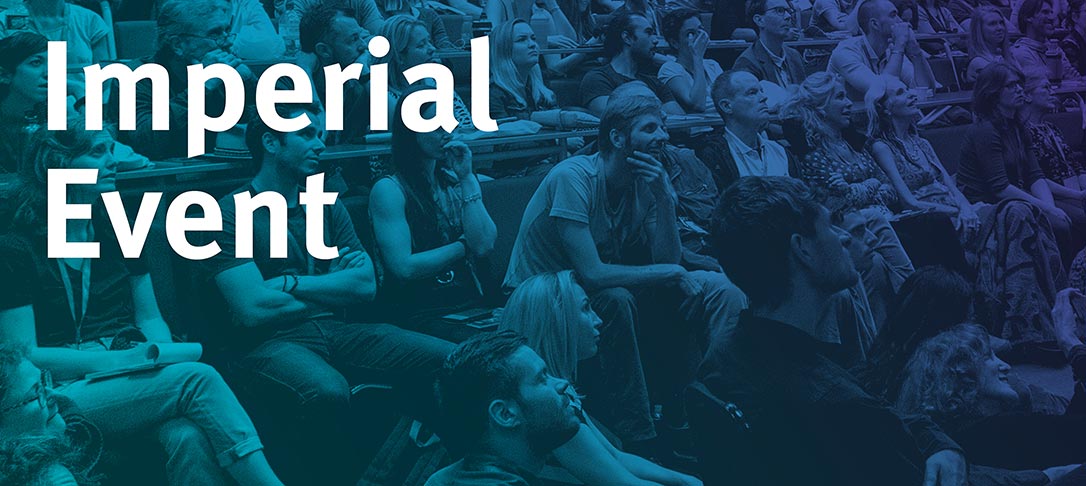
Title
—–
Mars Entry, Descent, and Landing guidance under dynamic uncertainty
The seminar is free to attend but registration is required – please email the organizers to receive an invitation
Abstract
——–
Future Mars exploration missions require improvements of one order of magnitude in landed mass and of two orders of magnitude in landing accuracy with respect to current (Mars 2020) capabilities. These stringent requirements pose significant challenges to current Entry, Descent, and Landing (EDL) guidance algorithms. In addition, the behaviour of the dynamical system is highly uncertain due to the partial knowledge of the Martian atmosphere and of its interaction with the entry vehicle.
In this talk, I will give an overview of some recent advances in EDL guidance under dynamic uncertainty.
First, I will present a novel approach to reconstruct and predict Martian atmospheric density and wind using a Long Short-Term Memory Network (LSTM), a particular class of recurrent neural networks. The LSTM is trained on simulated inertial measurements from a large number of entry trajectories, in which the controls are computed by the Fully Numerical Predictor-corrector Guidance (FNPEG) algorithm.
I show that the network is able to reconstruct atmospheric quantities with very high accuracy, and I am currently working on a generalization of the method to improve the accuracy of the controls obtained through the FNPEG algorithm. I also expound on a stochastic guidance method applying corrections to the controls computed during powered descent to minimize the expected value of the error at landing.
The method is based on the observation that, in nonlinear systems, the mean of the propagated pdf does not coincide with the propagated mean. Thus, we feed to the baseline guidance algorithm a corrected target designed to minimize the expected value of the navigation pdf at the surface; with the latter propagated through an Unscented Transform. Both approaches are of significant import for improving the accuracy and robustness of state-of-the-art EDL algorithms.
Bio
—
Davide Amato is a lecturer in Spacecraft Engineering in the Department of Aeronautics at Imperial College. Prior to joining Imperial, he was a postdoc at the University of Colorado at Boulder, and at the University of Arizona. He obtained his PhD in Aerospace Engineering from the Technical University of Madrid (Spain), and both his Masters and Bachelors in Aerospace Engineering from the Federico University of Naples (Italy). His research area is astrodynamics, with his main interests being space situational awareness, machine learning applied to astrodynamics, and entry, descent, and landing.