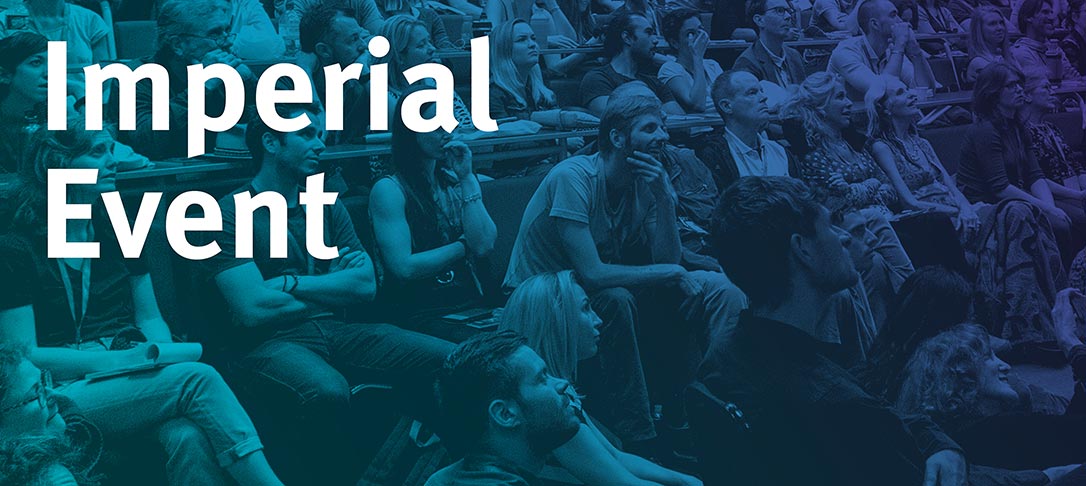
Abstract: This paper presents an analytical characterization of the long run policies learned by algorithms that interact repeatedly. The algorithms observe a state variable and update policies in order to maximize long term discounted payoffs. I show that the long run policies correspond to equilibria that are stable points of a tractable differential equation. As a running example, I consider a repeated Cournot game, for which learning the stage game Nash equilibrium serves as non-collusive benchmark. I give necessary and sufficient conditions for this Nash equilibrium not to be learned. These are requirements on the state variables algorithms use to determine their actions, and on the stage game. When algorithms determine actions based only on the past period’s price, the Nash equilibrium can be learned. However, agents may condition their actions on richer types of information beyond the past period’s price. In that case, I give sufficient conditions such that the policies converge with positive probability to a collusive equilibrium, while never converging to the Nash equilibrium. I show that such richer types of information can enable the learning of the payoff-maximal strongly symmetric equilibrium of the repeated game.