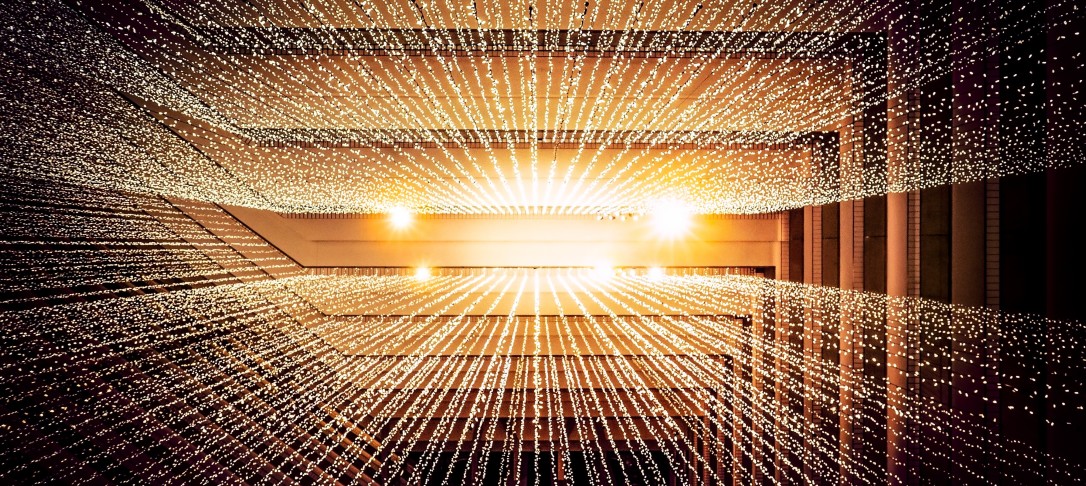
Please note this event has now passed. You can watch a recording of the event via YouTube.
Join the Department of Aeronautics as we launch our exciting new Research Centre in Data-Driven Engineering. The launch event will include inaugural talks from Professor George Karniadakis (Brown University) and Professor Mark Girolami (The Alan Turing Institute, University of Cambridge).
2.00 – 2.45 pm: Professor George Karniadakis – “From Physics-Informed Machine Learning to Physics-Informed Machine Intelligence: Quo Vadimus?”
We will review physics-informed neural networks (NNs) and summarize available extensions for applications in computational science and engineering. We will also introduce new NNs that learn functionals and nonlinear operators from functions and corresponding responses for system identification. The universal approximation theorem of operators is suggestive of the potential of NNs in learning from scattered data any continuous operator or complex system. We first generalise the theorem to deep neural networks, and subsequently we apply it to design a new composite NN with small generalization error, the deep operator network (DeepONet), consisting of a NN for encoding the discrete input function space (branch net) and another NN for encoding the domain of the output functions (trunk net). We demonstrate that DeepONet can learn various explicit operators, e.g., integrals, Laplace transforms and fractional Laplacians, as well as implicit operators that represent deterministic and stochastic differential equations. More generally, DeepOnet can learn multiscale operators spanning across many scales and trained by diverse sources of data simultaneously. Finally, we will present first results on the next generation of these architectures to biologically plausible designs based on spiking neural networks and Hebbian learning that are more efficient and closer to human intelligence.
2.45 – 3.30 pm: Professor Mark Girolami – “Data Driven Finite Element Methodology”
The Finite Element Method (FEM) is a triumph of the mathematical and computational sciences delivering a suite of numerical methods and their software implementations to solve systems of partial differential equations. There is not an area of Science or Engineering that has not been impacted by the availability of rigorous FEM theory, methods, and software. Yet the FEM does not systematically take into account possible observed data from the system being modelled, nor indeed admit that the assumed models themselves may be misspecified. In this work the Hilbert space in which all FEM theory is developed is enriched by including the additional structure of an appropriately defined reference measure from which a probabilistic – data driven – construction of the FEM can proceed. This enables the full suite of statistical tools and analysis to be brought to bear on many engineering problems, which are reliant on the FEM.
About the Data-Driven Engineering Research Centre
The Data-Driven Engineering Research Centre is a consortium of research groups and laboratories to tackle global challenges, such as climate change and sustainable aviation, through accurate modelling, control and optimisation of complex systems with artificial intelligence.