Main content blocks
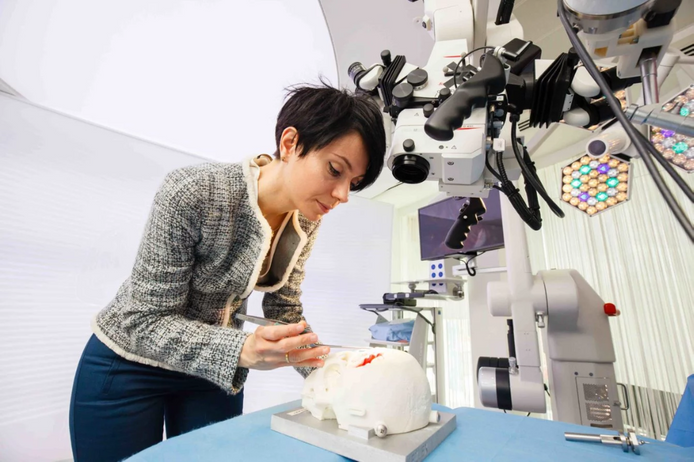
Head of Group
Dr Stamatia Giannarou
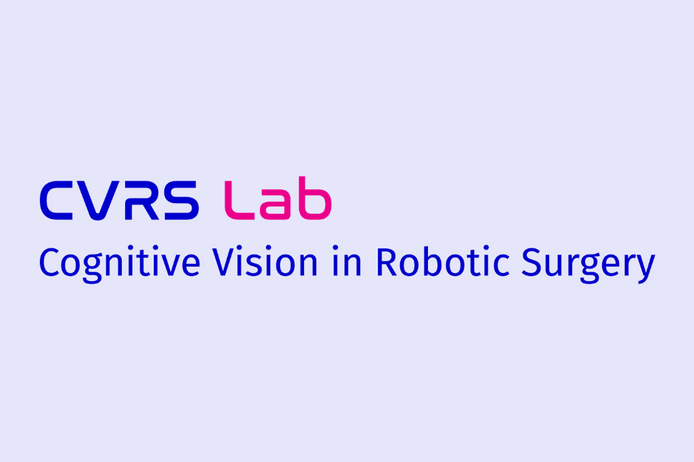
About us
The Cognitive Vision in Robotic Surgery Lab is developing computer vision and AI techniques for intraoperative navigation and real-time tissue characterisation.
Research lab info
What we do
Surgery is undergoing rapid changes driven by recent technological advances and our on-going pursuit towards early intervention and personalised treatment. We are developing computer vision and Artificial Intelligence techniques for intraoperative navigation and real-time tissue characterisation during minimally invasive and robot-assisted operations to improve both the efficacy and safety of surgical procedures. Our work will revolutionize the treatment of cancers and pave the way for autonomous robot-assisted interventions.
Why it is important?
With recent advances in medical imaging, sensing, and robotics, surgical oncology is entering a new era of early intervention, personalised treatment, and faster patient recovery. The main goal is to completely remove cancerous tissue while minimising damage to surrounding areas. However, achieving this can be challenging, often leading to imprecise surgeries, high re-excision rates, and reduced quality of life due to unintended injuries. Therefore, technologies that enhance cancer detection and enable more precise surgeries may improve patient outcomes.
How can it benefit patients?
Our methods aim to ensure patients receive accurate and timely surgical treatment while reducing surgeons' mental workload, overcoming limitations, and minimizing errors. By improving tumor excision, our hybrid diagnostic and therapeutic tools will lower recurrence rates and enhance survival outcomes. More complete tumor removal will also reduce the need for repeat procedures, improving patient quality of life, life expectancy, and benefiting society and the economy.
Meet the team
Dr Stamatia Giannarou
Dr Stamatia Giannarou
Senior Lecturer
Dr Po Wen Lo
Dr Po Wen Lo
Research Associate
Mr Alfie Roddan
Mr Alfie Roddan
Research Postgraduate
Mr Alistair G Weld
Mr Alistair G Weld
Research Postgraduate
Mr Chi Xu
Mr Chi Xu
Research Assistant
Mr Haozheng Xu
Mr Haozheng Xu
Research Assistant
Mr Yihang Zhou
Mr Yihang Zhou
Research Assistant
Results
- Showing results for:
- Reset all filters
Search results
-
Journal articleWeld A, Dixon L, Anichini G, et al., 2024,
Challenges with segmenting intraoperative ultrasound for brain tumours
, Acta Neurochirurgica: the European journal of neurosurgery, Vol: 166, ISSN: 0001-6268Objective - Addressing the challenges that come with identifying and delineating brain tumours in intraoperative ultrasound. Our goal is to both qualitatively and quantitatively assess the interobserver variation, amongst experienced neuro-oncological intraoperative ultrasound users (neurosurgeons and neuroradiologists), in detecting and segmenting brain tumours on ultrasound. We then propose that, due to the inherent challenges of this task, annotation by localisation of the entire tumour mass with a bounding box could serve as an ancillary solution to segmentation for clinical training, encompassing margin uncertainty and the curation of large datasets. Methods - 30 ultrasound images of brain lesions in 30 patients were annotated by 4 annotators - 1 neuroradiologist and 3 neurosurgeons. The annotation variation of the 3 neurosurgeons was first measured, and then the annotations of each neurosurgeon were individually compared to the neuroradiologist's, which served as a reference standard as their segmentations were further refined by cross-reference to the preoperative magnetic resonance imaging (MRI). The following statistical metrics were used: Intersection Over Union (IoU), Sørensen-Dice Similarity Coefficient (DSC) and Hausdorff Distance (HD). These annotations were then converted into bounding boxes for the same evaluation. Results - There was a moderate level of interobserver variance between the neurosurgeons [ I o U : 0.789 , D S C : 0.876 , H D : 103.227 ] and a larger level of variance when compared against the MRI-informed reference standard annotations by the neuroradiologist, mean across annotators [ I o U : 0.723 , D S C : 0.813 , H D : 115.675 ] . After converting the segments to bounding boxes, all metrics improve, most significantly, the interquartile range drops by [ I o U : 37 % , D S C : 41 % , H D : 54 % ] . Conclusion - This study highlights the current challenges with detecting and defining tumour boundaries in neuro-oncologi
-
Journal articleLo FP-W, Qiu J, Wang Z, et al., 2024,
Dietary Assessment with Multimodal ChatGPT: A Systematic Analysis.
