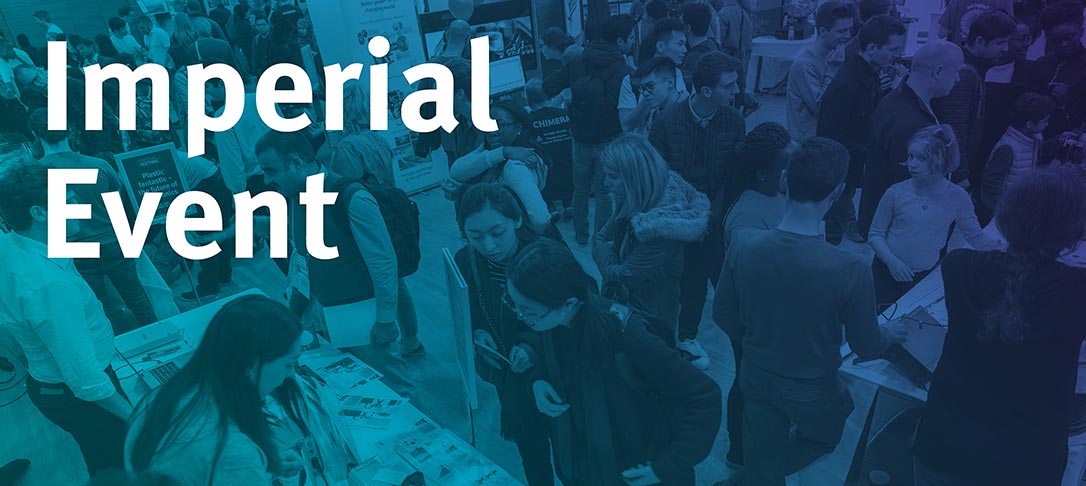
Title: Optimal design of the Barker proposal and other locally-balanced Metropolis- Hastings algorithms
Abstract: We will study the class of first-order locally-balanced Metropolis-Hastings algorithms. Popular choices within the class are the Metropolis-adjusted Langevin algorithm (MALA) andthe recently introduced robust alternative, the Barker proposal. To choose a specific algorithm within the class the user must select a balancing function g : R → R satisfying g(t) = tg(1/t), and a noise distribution for the proposal increment. For targets of product form we establish a universal limiting optimal acceptance rate of 57% and scaling of n^{−1/3} as the dimension n tends to infinity valid for all members of the class. In particular we obtain an explicit expression for the asymptotic efficiency of an arbitrary algorithm in the class, as measured by expected squared jumping distance. Optimising this expression under various constraints can identify an optimal choice of noise distribution for the Barker proposal, optimal choice of balancing function under a Gaussian noise distribution, and optimal choice of first-order locally-balanced algorithm among the entire class, which turns out to depend on the specific target distribution. Numerical simulations confirm our theoretical findings and in particular show that a bi-modal choice of noise distribution in the Barker proposal gives rise to a practical algorithm that is consistently at least as efficient as MALA and as robust as the original version of the Barker proposal with Gaussian noise. This is joint work with Sam Livingstone and Giacomo Zanella.