Videos
Getting Robots In The Future To Truly See
Developing robots that can process visual information in real-time could lead to a new range of handy and helpful robots for around the home and in industry. Professor Andrew Davison and Dr Stefan Leutenegger from the Dyson Robotics Lab at Imperial College London discuss the advances they are making in developing robotic vision.
2021 Widget
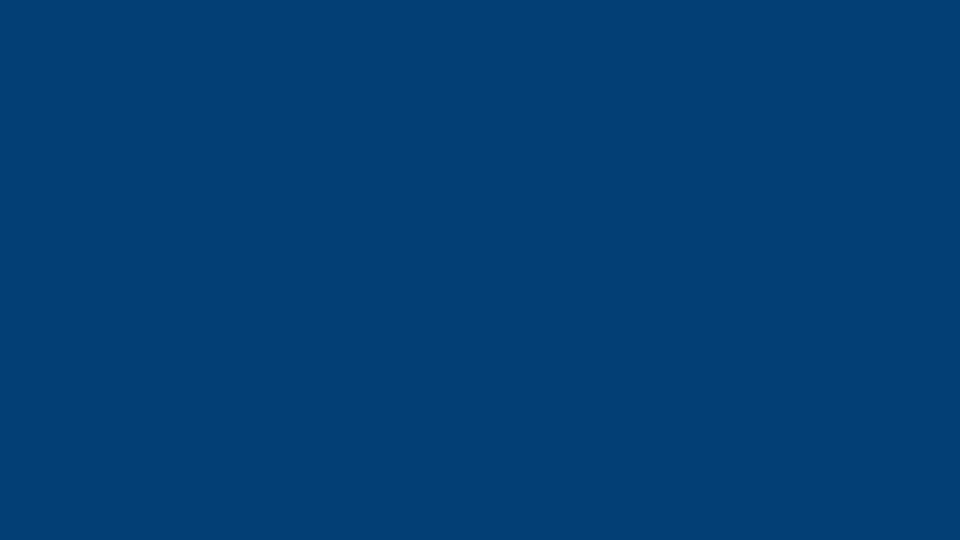
CodeMapping
CodeMapping: Real-Time Dense Mapping for Sparse SLAM using Compact Scene Representations
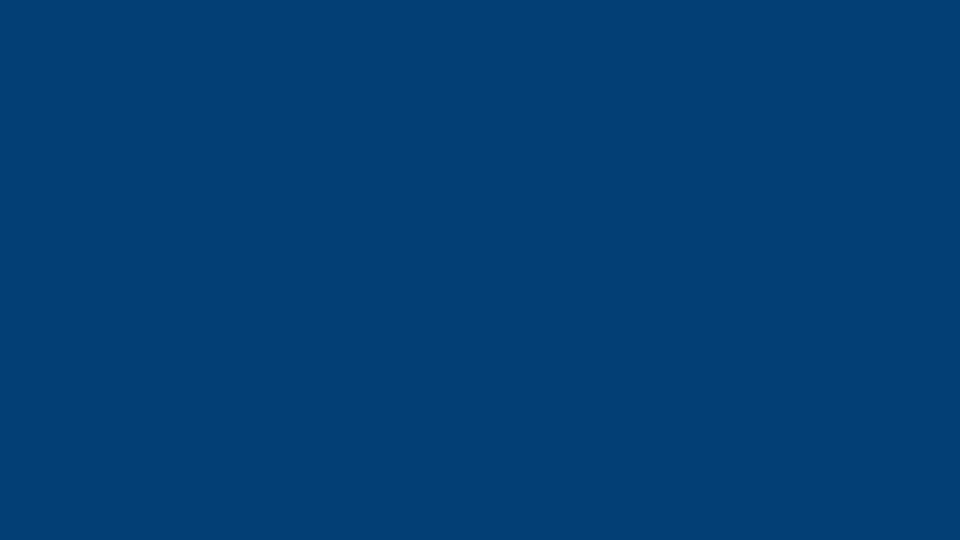
End-to-End Egospheric Spatial Memory
End-to-End Egospheric Spatial Memory
