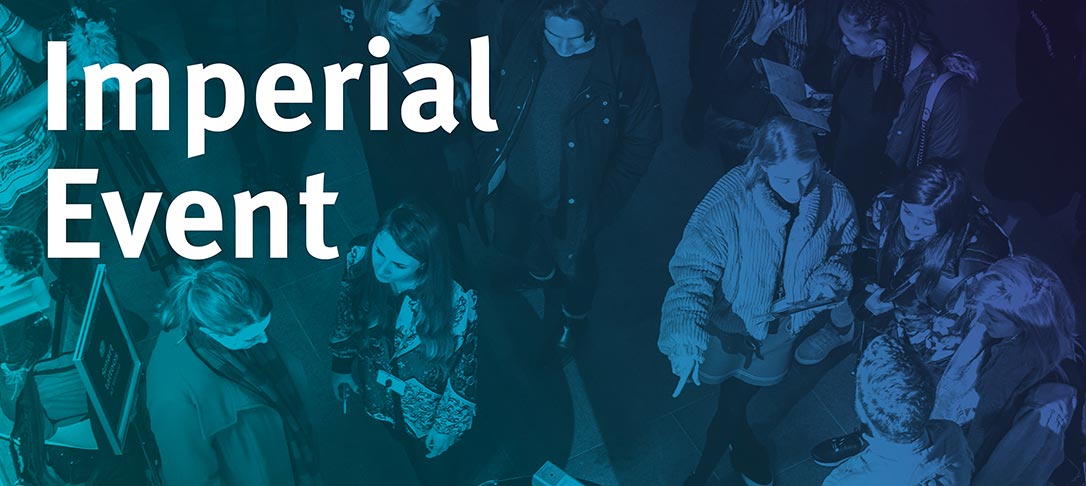
13:30 – 14:30 – Prof. Suhasini Subba Rao (Texas A&M University)
Title: Learning graphical models for nonstationary time series
Abstract: We propose NonStGM, a general nonparametric graphical modeling framework for studying dynamic associations among the components of a nonstationary multivariate time series. It builds on the framework of Gaussian Graphical Models (GGM) and stationary time series, Graphical models (StGM), and complements existing works on parametric graphical models based on change point vector autoregressions (VAR). Analogous to StGM, the proposed framework captures conditional noncorrelations (both intertemporal and contemporaneous) in the form of an undirected graph. In addition, to describe the more nuanced nonstationary relationships among the components of the time series, we introduce the new notion of conditional nonstationarity/stationarity and incorporate it within the graph. This can be used to search for small subnetworks that serve as the “source” of nonstationarity in a large system.
Analogous to classical GMM, we connect conditional noncorrelation and stationarity between and within components of the multivariate time series to zero and Toeplitz embeddings of an infinite-dimensional precision operator. This can be used to build both a micro graphical model (similar to GGM) and a global graphical model (similar to StGM). In the Fourier domain, we show that conditional stationarity and noncorrelation relationships in the precision operator are encoded with a specific sparsity structure of its integral kernel operator. In the last part of the talk we describe how the graphical model can be estimated from the observed time series and some of the sampling properties of the proposed estimation scheme.
15:00 – 16:00 – Dr. Eleni Matechou (University of Kent)
Title: Statistical ecology in the era of big data
Abstract: Ecological data are the result of two, often complex, processes: the underlying ecological process, which is latent, and the error-prone observation process that gives rise to the data. Statistical ecology deals with modelling and separating these two processes so that we can answer questions about the latent ecological process while accounting for the observation process. However, as the dimensions of the data increase, ecological modelling becomes more computationally demanding. In this talk, I will discuss recent examples of “big data” in ecology, some solutions we have explored so far, and the need for more computationally efficient approaches in the future.
Refreshments available between 14:30 – 15:00