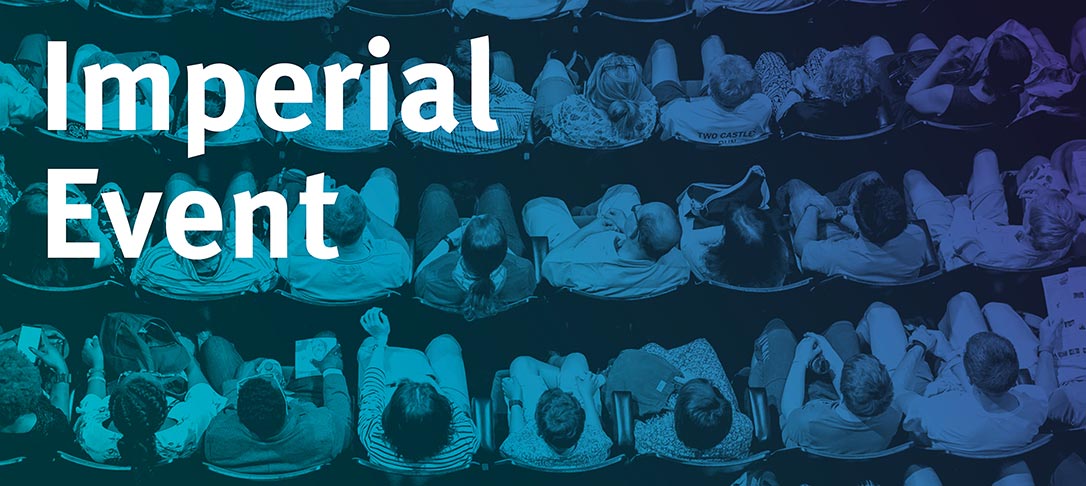
Title:
Learning High-Dimensional McKean-Vlasov Forward-Backward Stochastic Differential Equations with General Distribution Dependence
Abstract:
One of the core problems in mean-field control and mean-field games is to solve the corresponding McKean-Vlasov forward-backward stochastic differential equations (MV-FBSDEs). Most existing methods are tailored to special cases in which the mean-field interaction only depends on expectation or other moments and thus inadequate to solve problems when the mean-field interaction has full distribution dependence.
In this talk, I will introduce a deep learning method for solving MV-FBSDEs with a general form of mean-field interactions. Specifically, built on the fictitious play, we recast the problem into repeatedly solving standard FBSDEs with explicit coefficient functions. These coefficient functions are used to approximate the MV-FBSDEs’ model coefficients with full distribution dependence, and are updated by solving another supervising learning problem using training data simulated from the last iteration’s FBSDE solutions. Deep neural networks are employed to solve standard BSDEs and approximate coefficient functions in order to handle high-dimensional problems. We prove that the step of approximating the coefficient functions does not suffer from the curse of dimensionality. Some numerical examples are presented, including a mean-field game example of the well-known Cucker-Smale model whose cost depends on the full distribution of the forward process.
Biography:
Jiequn Han is a Research Fellow in the Center for Computational Mathematics, Flatiron Institute, Simons Foundation. Previously, he worked as an Instructor in the Department of Mathematics at Princeton University. His research draws inspiration from various disciplines of science and is devoted to solving high-dimensional problems arising from scientific computing. His current research interests mainly focus on solving high-dimensional controls/games and machine learning based-multiscale modeling. He holds a Ph.D. in Applied Mathematics from Princeton University, a B.S. in Computational Mathematics and a B.A. in Economics from Peking University.
Zoom Meeting Details
- Link: https://imperial-ac-uk.zoom.us/j/96333146654?pwd=QnV6blhKSTI5c3lDSExBbXdFSGRwZz09
- Meeting ID: 963 3314 6654
- Passcode: B.p7VZ