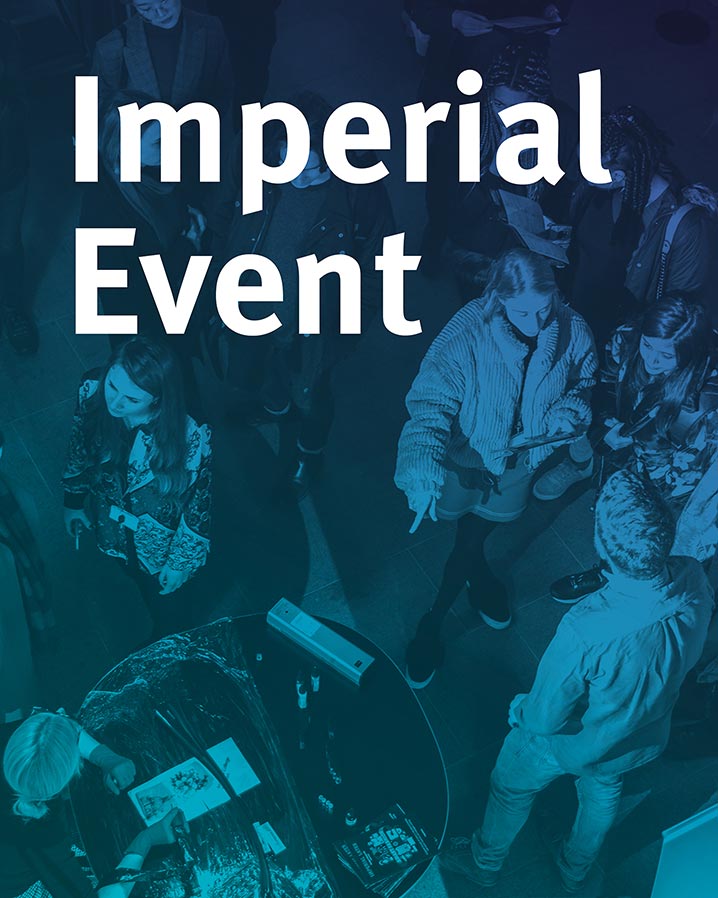
Title: Inferring genotype, phenotype, and clinical relationships with biclustering and statistics
Abstract: The advent of sequencing and high-throughput biotechnologies have transformed our understanding of biology and medicine and have resulted in major advances in diagnosis and treatment of human diseases. The field is moving into an era characterized by vast amounts of unbiased data, including genotyping and inference of the phenotypes from single specimens or individual cells. This in turn demands rigorous computational methods that can extract biologically significant relationships. In particular to the study of cancers, it is imperative to determine tumor mutational landscapes that correspond to transcriptional and epigenetic heterogeneity and quantify clonal remodeling during disease development and under treatment. In this talk, I will present a graph-based biclustering method, which unlike traditional, exclusive and exhaustive clustering, identifies groups of co-regulated genes with respect to a subset of samples and vice versa. The proximity measure embedded in this algorithm allows simultaneous analysis of RNA expression and other measurements such as chromatin accessibility and DNA mutation and methylation, linking the phenotypic profiles to epigenetic and genetic changes. I will also discuss recent evidence that suggest that the solutions to the genotype-to-phenotype problem may reside in the non-coding genome, particularly in regions involved in transcriptional regulation. Based on the results from a set of sequencing and statistical experiments, I will describe the pattern and mechanism of a pervasive hypermutation in super-enhancers as a new layer of genetic alterations that dysregulate gene expression in lymphomas.