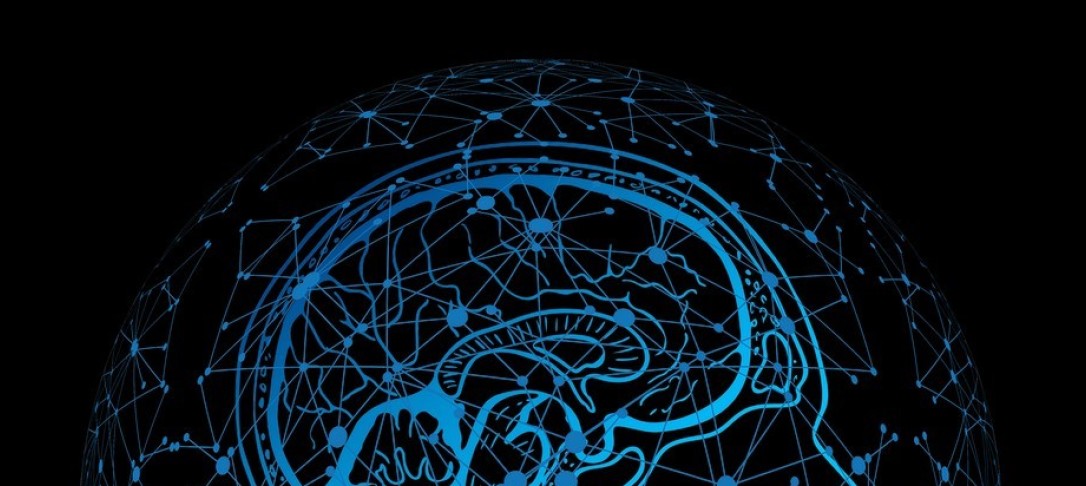
The event intends to bring together leading researchers from academia and financial industry at the intersection of machine learning and finance. The workshop centres around the application and development of novel machine learning methods for hedging, market microstructure and market scenario generation.
Speakers
- Amira Akkari (J.P. Morgan)
- Romuald Elie (Deepmind & Université Gustave Eiffel)
- Blanka Horvath (University of Oxford)
- Antoine Jacquier (Imperial College London)
- Semyon Malamud (École polytechnique fédérale de Lausanne)
- Hao Ni (University College London)
- Johannes Ruf (London School of Economics)
- Josef Teichmann (ETH Zürich)
- Nicholas Westray (Alliance Bernstein and New York University)
The workshop is supported by the Cecilia Tanner Research Funding Scheme.
09:10-09:15 | Opening |
09:15-09:55 | Antoine Jacquier: Random neural networks for rough volatility |
09:55-10:35 | Johannes Ruf: Hedging with linear regressions and neural networks |
10:35-11:00 | Coffee break |
11:00-11:40 | Josef Teichmann: (Learning) Strategies for ergodic robust optimal asymptotic growth under stochastic volatility |
11:40-12:20 | Romuald Elie: Learning equilibria in mean field games |
12:20-13:30 | Lunch break |
13:30-14:10 |
Nicholas Westray: The Informational Content of Cross-Sectional Multi-level Order Flow Imbalance in US Equity Markets |
14:10-14:50 | Amira Akkari: Deep Hedging & Deep Bellman Hedging |
14:50-15:15 | Coffee break |
15:15-15:55 | Hao Ni: PCF-GAN: generating sequential data via the characteristic function of measures on the path space |
15:55-16:35 | Semyon Malamud: Complexity in Factor Pricing Models |
16:35-16:45 | Break |
16:45-17:25 | Blanka Horvath: Pathwise methods and generative models for pricing and trading |
Titles and Abstracts
Antoine Jacquier (Imperial College London)
Title: Random neural networks for rough volatility
Abstract: The classical Feynman-Kac bridge between Markovian SDEs and PDEs has recently been extended in the context of rough (i.e. non-Markovian) stochastic volatility models, giving rise to path-dependent PDEs. The latter, however, lack the numerical analysis foundations their finite-dimensional counterparts have. A naïve discretisation forces one to deal with a high-dimensional PDE, notoriously hard to solve numerically. We focus here on recent developments by Hure-Pham-Warin and Bayer-Qiu-Yao, where the classical backward resolution technique is combined with neural networks to estimate both the solution and its gradient. Not only does this approach successfully diminish the curse of dimensionality, but is also shown to be more effective in both accuracy and computational efficiency than existing Euler-based approaches. Our contribution is to replace their neural networks by reservoir networks (following the steps developed by Gonon), leading to simple least-square minimisation problems instead of large ML-type training considerations. This is a joint work with Zan Zuric (Imperial College London).
