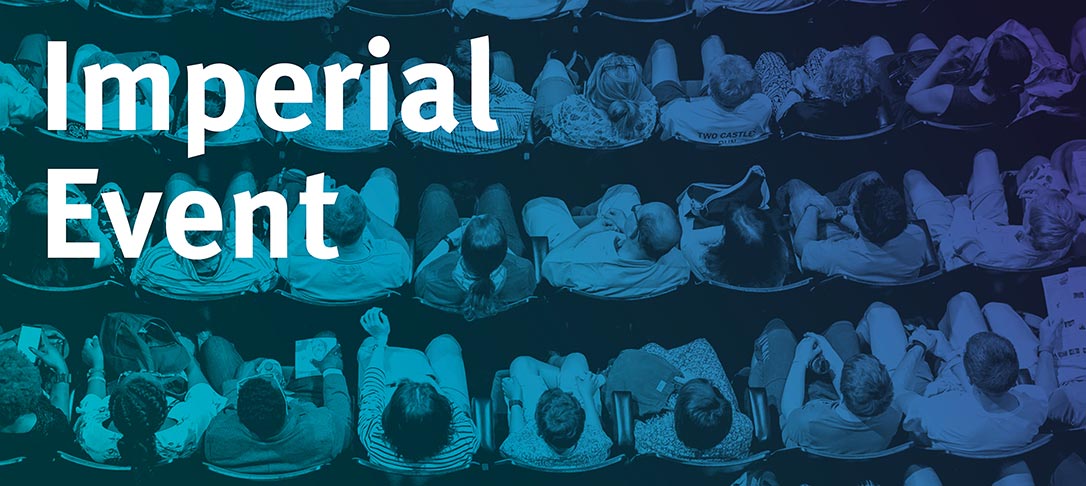
Reduced models of transport necessarily have unknown closure relations which encode higher order physics; for example, the notorious flux limiter. In this work, we present a machine learning approach to finding accurate closure relations utilising differentiable programming. This involves computing derivatives through the solution of a system of partial differential equations. As a case study, we consider the transport of photons and use a literature radiation transport test problem from Su and Olson as a training dataset. We present novel closures for a number of reduced order models including flux-limited diffusion. We evaluate the improvement of the machine-learnt closures over those from the literature. These improvements are then verified by considering a modification to the Su Olson problem; testing the generality of the machine-learnt closures.