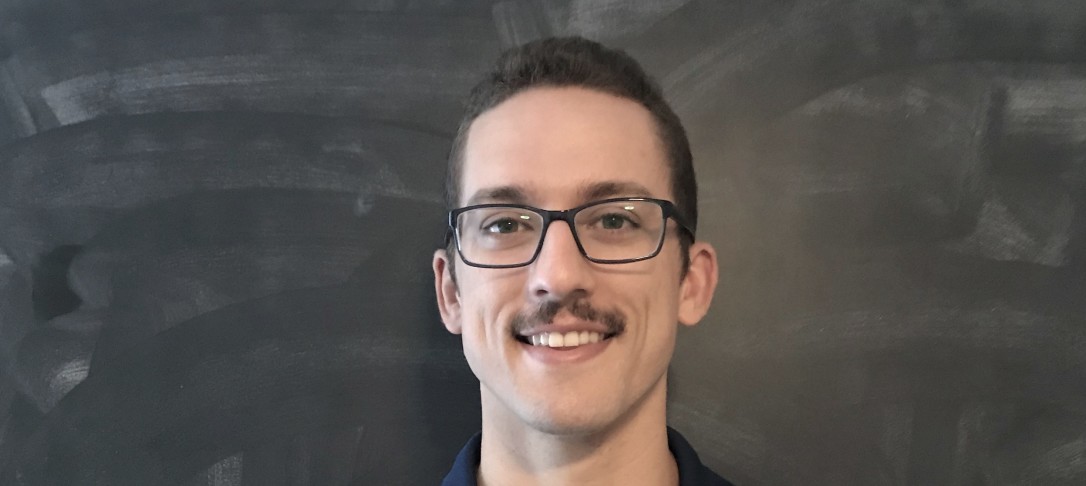
In this talk I am going to discuss a recent work on the convergence problem for mean field control (MFC), which is joint with Pierre Cardaliaguet, Samuel Daudin, and Panagiotis Souganidis. MFC theory is concerned with high-dimensional stochastic control problems, in which an agent controls a large number of particles in order to minimize a “symmetric” cost. In the large population limit, one formally obtains a MFC problem. The convergence problem is the challenge of understanding the precise sense in which the large population models converge to their mean field limit. Qualitative answers to the convergence problem are available under general conditions, but so far quantitative answers are known only when the value function of the MFC problem is smooth. This smoothness is in turn expected to hold only under a convexity assumption on the data. The result I am presenting provides a rate of convergence without convexity. If time permits, I will also discuss a related problem concerning “approximately distributed control problems” outside of the mean field setting, which is based on an ongoing joint work with Daniel Lacker.