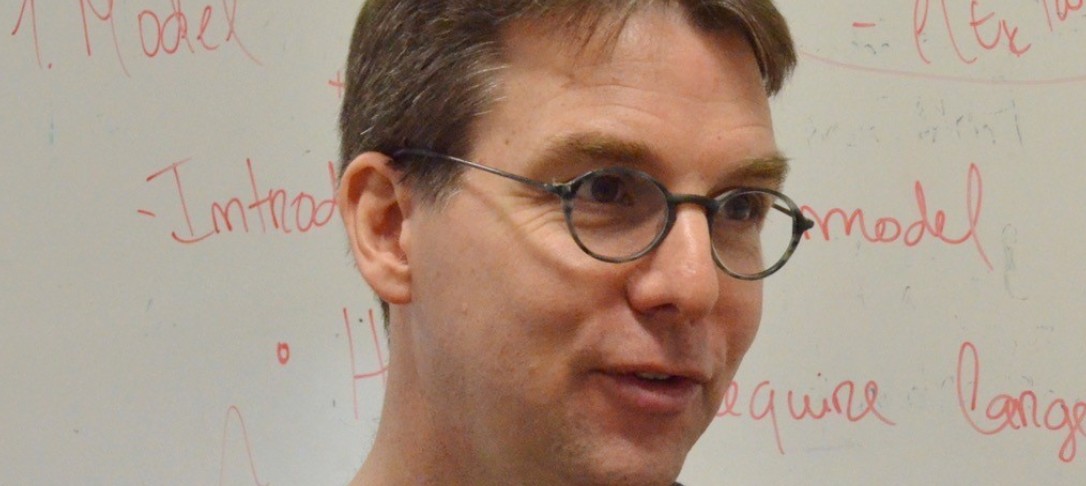
Physics offers countless examples for which theoretical predictions are astonishingly powerful. But it’s hard to imagine a similar precision in complex systems where the number and interdependencies between components simply prohibits a first-principles approach, look no further than the challenge of the billions of neurons and trillions of connections within our own brains. In such settings how do we even identify the important theoretical questions? We describe a systems-scale perspective in which we integrate information theory, dynamical systems and statistical physics to extract understanding directly from measurements. We demonstrate our approach with a reconstructed state space of the behavior of the nematode C. elegans, revealing a chaotic attractor with symmetric Lyapunov spectrum and a novel perspective of motor control. We then outline a maximally predictive coarse-graining in which nonlinear dynamics are subsumed into a linear, ensemble evolution to obtain a simple yet accurate model on multiple scales. With this coarse-graining we identify long timescales and collective states in the Langevin dynamics of a double-well potential, the Lorenz system and in worm behavior. We suggest that such an “inverse’’ approach offers an emergent, quantitative framework in which to seek rather than impose effective organizing principles of complex systems.