

A new approach to producing realistic expressions of pain on robotic patients could help to reduce error and bias during physical examination.
A team led by researchers at Imperial College London has developed a way to generate more accurate expressions of pain on the face of medical training robots during physical examination of painful areas.
Findings, published today in Scientific Reports, suggest this could help teach trainee doctors to use clues hidden in patient facial expressions to minimise the force necessary for physical examinations.
The technique could also help to detect and correct early signs of bias in medical students by exposing them to a wider variety of patient identities.
Study author Sibylle Rérolle, from Imperial’s Dyson School of Design Engineering, said: “Improving the accuracy of facial expressions of pain on these robots is a key step in improving the quality of physical examination training for medical students.”
Understanding facial expressions: About the findings
In the study, undergraduate students were asked to perform a physical examination on the abdomen of a robotic patient. Data about the force applied to the abdomen was used to trigger changes in six different regions of the robotic face – known as MorphFace - to replicate pain-related facial expressions.
This method revealed the order in which different regions of a robotic face, known as facial activation units (AUs), must trigger to produce the most accurate expression of pain. The study also determined the most appropriate speed and magnitude of AU activation.
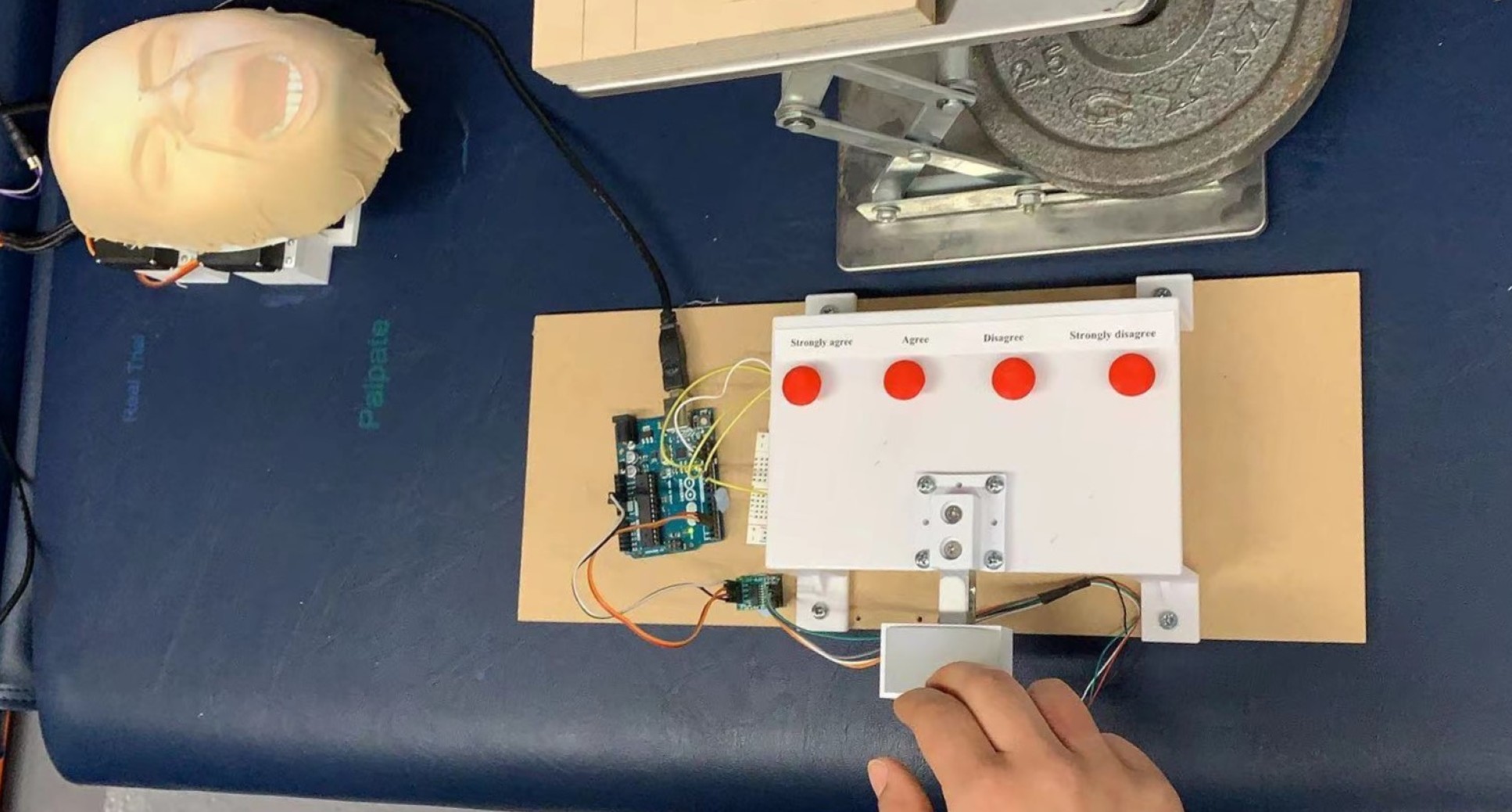
The researchers found that the most realistic facial expressions happened when the upper face AUs (around the eyes) were activated first, followed by the lower face AUs (around the mouth). In particular, a longer delay in activation of the Jaw Drop AU produced the most natural results.
The paper also found that how participants perceived the pain of the robotic patient was dependent on the gender and ethnic differences between the participant and the patient, and that these perception biases affected the force applied during physical examination.
For example, White participants perceived shorter delay facial expressions as most realistic on White robotic faces, whereas Asian participants perceived longer delays to be more realistic. This perception bias affected the force applied by White and Asian participants to different White robotic patients during examination, because participants applied more consistent force when they believed that the robot was showing realistic expressions of pain.
The importance of diversity in medical training simulators
When doctors conduct physical examination of painful areas, the feedback of patient facial expressions is important. However, many current medical training simulators cannot display real-time facial expressions relating to pain and include a limited number of patient identities in terms of ethnicity and gender.