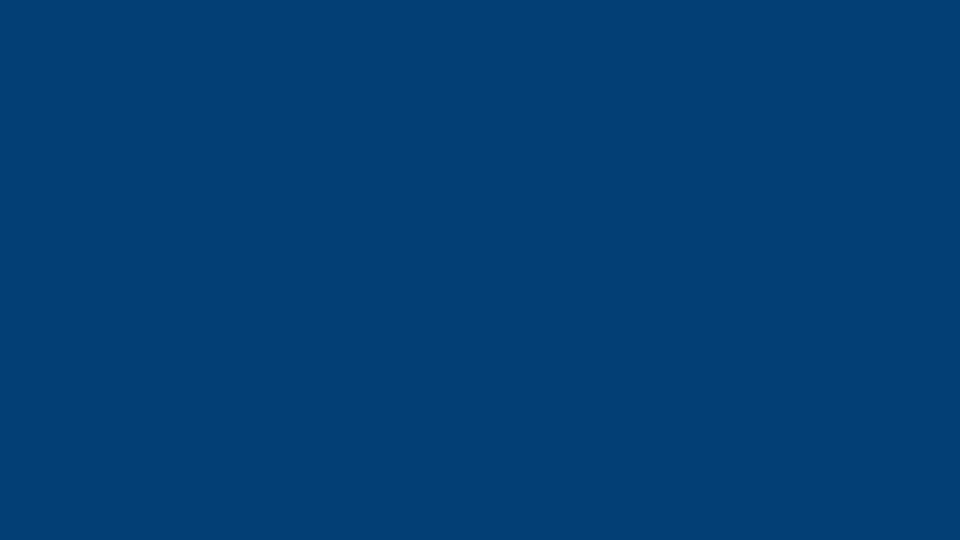
iMAP
iMAP: Implicit Mapping and Positioning in Real-Time
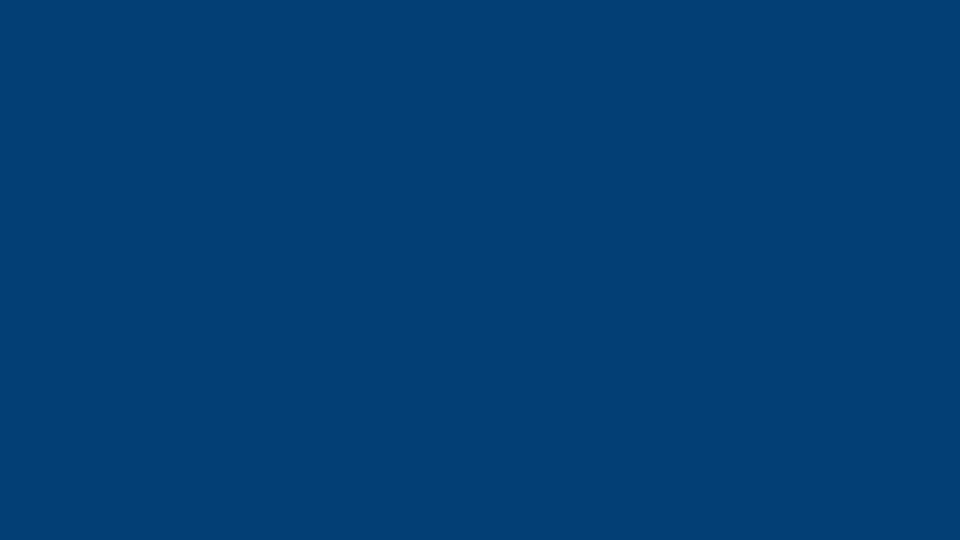
In-Place Scene Labelling
In-Place Scene Labelling and Understanding with Implicit Scene Representation
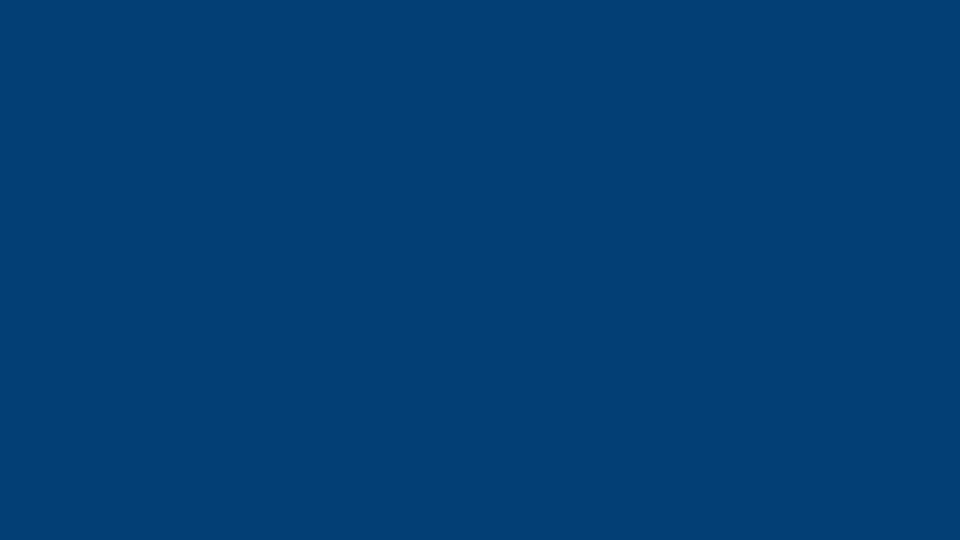
NodeSLAM
NodeSLAM: Neural Object Descriptors for Multi-View Shape Reconstruction
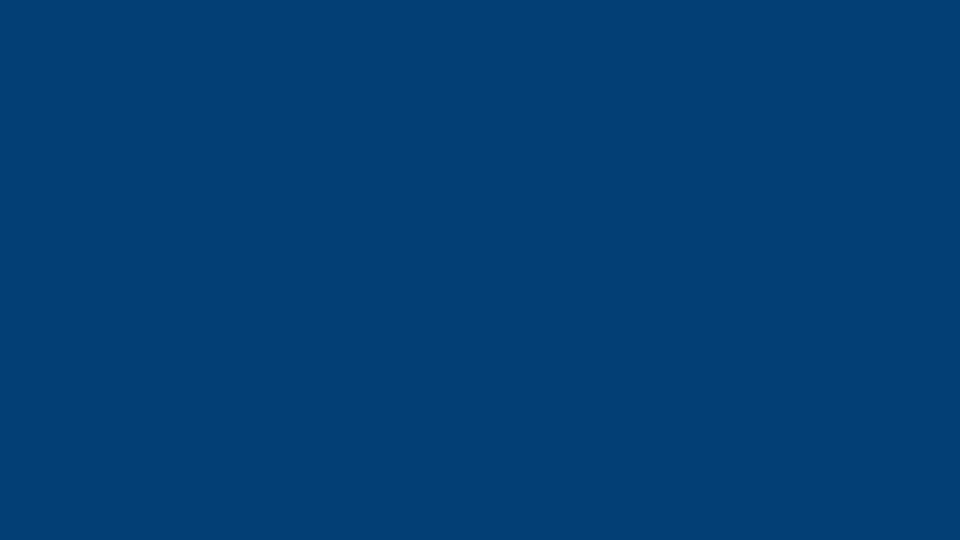
SIMstack
SIMstack: A Generative Shape and Instance Model for Unordered Object Stacks
2020 Widget
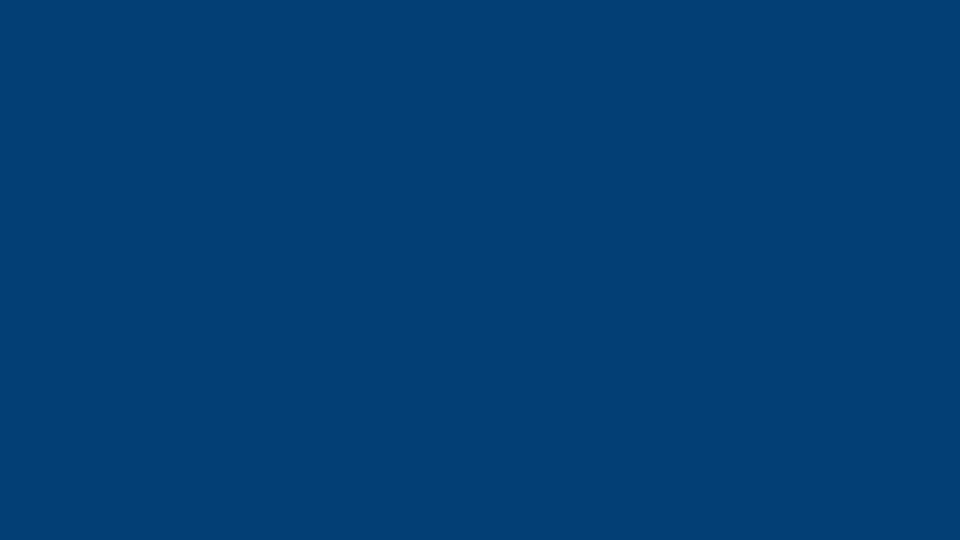
MoreFusion
Comparing View-Based and Map-Based Semantic Labelling in Real-Time SLAM
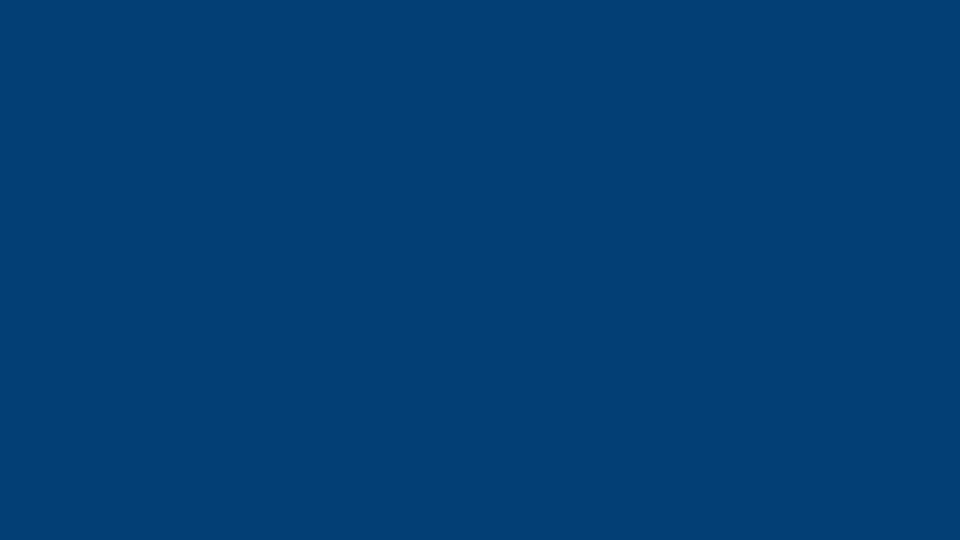
