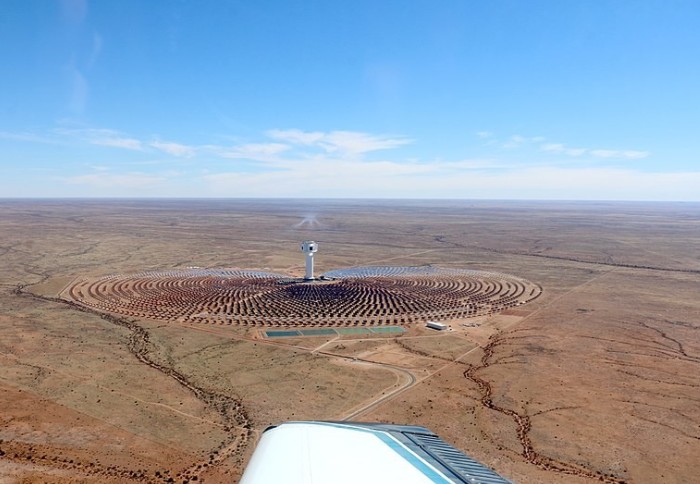

A Kenyan power sector study
By Dr Alexander Kell, Research Associate, Sustainable Gas Institute.
Knowing the best way to accelerate the long-term transition to renewable energy resources can be a difficult task. There are many uncertain variables at play, particularly over the 30+ year timeframes that we are analysing. What happens if onshore wind power costs don’t fall as fast as we expect or the price of gas spikes? These are some of the key questions that can be analysed using quantitative energy models such as MUSE, a novel agent-based model developed at the Sustainable Gas Institute (SGI) designed to answer these sorts of questions.
In this article we explore the benefits of using MUSE and compare it to another type of long-term energy model called OSeMOSYS applied to Kenya’s power sector. The primary question we want to answer is how much does the type of model affect the investments modelled in Kenya’s power sector? To help answer this question, we harmonised the models using the same input data and scenarios. For example, assuming the same technology costs and the same electricity demand profile between 2020 and 2050.
MUSE differs from OSeMOSYS in several ways. Firstly, MUSE is a simulation model, whereas OSeMOSYS is an optimisation model. This means that OSeMOSYS tries to minimise total energy costs over the full future horizon. This requires OSeMOSYS to know the technological costs in the future at the beginning of the simulation, known as perfect foresight. MUSE, however, relaxes this constraint and allows energy companies to invest based on imperfect future information, or not knowing what the cost of onshore wind will be in 10 years’ time, for instance. Secondly, MUSE is an agent-based model, which allows us to make different assumptions based on different types of investors, consumers, or both. OSeMOSYS, on the other hand, assumes that there is one central planner that makes cost-optimal decisions. Comparing these models is a difficult task as there is no known truth of what will happen in the future, therefore the best we can do is to compare outputs of case studies based on our knowledge of the assumptions.
Based on this harmonisation we run the models and get different outputs.
For both models the dominant power source over the future horizon is geothermal energy. This is used to meet the rapidly increasing electricity demand. Onshore wind grows for both models by 2050. However, a difference observed - there is a higher fraction of fossil fuel in MUSE as compared to OSeMOSYS. This may be due to decommissioning assumptions, where OSeMOSYS allows early retirement to reduce overall system costs, whereas in MUSE capacities do not become stranded.
Another difference is that MUSE invests more in biomass, which has a higher capacity factor, whereas OSeMOSYS invests more in other renewables (such as Solar PV, offshore wind and medium hydropower plants). This may be because the falling costs of renewables are known at the start of the simulation in OSeMOSYS, however, in MUSE, investors must react to the following price, causing a delay in their uptake.
These results show the differences in modelling assumptions for Kenya’s power sector. The models agree on the dominant technology being geothermal. However, there is a divergence in the renewable energy technologies invested in. This difference is explained by the underestimation of the falling prices of renewable technologies such as wind and solar, in MUSE, and biomass is therefore preferred.
Article text (excluding photos or graphics) © Imperial College London.
Photos and graphics subject to third party copyright used with permission or © Imperial College London.
Reporter

Emily Govan
Department of Life Sciences