, IEEE J Biomed Health Inform, Vol: PPConventional approaches to dietary assessment are primarily grounded in self-reporting methods or structured interviews conducted under the supervision of dietitians. These methods, however, are often subjective, potentially inaccurate, and time-intensive. Although artificial intelligence (AI)-based solutions have been devised to automate the dietary assessment process, prior AI methodologies tackle dietary assessment in a fragmented landscape (e.g., merely recognizing food types or estimating portion size), and encounter challenges in their ability to generalize across a diverse range of food categories, dietary behaviors, and cultural contexts. Recently, the emergence of multimodal foundation models, such as GPT-4V, has exhibited transformative potential across a wide range of tasks (e.g., scene understanding and image captioning) in various research domains. These models have demonstrated remarkable generalist intelligence and accuracy, owing to their large-scale pre-training on broad datasets and substantially scaled model size. In this study, we explore the application of GPT-4V powering multimodal ChatGPT for dietary assessment, along with prompt engineering and passive monitoring techniques. We evaluated the proposed pipeline using a self-collected, semi free-living dietary intake dataset comprising 16 real-life eating episodes, captured through wearable cameras. Our findings reveal that GPT-4V excels in food detection under challenging conditions without any fine-tuning or adaptation using food-specific datasets. By guiding the model with specific language prompts (e.g., African cuisine), it shifts from recognizing common staples like rice and bread to accurately identifying regional dishes like banku and ugali. Another GPT-4V's standout feature is its contextual awareness. GPT-4V can leverage surrounding objects as scale references to deduce the portion sizes of food items, further facilitating the process of dietary assessment.
-
Journal articleYou J, Ajlouni S, Kakaletri I, et al., 2024,
XRelevanceCAM: towards explainable tissue characterization with improved localisation of pathological structures in probe-based confocal laser endomicroscopy
, INTERNATIONAL JOURNAL OF COMPUTER ASSISTED RADIOLOGY AND SURGERY, Vol: 19, Pages: 1061-1073, ISSN: 1861-6410 -
Journal articleXu C, Xu H, Giannarou S, 2024,
Distance Regression Enhanced with Temporal Information Fusion and Adversarial Training for Robot-Assisted Endomicroscopy.
, IEEE Trans Med Imaging, Vol: PPProbe-based confocal laser endomicroscopy (pCLE) has a role in characterising tissue intraoperatively to guide tumour resection during surgery. To capture good quality pCLE data which is important for diagnosis, the probe-tissue contact needs to be maintained within a working range of micrometre scale. This can be achieved through micro-surgical robotic manipulation which requires the automatic estimation of the probe-tissue distance. In this paper, we propose a novel deep regression framework composed of the Deep Regression Generative Adversarial Network (DR-GAN) and a Sequence Attention (SA) module. The aim of DR-GAN is to train the network using an enhanced image-based supervision approach. It extents the standard generator by using a well-defined function for image generation, instead of a learnable decoder. Also, DR-GAN uses a novel learnable neural perceptual loss which combines for the first time spatial and frequency domain features. This effectively suppresses the adverse effects of noise in the pCLE data. To incorporate temporal information, we've designed the SA module which is a cross-attention module, enhanced with Radial Basis Function based encoding (SA-RBF). Furthermore, to train the regression framework, we designed a multi-step training mechanism. During inference, the trained network is used to generate data representations which are fused along time in the SA-RBF module to boost the regression stability. Our proposed network advances SOTA networks by addressing the challenge of excessive noise in the pCLE data and enhancing regression stability. It outperforms SOTA networks applied on the pCLE Regression dataset (PRD) in terms of accuracy, data quality and stability.