DeepFactors
DeepFactors: Real-Time Probabilistic Dense Monocular SLAM
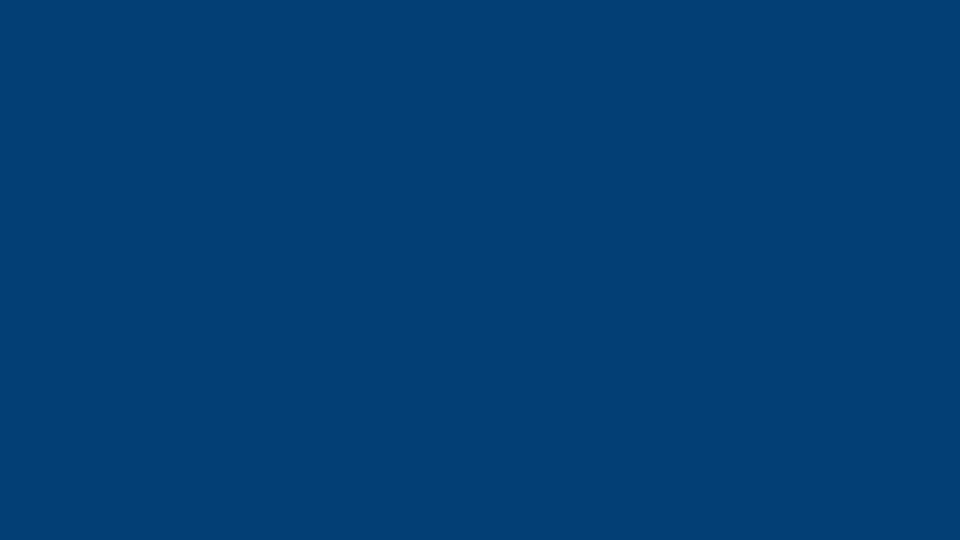
Learning One-shot Imitation
Learning One-Shot Imitation from Humans without Humans
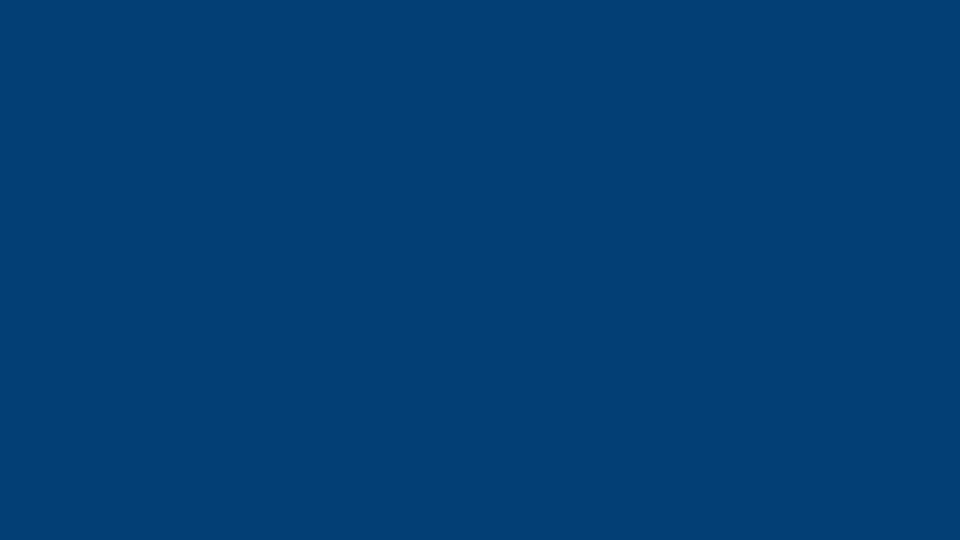
MoreFusion
MoreFusion: Multi-object Reasoning for 6D Pose Estimation from Volumetric Fusion
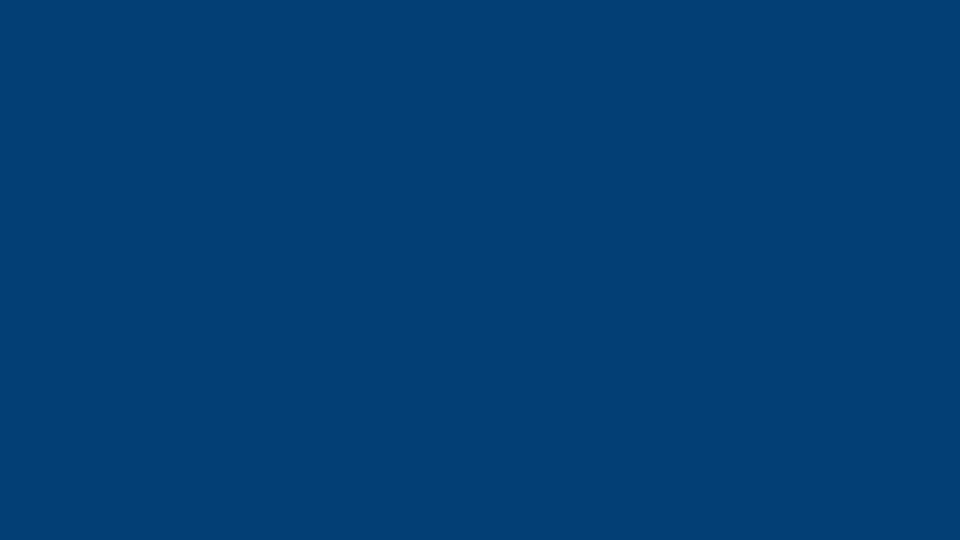
RLBench
RLBench: The Robot Learning Benchmark
2019 Widget
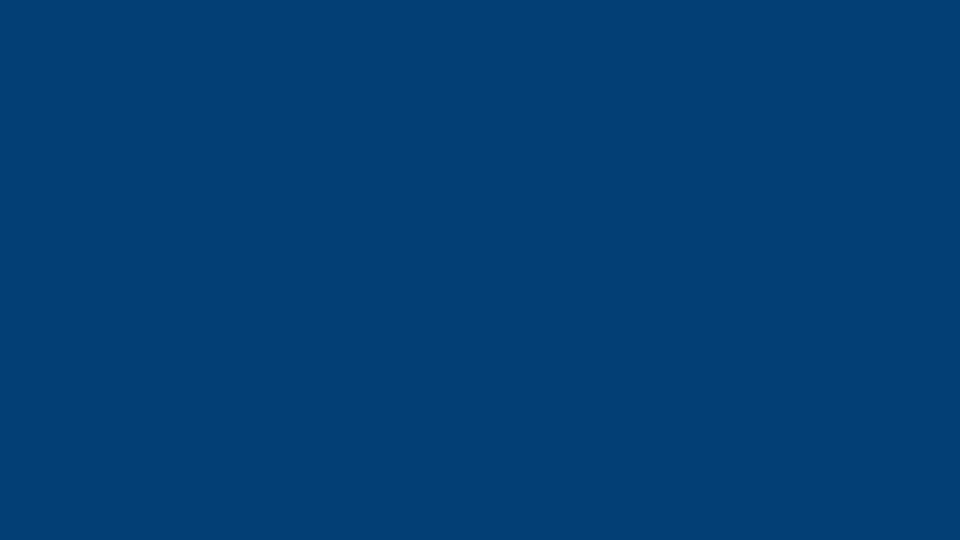
KO Fusion
KO Fusion: Dense Visual SLAM with TIghtly-Coupled Kinematic and Odometric Tracking
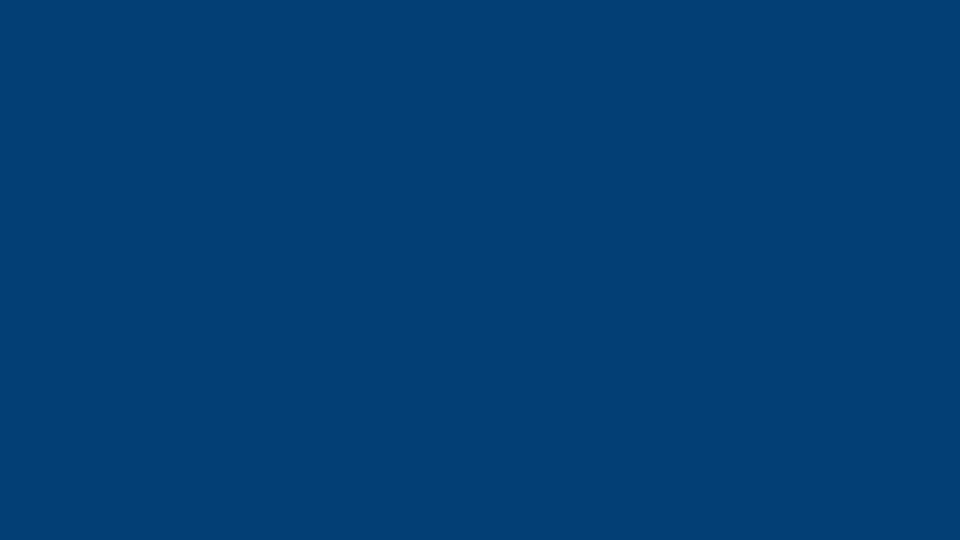
Learning Meshes for Dense Visual SLAM
Learning Meshes for Dense Visual SLAM
