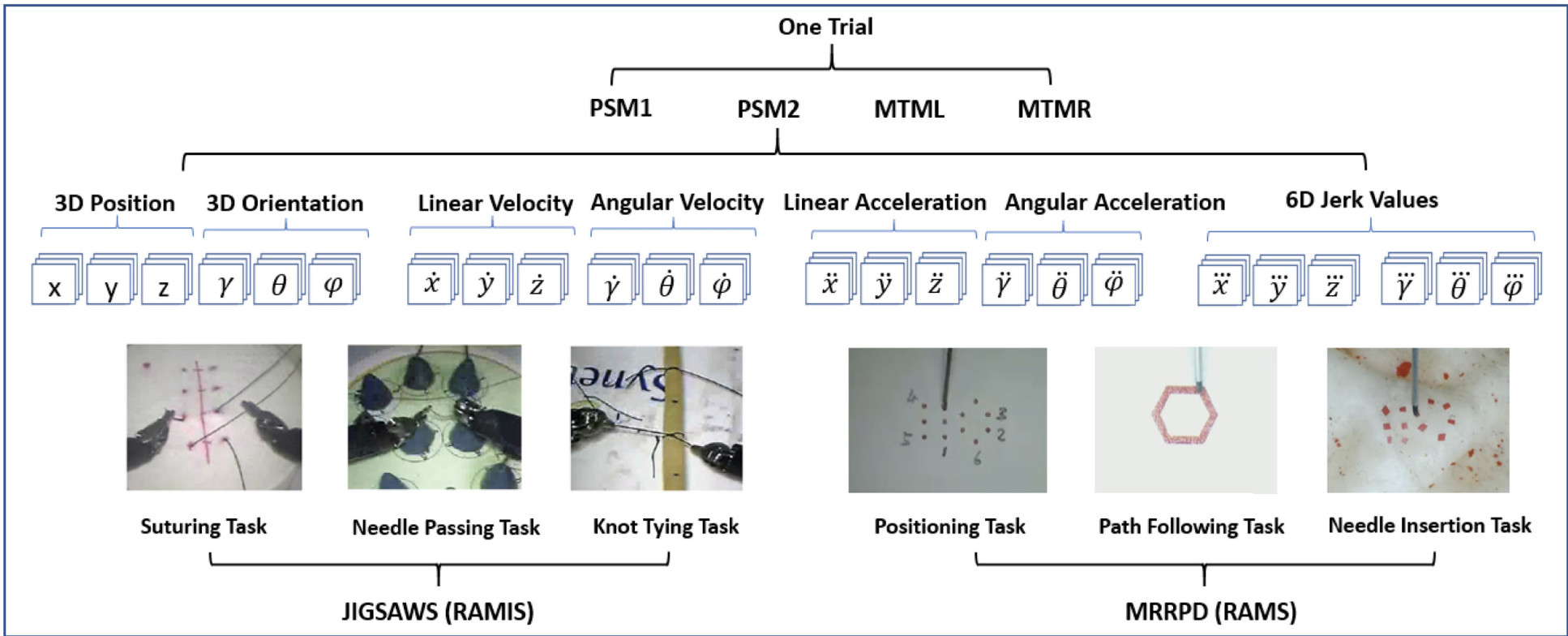
Following this, the knowledge gained from the JIGSAWS was transferred to MRRP, in order to accelerate the learning process of the model on microsurgical skill level classification. The model was fine-tuned after training with the data obtained from the MRRP.