-
Journal articleTukra S, Xu H, Xu C, et al., 2024,
Generalizable stereo depth estimation with masked image modelling
, Healthcare Technology Letters, Vol: 11, Pages: 108-116, ISSN: 2053-3713Generalizable and accurate stereo depth estimation is vital for 3D reconstruction, especially in surgery. Supervised learning methods obtain best performance however, limited ground truth data for surgical scenes limits generalizability. Self-supervised methods don't need ground truth, but suffer from scale ambiguity and incorrect disparity prediction due to inconsistency of photometric loss. This work proposes a two-phase training procedure that is generalizable and retains the high performance of supervised methods. It entails: (1) performing self-supervised representation learning of left and right views via masked image modelling (MIM) to learn generalizable semantic stereo features (2) utilizing the MIM pre-trained model to learn robust depth representation via supervised learning for disparity estimation on synthetic data only. To improve stereo representations learnt via MIM, perceptual loss terms are introduced, which improve the model's stereo representations learnt by explicitly encouraging the learning of higher scene-level features. Qualitative and quantitative performance evaluation on surgical and natural scenes shows that the approach achieves sub-millimetre accuracy and lowest errors respectively, setting a new state-of-the-art. Despite not training on surgical nor natural scene data for disparity estimation.
-
Journal articleDyck M, Weld A, Klodmann J, et al., 2024,
Toward Safe and Collaborative Robotic Ultrasound Tissue Scanning in Neurosurgery
, IEEE TRANSACTIONS ON MEDICAL ROBOTICS AND BIONICS, Vol: 6, Pages: 64-67 -
Conference paperRoddan A, Yu Z, Leiloglou M, et al., 2024,
Towards real-time hyperspectral imaging in neurosurgery
, ISSN: 0277-786XThis study aims to integrate real-time hyperspectral (HS) imaging with a surgical microscope to assist neurosurgeons in differentiating between healthy and pathological tissue during procedures. Using the LEICA M525 microscope's optical ports, we register HS data and RGB, in an efforts to improve margin delineation and surgical outcomes. The CUBERT ULTRIS SR5 camera with 51 bands and 15 Hz is employed, and critical calibration steps are outlined for clinical application. Experimental validation is conducted on ex-vivo animal tissue using reflectance spectroscopy. We present the preliminary validation results of the performance comparison between the designed hyperspectral imaging microscope prototype and diffuse reflectance spectroscopy conducted on animal tissue.
-
Book chapterGiannarou S, Xu C, Roddan A, 2024,
Endomicroscopy
, Biophotonics and Biosensing: from Fundamental Research to Clinical Trials through Advances of Signal and Image Processing, Pages: 269-284Endomicroscopy is an enabling technology that can transform tissue characterization, allowing optical biopsies to be taken during diagnostic and interventional procedures, assisting tissue characterization and decision-making. New techniques, such as probe-based confocal laser endomicroscopy (pCLE) have enabled direct visualization of the tissue at a microscopic level and have been approved for clinical use in a range of clinical applications. Recent pilot studies suggest that the technique may have a role in identifying residual cancer tissue and improving resection rates. The aim of this chapter is to present the technological advances in this area, describe the challenges and limitations associated with this imaging modality and present methods which have been developed to facilitate the application of this technique as well as understanding of the collected data.
-
Journal articleCartucho J, Weld A, Tukra S, et al., 2024,
SurgT challenge: Benchmark of soft-tissue trackers for robotic surgery
, MEDICAL IMAGE ANALYSIS, Vol: 91, ISSN: 1361-8415 -
Conference paperRoddan A, Xu C, Ajlouni S, et al., 2023,
Explainable image classification with improved trustworthiness for tissue characterisation
, MICCAI 2023, Publisher: Springer Nature Switzerland, Pages: 575-585, ISSN: 0302-9743The deployment of Machine Learning models intraoperatively for tissue characterisation can assist decision making and guide safe tumour resections. For the surgeon to trust the model, explainability of the generated predictions needs to be provided. For image classification models, pixel attribution (PA) and risk estimation are popular methods to infer explainability. However, the former method lacks trustworthiness while the latter can not provide visual explanation of the model’s attention. In this paper, we propose the first approach which incorporates risk estimation into a PA method for improved and more trustworthy image classification explainability. The proposed method iteratively applies a classification model with a PA method to create a volume of PA maps. We introduce a method to generate an enhanced PA map by estimating the expectation values of the pixel-wise distributions. In addition, the coefficient of variation (CV) is used to estimate pixel-wise risk of this enhanced PA map. Hence, the proposed method not only provides an improved PA map but also produces an estimation of risk on the output PA values. Performance evaluation on probe-based Confocal Laser Endomicroscopy (pCLE) data verifies that our improved explainability method outperforms the state-of-the-art.
This data is extracted from the Web of Science and reproduced under a licence from Thomson Reuters. You may not copy or re-distribute this data in whole or in part without the written consent of the Science business of Thomson Reuters.
Contact Us
General enquiries
hamlyn@imperial.ac.uk
Facility enquiries
hamlyn.facility@imperial.ac.uk
The Hamlyn Centre
Bessemer Building
South Kensington Campus
Imperial College
London, SW7 2AZ
Map location