Johannes Ruf (London School of Economics)
Title: Hedging with linear regressions and neural networks
Abstract: We study the use of neural networks as nonparametric estimation tools for the hedging of options. To this end, we design a network, named HedgeNet, that directly outputs a hedging strategy given relevant features as input. This network is trained to minimise the hedging error instead of the pricing error. Applied to end-of-day and tick prices of S&P 500 and Euro Stoxx 50 options, the network is able to reduce the mean squared hedging error of the Black-Scholes benchmark significantly. We illustrate, however, that a similar benefit arises by a simple linear regression model that incorporates the leverage effect. Joint work with Weiguan Wang.
Josef Teichmann (ETH Zürich)
Title: (Learning) Strategies for ergodic robust optimal asymptotic growth under stochastic volatility
Abstract: We consider an asymptotic robust growth problem under model uncertainty and in the presence of (non-Markovian) stochastic covariance. We fix two inputs representing the instantaneous covariance for the asset process $X$, which depends on an additional stochastic factor process $Y$, as well as the invariant density of $X$ together with $Y$. The stochastic factor process $Y$ has continuous trajectories but is not even required to be a semimartingale. Our setup allows for drift uncertainty in $X$ and model uncertainty for the local dynamics of $Y$. This work builds upon a recent paper of Kardaras & Robertson, where the authors consider an analogous problem, however, without the additional stochastic factor process. Under suitable, quite weak assumptions we are able to characterize the robust optimal trading strategy and the robust optimal growth rate. The optimal strategy is shown to be functionally generated and, remarkably, does not depend on the factor process $Y$. Our result provides a comprehensive answer to a question proposed by Fernholz in 2002. Mathematically, we use a combination of partial differential equation (PDE), calculus of variations and generalized Dirichlet form techniques. We also point towards ML ways to illustrate the result. Joint work with David Itkin, Benedikt Koch, Martin Larsson.
The theoretical results are accompanied by generative adversarial learning approaches for robust strategies (joint work with Florian Krach and Hanna Wutte).
Romuald Elie (Deepmind & Université Gustave Eiffel)
Title: Learning equilibria in mean field games
Abstract: We will present different approaches and algorithms for learning equilibria in mean field games. In particular, we will consider frameworks where uniqueness of Nash does not hold, and see how one can approximate alternative solution concepts, such as Correlated or Coarse correlated equilibria. Applications such as animal flocking, vehicle routing as well as connections with exploration problems in Reinforcement learning will also be discussed.
Nicholas Westray (Alliance Bernstein and New York University)
Title: The Informational Content of Cross-Sectional Multi-level Order Flow Imbalance in US Equity Markets
Abstract: In this talk we discuss the importance of different types of Order Flow Imbalance (OFI) for contemporaneous return prediction in the US Equity market. We consider multilevel OFI, built from the deeper layers of the order book as well as cross sectional OFI built from the imbalances of other stocks. In the multi-level OFI case we provide a Bayesian formulation to help identify the best number of levels to be used in prediction. In the cross sectional OFI case we provide a highly efficient implementation of the well known Automatic Relevance Determination (ARD) method to help identify the number of cross sectional stocks contributing to the return forecast. We provide practical comments on how to obtain the best model in the cross sectional case, using the Shapley value to assess the contribution of various terms to performance.