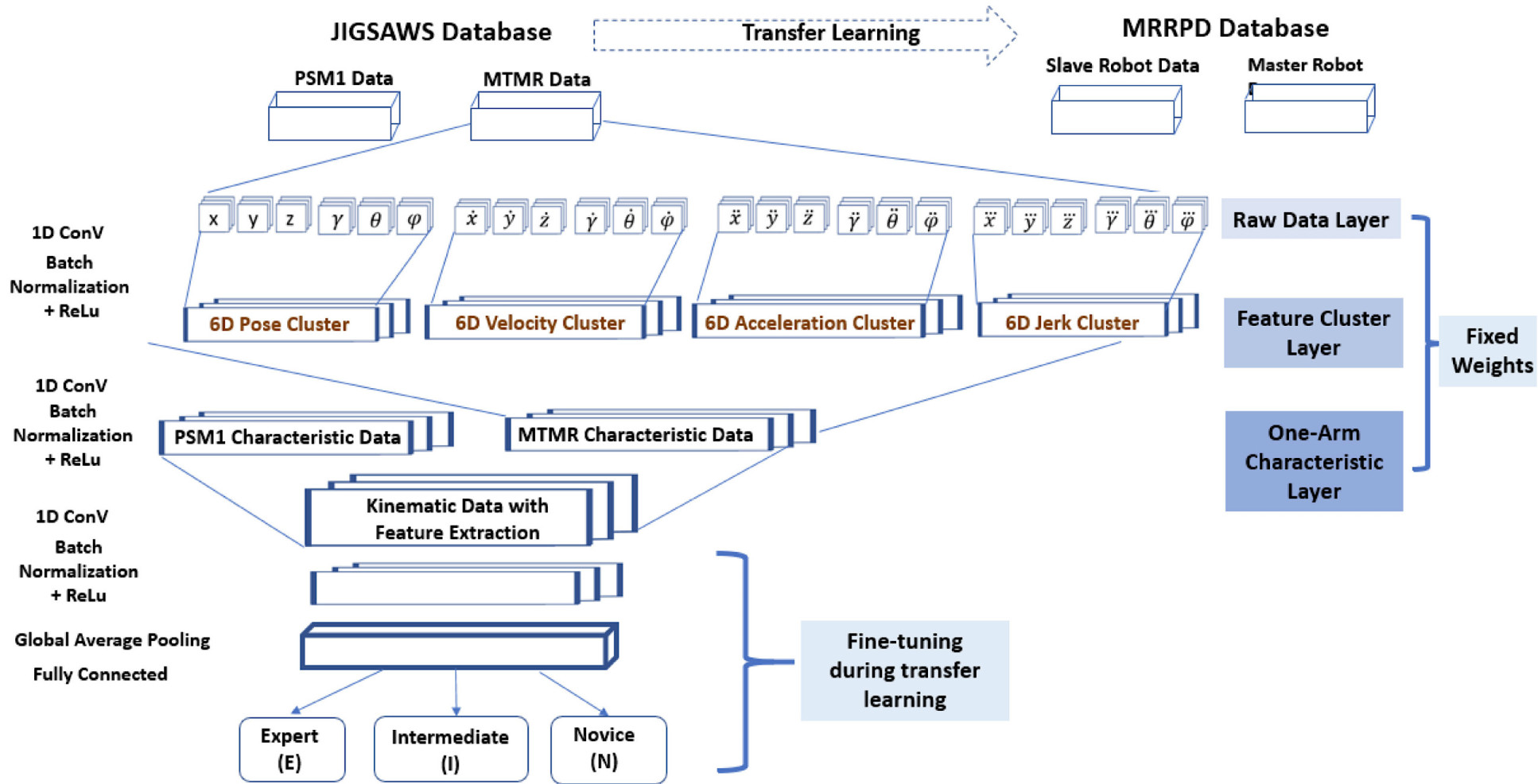
Moreover, microsurgical tool tracking was developed to provide visual feedback to the trainees, which guided them to gain higher level of skills. In addition, task-specific metrics and the other general evaluation metrics were also provided to the operators as a reference.
