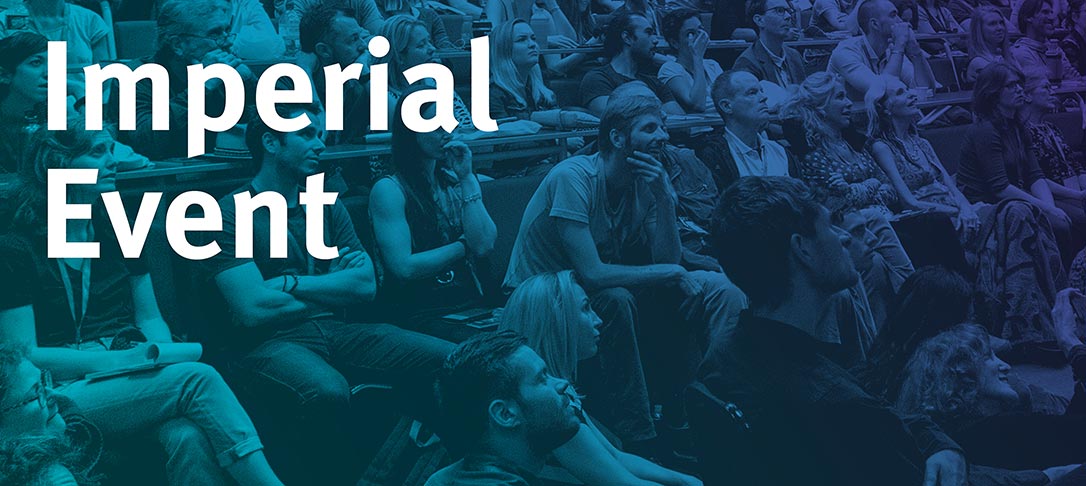
Title: Locally stationary processes in continuous-time
Abstract: Discrete-time locally stationary stochastic processes constitute a class of approaches to model non-stationary time series and find application throughout various disciplines. Despite their success, these approaches have mostly been carried out for models defined on Z, i.e. in a discrete-time framework. Surprisingly, there is so far no general theory for locally stationary models defined on R, i.e. in a continuous-time framework, available. In this talk, we tackle this issue and introduce a theory on stationary approximations for locally stationary continuous-time processes. Based on the stationary approximation, we use a notion of weak dependence to establish laws of large numbers and central limit type results under different observation schemes. Hereditary properties for a large class of finite and infinite memory transformations show the flexibility of the developed theory. In particular, we outline possible applications of our theory to model non-stationary extremes. In addition, we provide inference methodologies suitable for our non-stationary setting by establishing asymptotic properties of M-estimators. As examples we consider different time-varying continuous-time models including time-varying Ornstein-Uhlenbeck processes, for which we introduce a localized Least-Squares estimator. A simulation study shows the applicability of the estimation procedure.