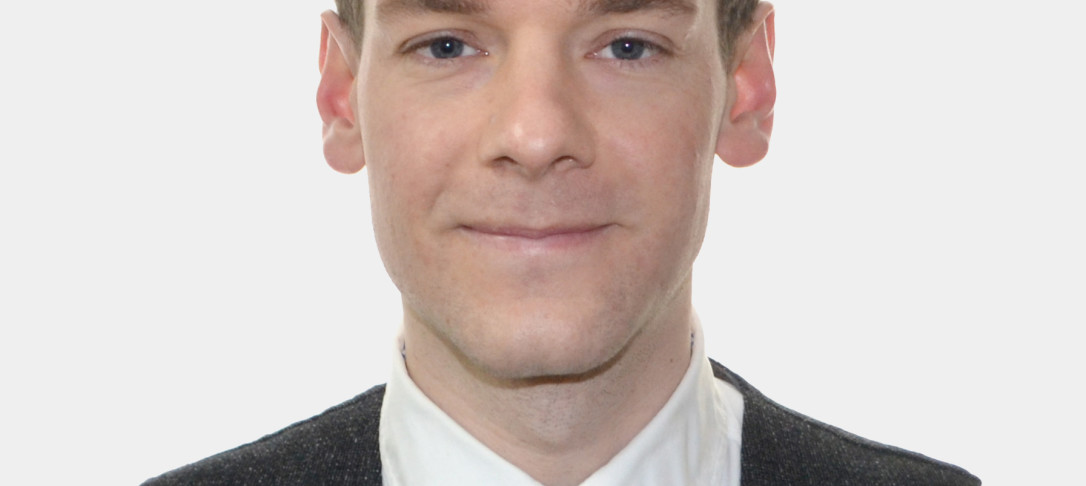
In talk, we will discuss a proximal gradient algorithm for feedback controls of finite-time horizon stochastic control problems. The state dynamics are continuous time nonlinear diffusions with controlled drift and possibly degenerate noise (hence our results also hold for deterministic control problems), and the objectives are nonconvex in the state and nonsmooth in the control. We prove under suitable conditions that the algorithm converges linearly to a stationary point of the control problem. The convergence result justifies the recent reinforcement learning heuristics that adding entropy regularization or a fictitious discount factor to the optimization objective accelerates the convergence of policy gradient methods. The proof exploits careful regularity estimates of backward stochastic differential equations.
This talk is based on joint work with Christoph Reisinger and Yufei Zhang.