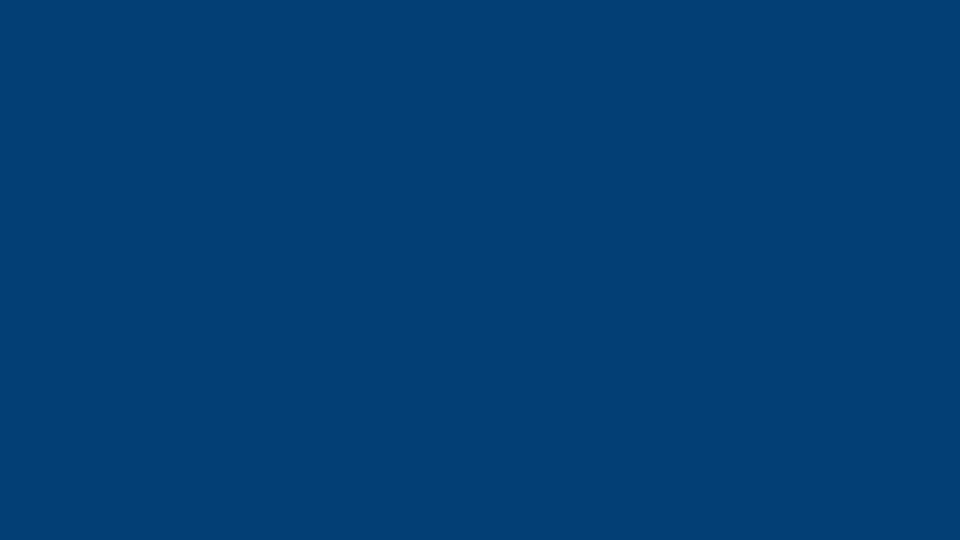
SceneCode
SceneCode: Monocular Dense Semantic Reconstruction using Learned Encoded Scene Representations
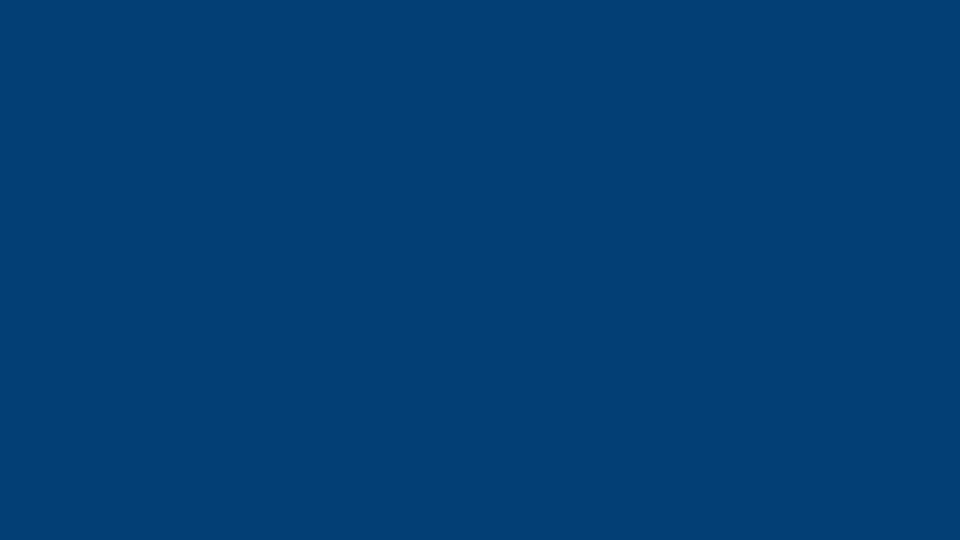
X-Section
X-Section: Cross-Section Prediction for Enhanced RGB-D Fusion
2018 Widget
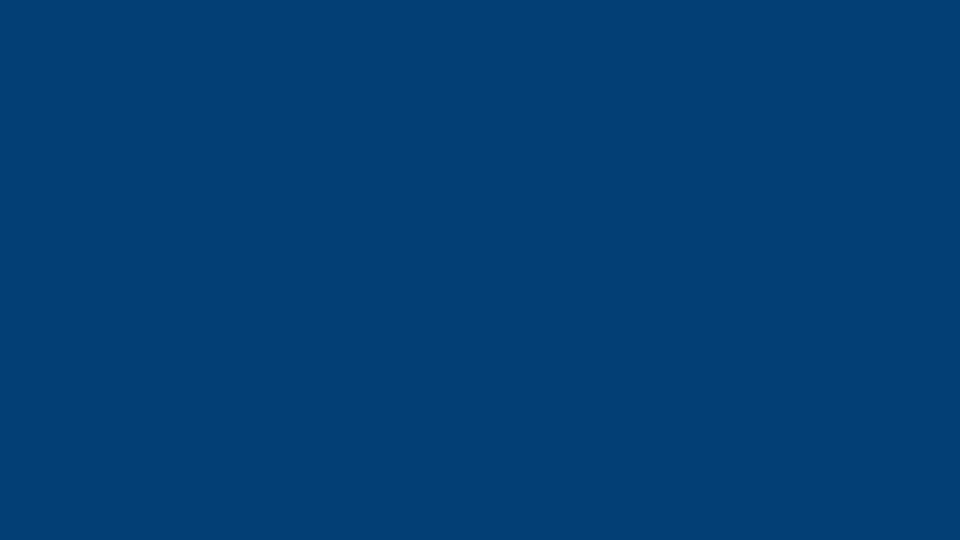
CodeSLAM
CodeSLAM - Learning a Compact, Optimisable Representation for Dense Visual SLAM.
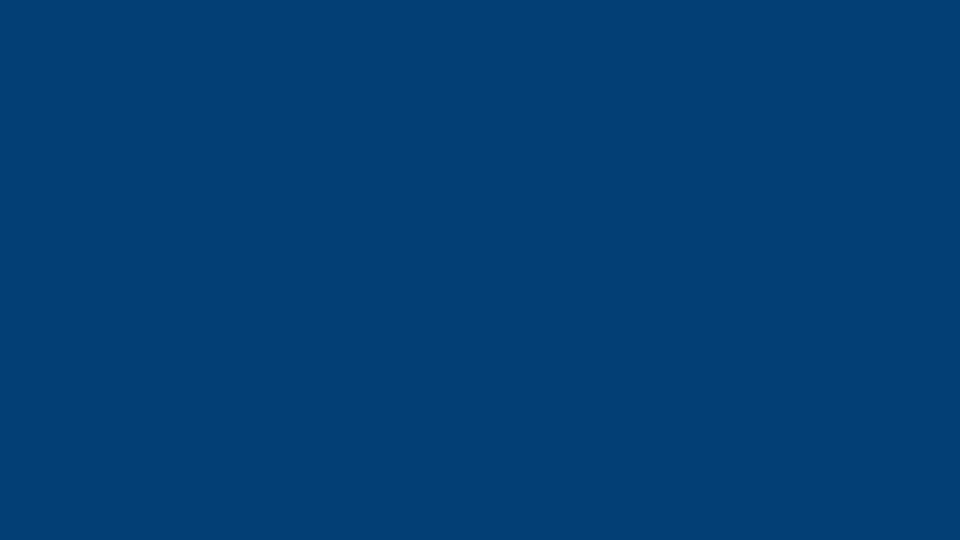
Few-Shot Imitation Learning
Task-Embedded Control Networks for Few-Shot Imitation Learning
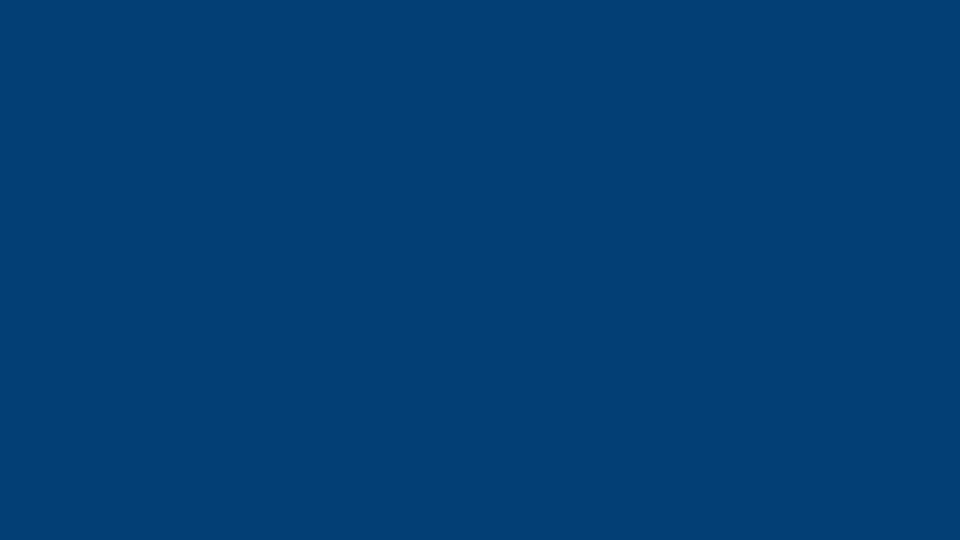
Fusion++
Fusion++: Volumetric Object-Level SLAM
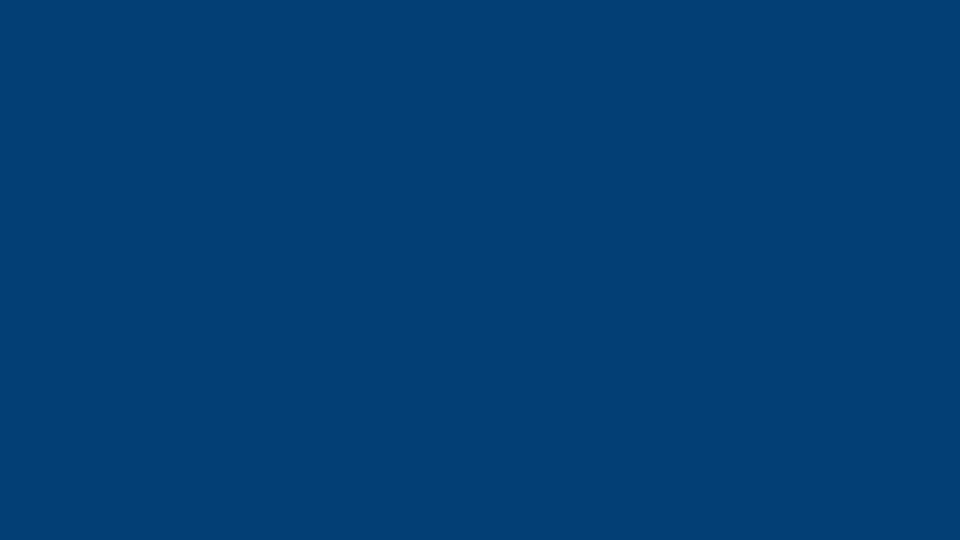