The researchers say these limitations could cause medical students to develop biased practices, with studies already highlighting racial bias in the ability to recognise facial expressions of pain.
“Previous studies attempting to model facial expressions of pain relied on randomly generated facial expressions shown to participants on a screen,” said lead author Jacob Tan, also of the Dyson School of Design Engineering. “This is the first time that participants were asked to perform the physical action which caused the simulated pain, allowing us to create dynamic simulation models.”
Participants were asked to rate the appropriateness of the facial expressions from “strongly disagree” to “strongly agree”, and the researchers used these responses to find the most realistic order of AU activation.
Sixteen participants were involved in the study, made up of a mix of males and females of Asian and White ethnicities. Each participant performed 50 examination trials on each of four robotic patient identities – Black female, Black male, White female, White male.
Co-author Thilina Lalitharatne, from the Dyson School of Design Engineering, said: “Underlying biases could lead doctors to misinterpret the discomfort of patients - increasing the risk of mistreatment, negatively impacting doctor-patient trust, and even causing mortality.
“In the future, a robot-assisted approach could be used to train medical students to normalise their perceptions of pain expressed by patients of different ethnicity and gender.”
Next steps
Dr Thrishantha Nanayakkara, the director of Morph Lab, urged caution in assuming these results apply to other participant-patient interactions that are beyond the scope of the study.
He said: “Further studies including a broader range of participant and patient identities, such as Black participants, would help to establish whether these underlying biases are seen across a greater ranger of doctor-patient interactions.
“Current research in our lab is looking to determine the viability of these new robotic-based teaching techniques and, in the future, we hope to be able to significantly reduce underlying biases in medical students in under an hour of training.”

Read the full research paper: Simulating dynamic facial expressions of pain from visuo?haptic interactions with a robotic patient.
Article text (excluding photos or graphics) © Imperial College London.
Photos and graphics subject to third party copyright used with permission or © Imperial College London.
Reporter

Alex Dadswell
Centre for Languages, Culture and Communication

Contact details
Email: press.office@imperial.ac.uk
Show all stories by this author
Leave a comment
Your comment may be published, displaying your name as you provide it, unless you request otherwise. Your contact details will never be published.