Healthcare AI
By Ian Mundell
Artificial intelligence (AI) offers a real opportunity in healthcare, not only to automate some of the problem-solving carried out by doctors and other medical professionals, but also to make quicker and better decisions and apply problem-solving techniques that humans alone could not.
“This will improve the cost of care, and improve outcomes, simply because things will happen earlier, faster, and better,” says Dr Aldo Faisal, who leads Imperial’s UKRI Centre for Doctoral Training in AI for Healthcare. “Rather than replacing people with machines, creating unemployment, we foresee this as a way of dealing with the growing unmet need for clinical care.”
The challenges involved touch on some of the hottest topics in AI research. For example, when you are dealing with people’s health, the explainability of AI – our ability to understand why systems have made certain decisions – becomes much more important. This means dealing with human–machine interaction, trust and the security of AI systems, and questions about autonomy. It also means operating in settings where there is tight regulation.
“AI for healthcare embodies everything that makes AI in general interesting” says Dr Faisal. He divides AI for healthcare into two broad categories. Perceptual AI replicates the ability of healthcare professionals to perceive disease, a skill that goes to the heart of diagnosis and monitoring. And intervention AI addresses decisions about how patients should be treated. Imperial College London is active in both domains.
Pooling clinical experience
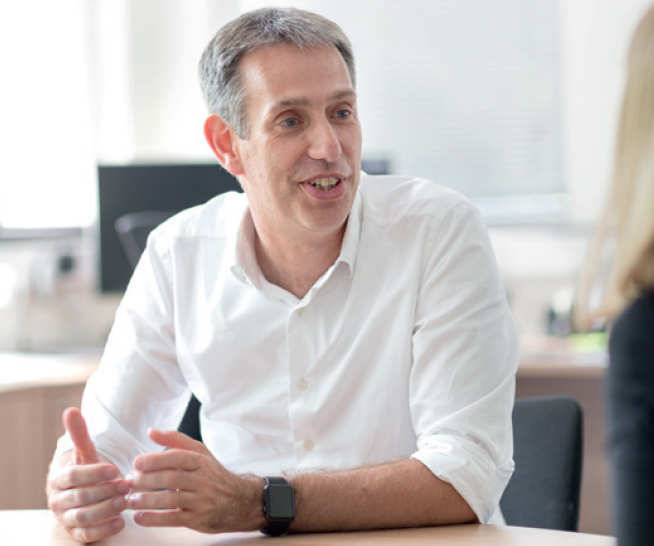
Applying AI to medical imaging is a focus for Professor Daniel Rueckert, head of the Department of Computing and leader of the Biomedical Image Analysis group (BioMedIA).
He describes imaging as a pipeline, beginning with a patient being scanned and ending with clinically useful information. “Our group is applying AI at each stage of the pipeline,” he explains. “We are using AI to acquire images faster and with better quality, to extract information from the images, and to take this information and turn it into a diagnosis or a prediction about the patient.”
For purely visual tasks, the AI is learning to emulate what human experts such as radiologists do when looking for signs of a disease such as cancer. “Humans have a very good perceptual system, and radiologists are trained to spot many different types of diseases. But when it comes to making predictions about the patient, even an experienced doctor may not have seen all types of cancer, or only a few cases of the rarest cancers.”
This is where AI can make a difference. “The learning algorithms can pool the data from hundreds of hospitals, with hundreds of thousands of these rare cases, and support the diagnosis of a clinician who will not have had this experience.”
This approach is also being applied to prenatal ultrasound screening in the iFIND project, a collaboration with clinicians at King’s College London. “One of the challenges of ultrasound is that certain foetal abnormalities are very difficult to spot, even for experienced sonographers,” says Professor Rueckert. There is also a postcode lottery in diagnosis that exists because of uneven training and human resources available across the country.
“AI offers a great way of improving the quality of screening by having the AI operate as a second observer. You still have the sonographer doing the examination, but the AI system can alert the sonographer to things they might need to pay closer attention to.”
Following the heart
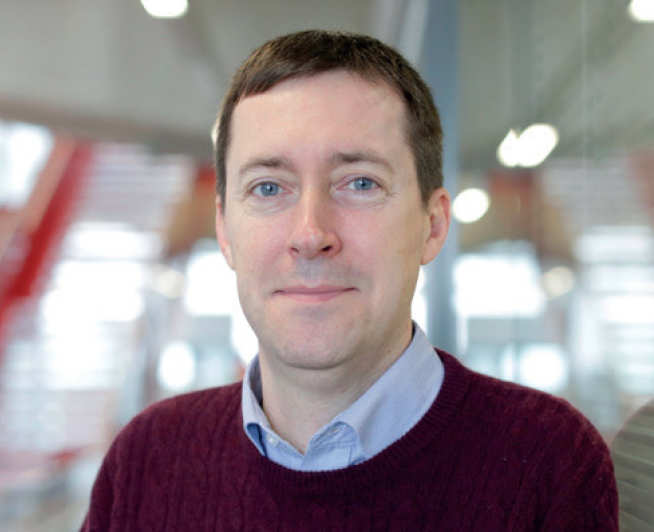
Dr Declan O’Regan, a clinical radiologist at the MRC London Institute of Medical Sciences at Imperial, has been working with Professor Rueckert to apply machine learning to magnetic resonance imaging (MRI) of the heart. The standard method of analysing these images is to draw contours on them by hand and so calculate simple measures such as heart mass and volume. “But there is so much more information in those images, particularly about the early signs of heart failure, that may be difficult for people to appreciate unaided,” he says. “The images may also help us understand some of the complex genetic effects on the structure and function of the heart.”