LS-Net
LS-Net: Learning to Solve Nonlinear Least Squares for Dense Tracking and Mapping
2017 Widget
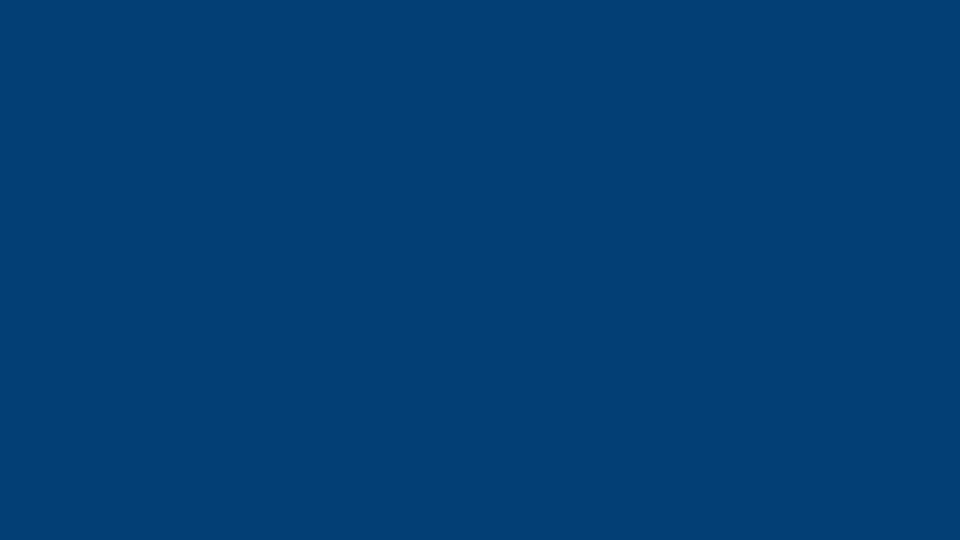
Dense RGB-D-Inertial SLAM with Map Deformations
Dense RGB-D-Inertial SLAM with Map Deformations
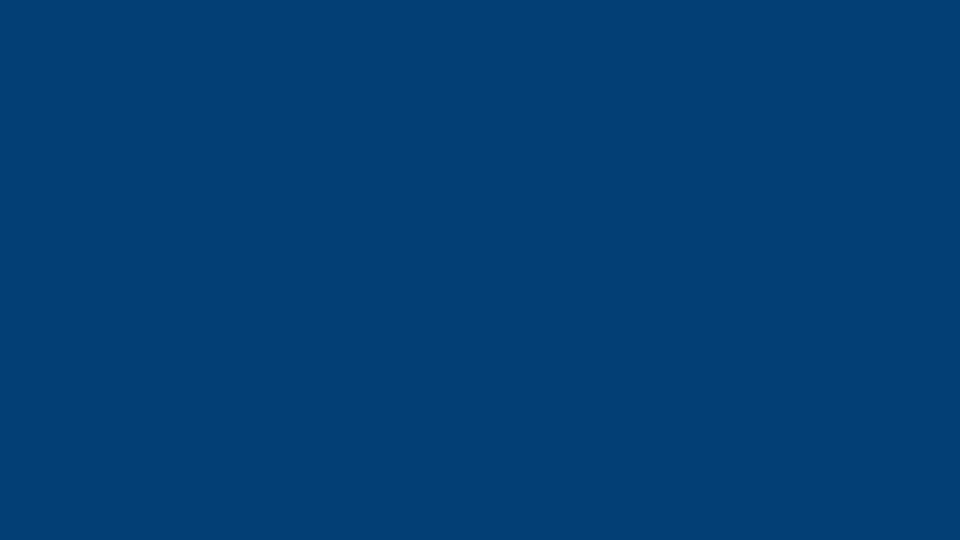
Multi-Stage Task
Transferring End-to-End Visuomotor Control from Simulation to Real World for a Multi-Stage Task
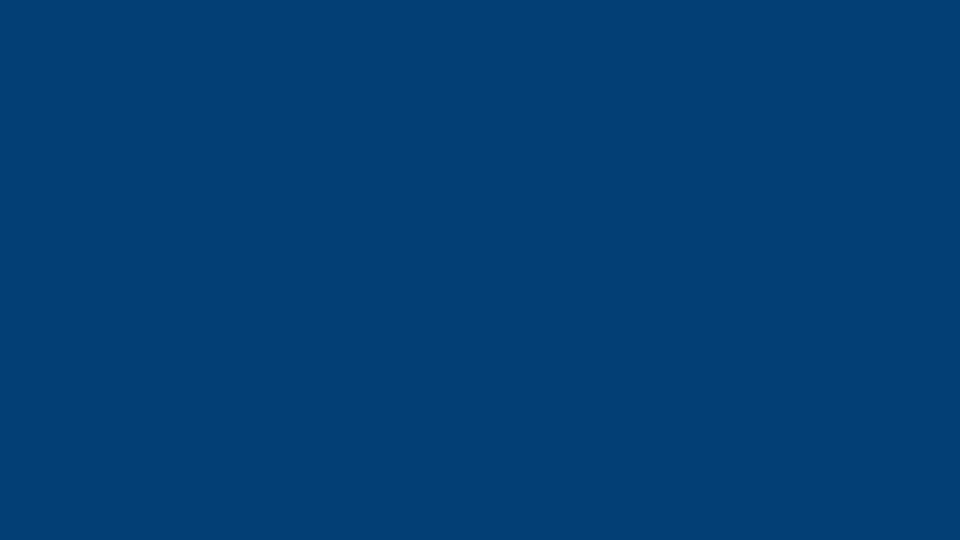
Semantic Texture for Robust Dense Tracking
Semantic Texture for Robust Dense Tracking
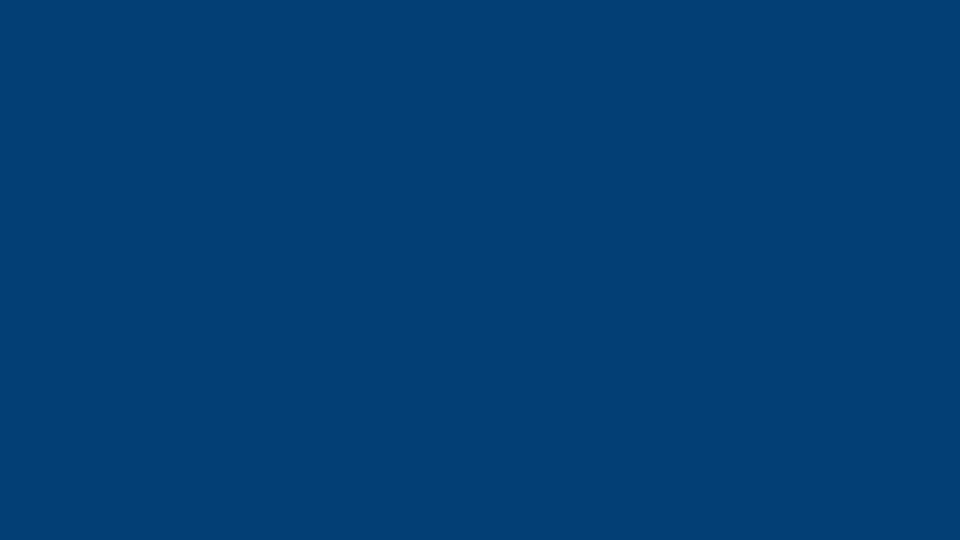
SemanticFusion
SemanticFusion: Dense 3D Semantic Mapping with Convolutional Neural Networks
