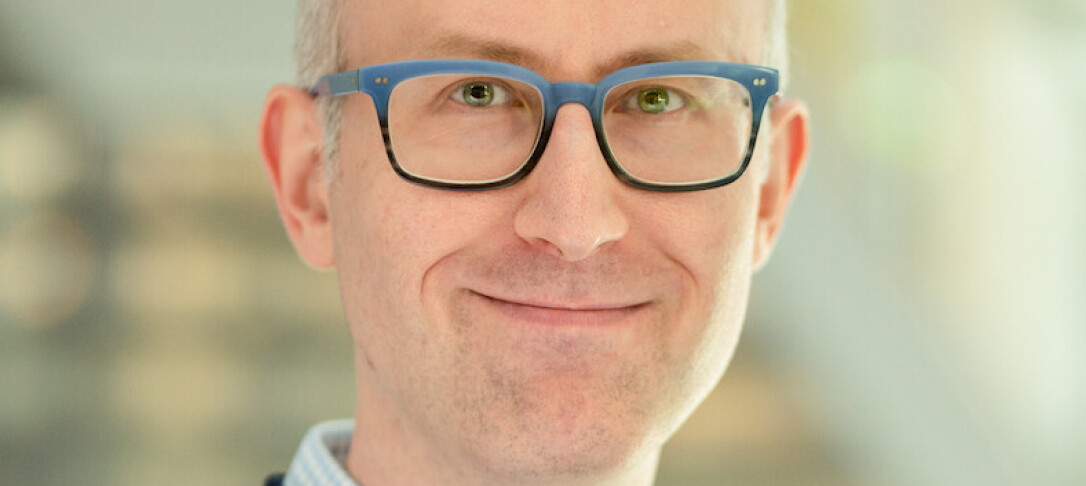
When data-driven trading algorithms are trained, statistical arbitrage opportunities present in the training data may “skew” the behaviour of the trading algorithm. For example, if a utility-maximising hedging algorithm is trained with price paths that possess an upward trend, the algorithm will over-hedge, as it aims to capture alpha in addition to hedging. Such behaviour is undesirable in practice as it may violate the mandate of the trading desk and also lead to poor performance if the trend has evaporated by the time the algorithm is deployed. In this talk I will present a deep learning-based approach to mitigate the problem. Concretely, the approach seeks to learn re-weighting of the training data, akin to constructing an equivalent martingale measure in theory. Joint work with Hans Bühler, Phillip Murray and Ben Wood.