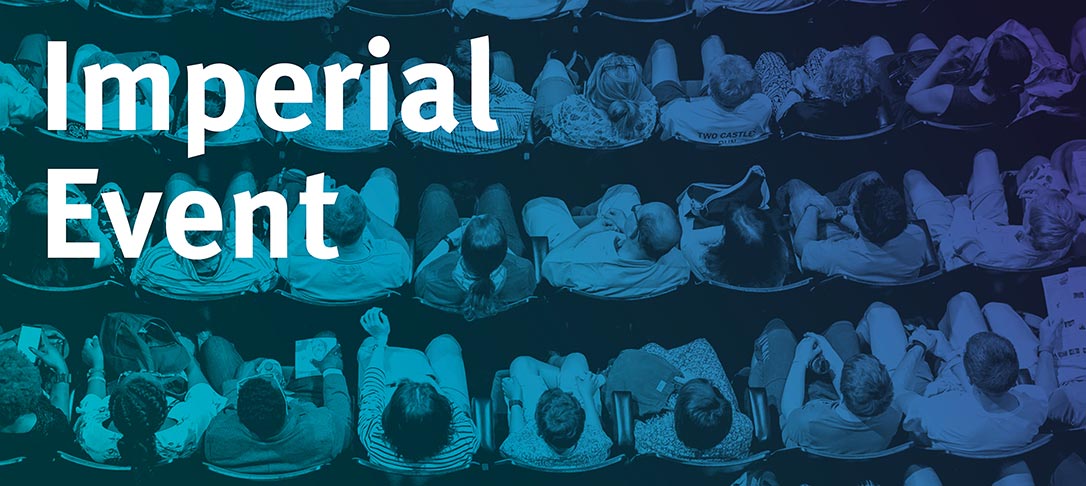
Title: New methods for Bayesian design of experiments with application to calibration of nonlinear models.
Abstract: The quality of statistical inference for the parameters of a nonlinear model depends strongly on the settings of the controllable variables that are applied in the experiment. The collection of such settings is referred to as the design of the experiment. In this talk we discuss how to construct good designs in a Bayesian framework, using expected Shannon information gain as a utility function measuring the quality of the design and the resulting inference. Some key questions that we address include the choice of numerical method for estimating the expected utility and the algorithms for design optimization. In particular we explore the performance of Laplace Importance Sampling (LIS) and a new somewhat cheaper counterpart, Approximate Laplace Importance Sampling (ALIS), showing that these give a good tradeoff between mean squared error and computational complexity. The methods are combined with approximate co-ordinate exchange (ACE), and applied to the case of design for the calibration of a potentially computationally expensive nonlinear model where Gaussian process emulation is required to facilitate timely inference.