2016 Widget
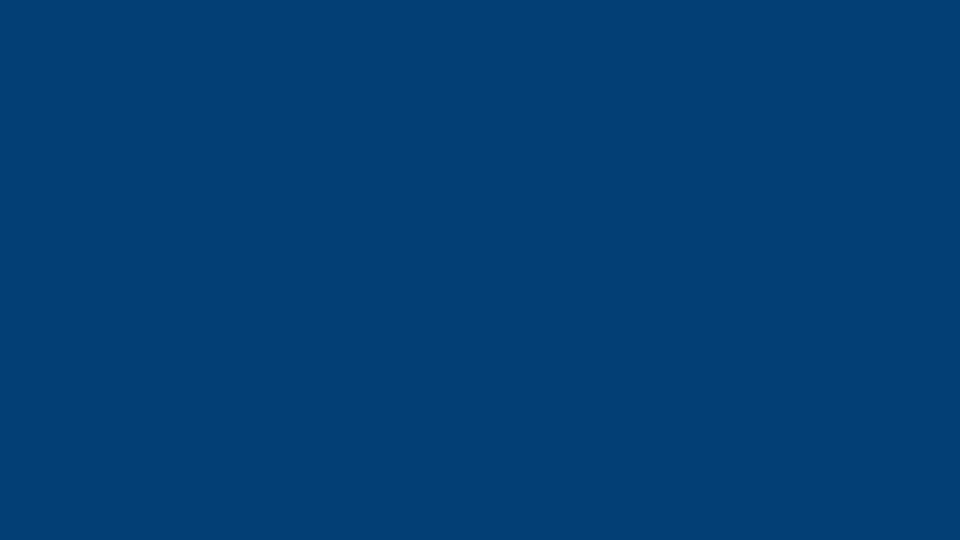
Deep Learning a Grasp Function
Deep Learning a Grasp Function for Grasping under Gripper Pose Uncertainty
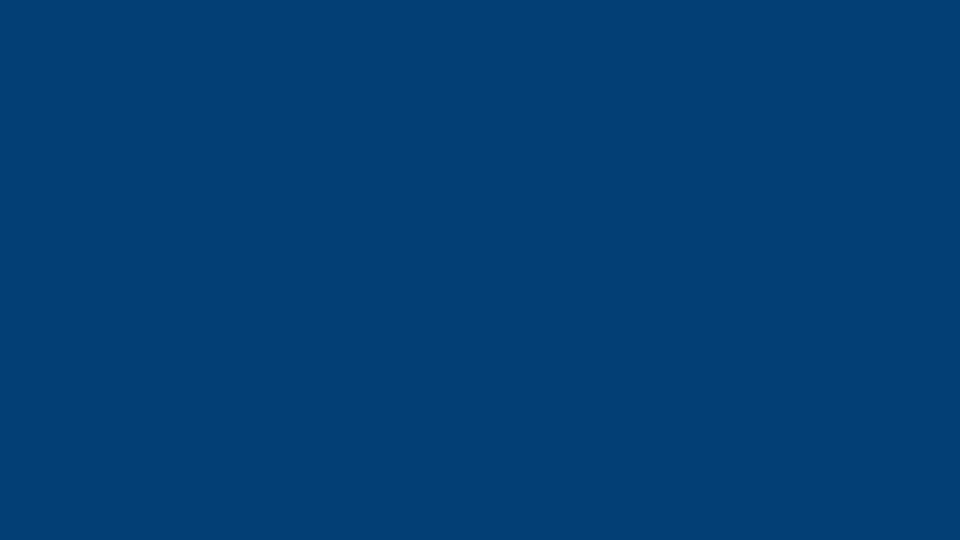
Event Camera
Simultaneous Optical Flow and Intensity Estimation from an Event Camera
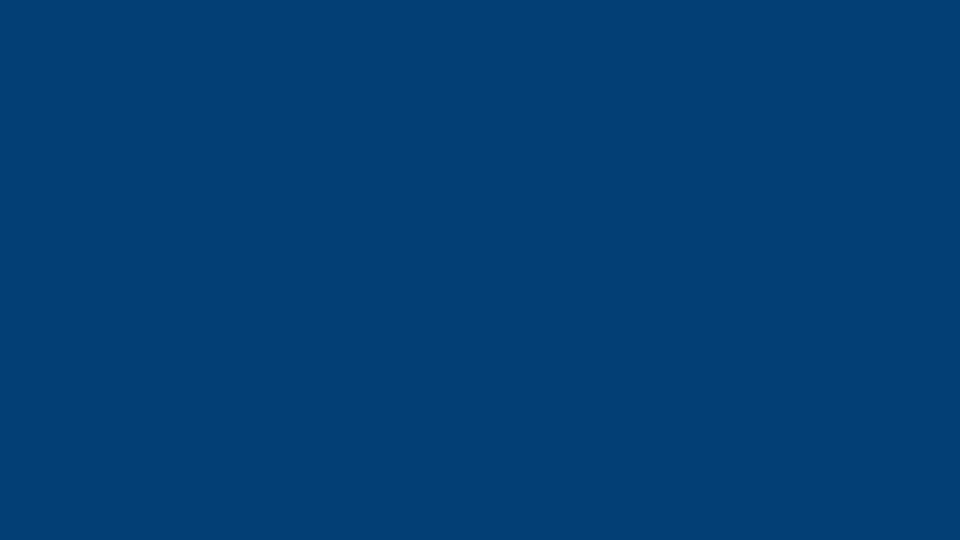
Height Map Fusion
Real Time Height Map Fusion using Differentiable Rendering
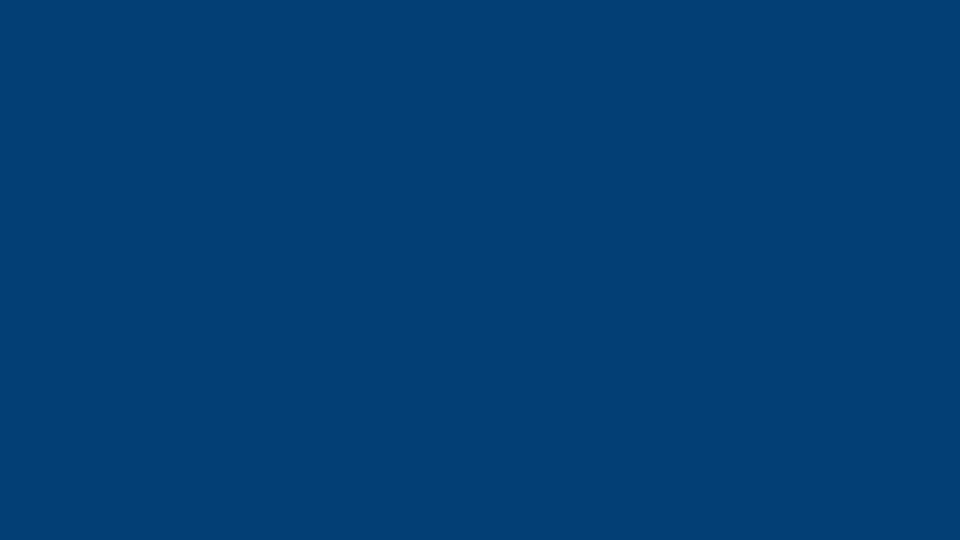
Monocular, Real-Time Surface Reconstruction
Monocular, Real-Time Surface Reconstruction using Dynamic Level of Detail
Elastic Fusion Widget
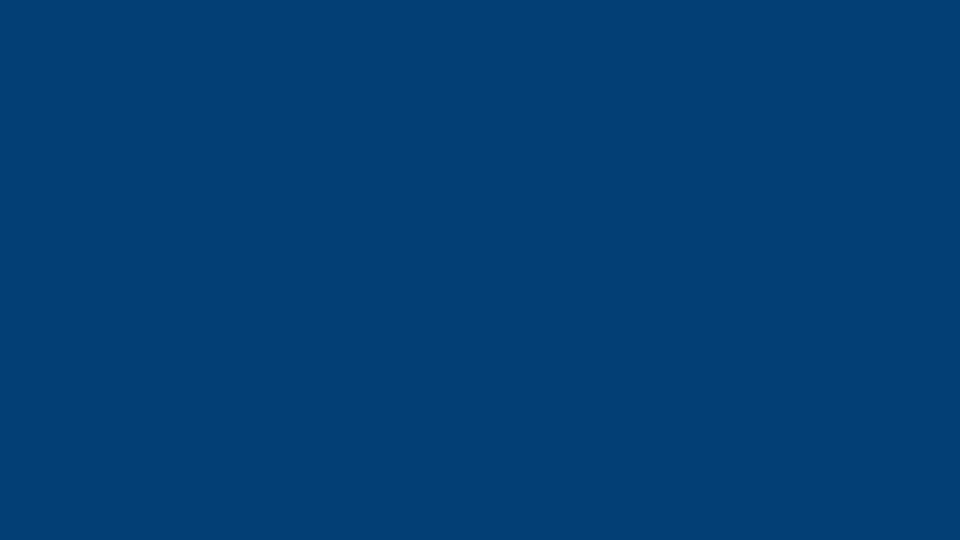
ElasticFusion: Dense SLAM Without A Pose Graph
