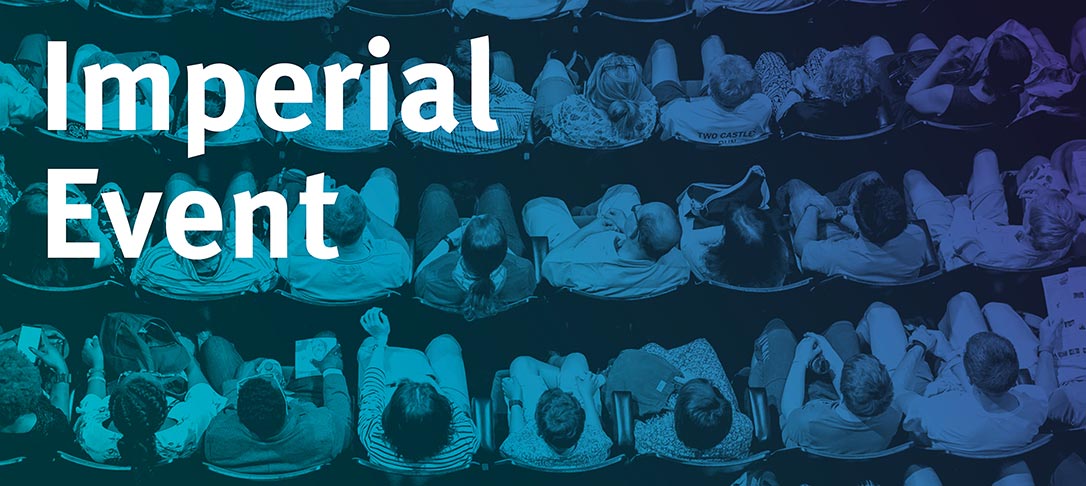
Title:
Convergence Analysis of Forward Propagation for Online Optimization of SDEs
Abstract:
Optimizing over the stationary distribution of stochastic differential equations (SDEs) is computationally challenging. A new forward propagation algorithm is developed and analyzed for the online optimization of SDEs. The algorithm solves an SDE, derived using forward differentiation, which provides a stochastic estimate for the gradient of the objective function. The algorithm continuously updates the SDE model’s parameters and the gradient estimate simultaneously. Convergence is proven for linear and nonlinear dissipative SDEs. We prove bounds on the solutions of a new class of Poisson partial differential equations (PDEs) for the expected time integral of the algorithm’s stochastic fluctuations around the direction of steepest descent. We then re-write the forward propagation algorithm using the PDE solution, which allows us to analyze the parameter evolution around the direction of steepest descent and prove convergence. The numerical performance of the forward propagation algorithm is evaluated for several SDE models.
Biography:
Justin Sirignano is an Associate Professor in the Mathematical Institute at the University of Oxford. Previously, he was an Assistant Professor at the University of Illinois at Urbana-Champaign and a Chapman Fellow at Imperial College London. Justin’s research interests include applied mathematics, stochastic modeling, financial mathematics, and machine learning.