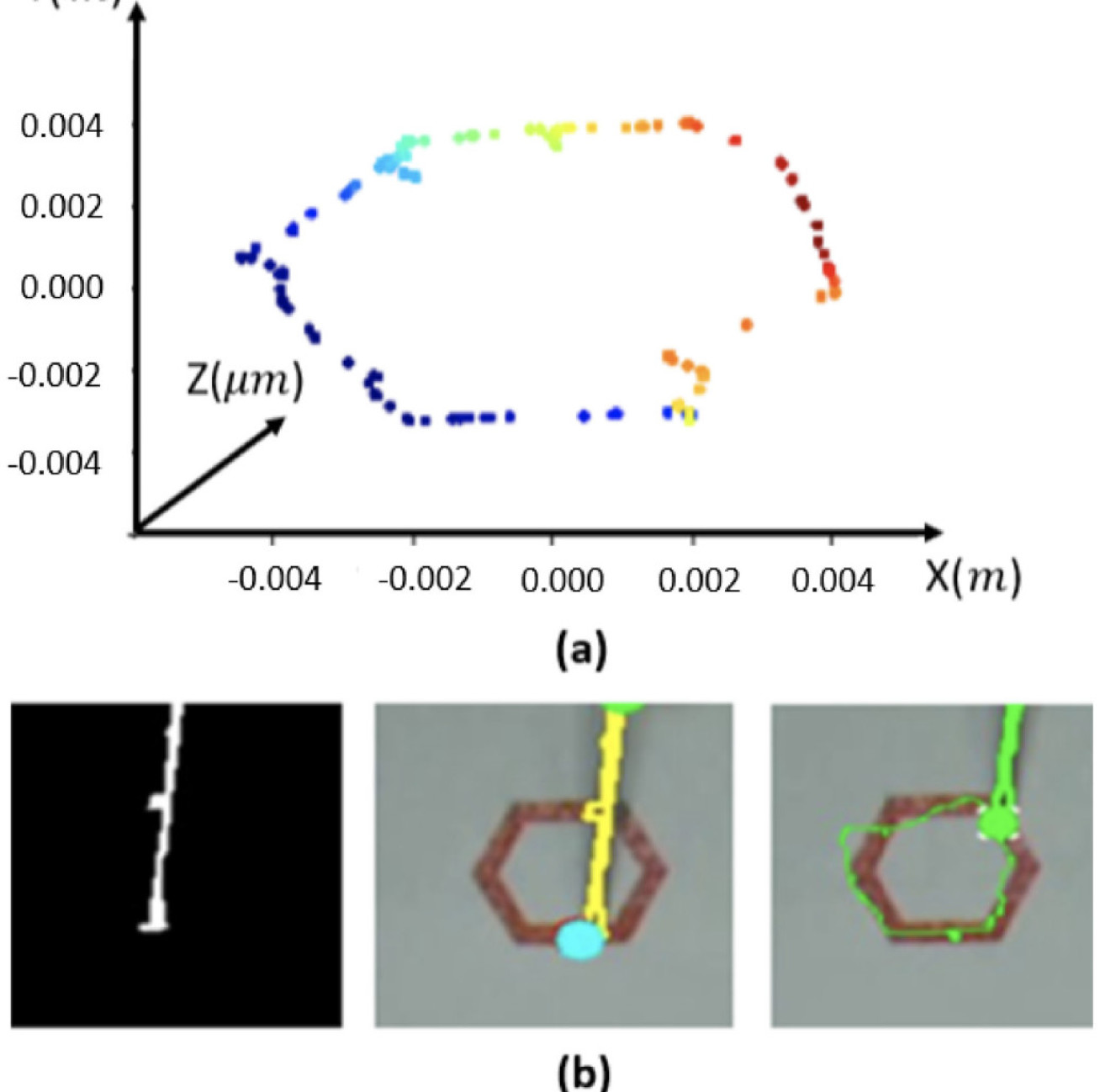
Based on the visual feedback, the operators could pay more attention to the practise of path following in that specific segments to accelerate the improvement of the overall operation performance.
In conclusion, our researcher team demonstrated that the transfer learning method is effective and potentially offers a valuable guidance for the operators to achieve a higher level of skills for microsurgical operation.
This research was supported by EPSRC Programme Grant “Micro-robotics for Surgery (EP/P012779/1)” (Zicong Wu, Junhong Chen, Anzhu Gao , Xu Chen, Peichao Li, Zhaoyang Wang, Guitao Yang, Benny Lo and , "Automatic Microsurgical Skill Assessment Based on Cross-Domain Transfer Learning", IEEE Robotics and Automation Letters, 5 (3), July 2020).
Supporters
Article text (excluding photos or graphics) © Imperial College London.
Photos and graphics subject to third party copyright used with permission or © Imperial College London.
Reporter
Erh-Ya (Asa) Tsui
Enterprise

Contact details
Tel: +44 (0)20 7594 8783
Email: e.tsui@imperial.ac.uk
Show all stories by this author