Demostration of real time ElasticFusion on an office, hotel and copy dataset
The video above demonstrates the Elastic Fusion system, a novel approach to real-time dense visual SLAM. Our approach applies local model-to-model surface loop closure optimisations to stay close to the mode of the map distribution, while utilising global loop optimisations to recover from arbitrary drift and maintain global consistency.
T Whelan, S Leutenegger, B Glocker, R F. Salas-Moreno, AJ Davison, ElasticFusion: Dense SLAM Without A Pose Graph. Robotics: Science and Systems (RSS), Rome, Italy, July 2015
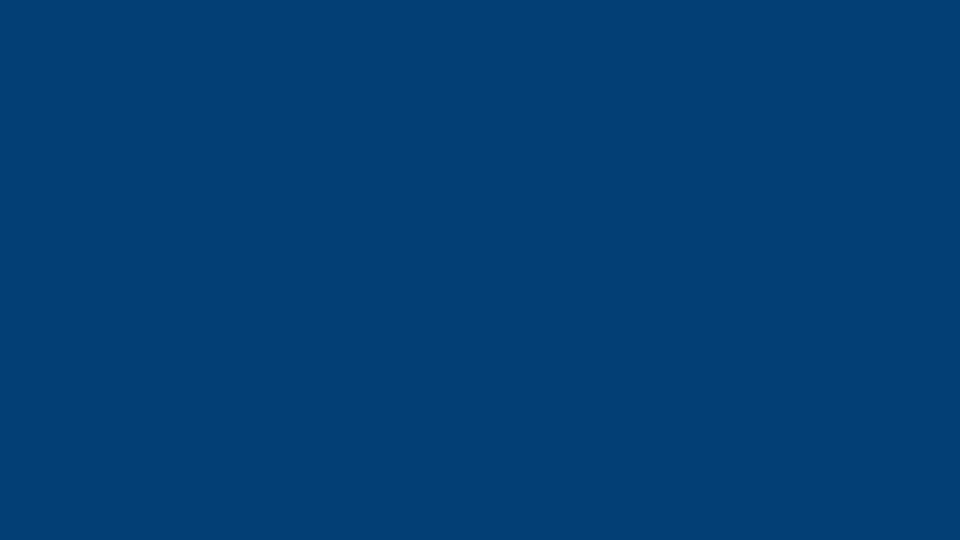
ElasticFusion: Dense SLAM Without A Pose Graph (extras)
ElasticFusion on seating area, garden, The Burghers of Calais, stairs, MIT-76-417b, loopback dataset
The video above demonstrates the Elastic Fusion system, a novel approach to real-time dense visual SLAM. Our approach applies local model-to-model surface loop closure optimisations to stay close to the mode of the map distribution, while utilising global loop optimisations to recover from arbitrary drift and maintain global consistency.
T Whelan, S Leutenegger, B Glocker, R F. Salas-Moreno, AJ Davison, ElasticFusion: Dense SLAM Without A Pose Graph. Robotics: Science and Systems (RSS), Rome, Italy, July 2015