Amira Akkari (J.P. Morgan)
Title: Deep Hedging & Deep Bellman Hedging
Abstract: Traditional risk management is based on the Greeks provided by classical valuation models. These models typically have simplified dynamics and assume perfect hedge-ability. As a result, decisions on when and how to hedge are based on traders’ intuition, experience, and view on market dynamics. With Deep Hedging (DH), we go beyond Greek-based hedging and take a new approach to exotics risk management.
DH formulates the hedging problem as a reinforcement learning problem and shifts towards the machine learning paradigm. We will present two formulations of DH. In the first formulation, we solve for the optimal hedging in incomplete markets using a periodic policy search. The model-based policy search approximates the hedging actions (the policy) using deep neural networks. In the second formulation, we solve the more rigorous dynamic programming problem under a Deep Bellman formulation, where the deep hedging problem is expressed into the infinite time horizon through a recursive Bellman representation. This can then be solved numerically by adapting techniques from deep reinforcement learning to give a risk-averse actor critic algorithm. The actor gives the optimal hedge and the critic gives the utility-indifference price of the portfolio.
Hao Ni (University College London)
Title: PCF-GAN: generating sequential data via the characteristic function of measures on the path space
Abstract: Implicit Generative Models (IGMs) have demonstrated a superior capacity in generating high-fidelity samples from the high-dimensional space, especially for static image data. However, these methods struggle to capture the temporal dependence of joint probability distributions induced by time-series data. To tackle this issue, we directly compare the path distributions via the characteristic function of measures on the path space (PCF) from rough path theory, which uniquely characterises the law of stochastic processes. The distance metric via PCF enjoyed several theoretical properties, and it also is linked with the MMD loss on the path space. Furthermore, the PCF loss can be optimised based on the path distribution by learning the optimal unitary representation of PCF, which avoids the need for manual kernel selection and improves test power. We validate the effectiveness of the proposed PCF-GAN on several benchmarking datasets, such as rough volatility data and empirical financial data.
Semyon Malamud (École polytechnique fédérale de Lausanne)
Title: Complexity in Factor Pricing Models
Abstract: We theoretically characterize the behavior of machine learning asset pricing models. We prove that expected out-of-sample model performance – in terms of SDF Sharpe ratio and average pricing errors – is improving in model parameterization (or “complexity”). Our results predict that the best asset pricing models (in terms of expected out-of-sample performance) have an extremely large number of factors (more than the number of training observations or base assets). Our empirical findings verify the theoretically predicted “virtue of complexity” in the cross-section of stock returns and find that the best model combines tens of thousands of factors. We also derive the feasible Hansen-Jagannathan (HJ) bound: The maximal Sharpe ratio achievable by a feasible portfolio strategy. The infeasible HJ bound massively overstates the achievable maximal Sharpe ratio due to a complexity wedge that we characterize. Joint work with Antoine Didisheim, Shikun Ke and Bryan Kelly.
Blanka Horvath (University of Oxford)
Title: Pathwise methods and generative models for pricing and trading
Abstract: The deep hedging framework as well as related deep trading setups have opened new horizons for solving hedging problems under a large variety of models and market conditions. In this setting, generative models and pathwise methods rooted in rough paths have proven to be a powerful tool from several perspectives. At the same time, any model – a traditional stochastic model or a market generator – is at best an approximation of market reality, prone to model-misspecification and estimation errors. In a data-driven setting, especially if sample sizes are limited by constraints, the latter issue becomes even more prevalent, which we demonstrate in examples. This raises the question, how to furnish a modelling setup (for deriving a strategy) with tools that can address the risk of the discrepancy between model and market reality, ideally in a way that is automatically built in the setting. A combination of classical and new tools yields insights into this matter.