Machine learning has two roles here. First, it drives computer vision techniques that can track the motion of the heart and build models that capture its structure and function in 3D. Then, together with conventional statistical models, AI can help predict outcomes, for example stratifying patients according to their risk of heart failure and likely response to therapy. This approach is currently the subject of a multicentre trial, which should report by the end of 2020.
Companies are also interested, and Dr O’Regan is leading a project for Bayer Pharmaceuticals that applies this approach. “We are using machine learning to analyse MRI data, then integrating genetic and other health information to identify pathways that could be potential drug targets,” he says. “The idea is to accelerate drug discovery, to find potential new ways of treating serious heart conditions.”
Sharing AI solutions
Meanwhile, Dr Ben Glocker in BioMedIA is working with another company interested in cardiac imaging. The company, HeartFlow, uses routine computed tomography scans to create a digital model of the arteries in the heart and simulate where blockages may be restricting blood flow.
“It’s a very promising non-invasive diagnostic tool, but it’s computationally demanding and this means throughput is limited,” Dr Glocker says. “Some steps in the analytical pipeline are already done with AI-type algorithms, so we are doing research with the company to see if we can make improvements.”
This might mean speeding up processes, increasing accuracy so the need for human verification is reduced, or enabling the system to work on a wider range of image qualities. “These are all research questions where we need to look at new methods and new algorithms.”
The novelty of this collaboration is that, in addition to funding two PhD students, HeartFlow has sent two of its research scientists – one directly from its offices in California – to work within BioMedIA. “They work on problems relevant to HeartFlow, but also discuss and exchange ideas with people here who do all kinds of research on medical imaging,” says Dr Glocker. “So far it is working really well, with benefits in both directions.”
Digital biomarkers
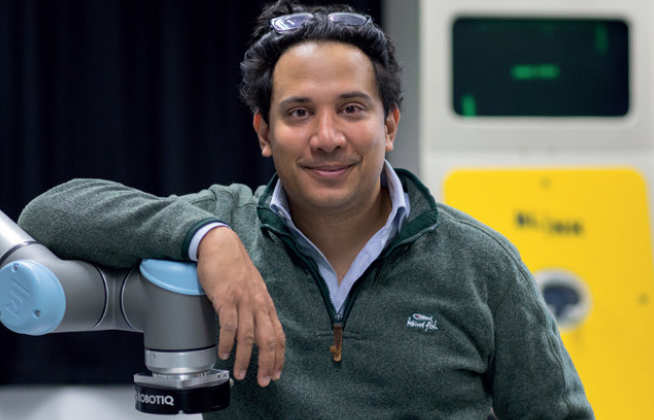
A dramatic case of AI becoming more perceptive than a human clinician can be found in Ethomix, a project to codify and monitor people’s behaviour. “The only way your brain can interact with the world is through movement – you talk, you eat, you walk – and so anything that affects your brain, your nervous system or your physiology is likely to have a signature in your movement behaviour,” says Dr Aldo Faisal, whose research group straddles the Departments of Computing and Bioengineering.
“By measuring behaviour at a very high resolution and applying novel algorithms to that data, we can detect very subtle changes in your brain or nervous system.” He calls these signatures ‘ethomic’ biomarkers, a reference to ethology, the science of animal behaviour.
“We have developed, patented and published a whole range of completely novel ethomic biomarkers that allows us to detect disease progression much faster and much more precisely than was possible before, especially in the area of degenerative diseases.”
These conditions are particularly challenging because their progress can be slow, subtle and hard to measure. This delays not only treatment decisions, but also the development of new therapies, since it can take years for a positive effect in a clinical trial to be confirmed. Using ethomic biomarkers speeds up the process. “We have been able reduce the amount of time it takes to run a clinical trial by 50%,” Dr Faisal says.
Meanwhile by wearing a motion tracker linked to the AI, a patient can be monitored continuously without visiting a clinic. “You simply wear the sensor and live your life.”
Detecting brain tumour changes
Movement is also the key to BrainWear, a system for assessing the progress of brain tumours, which is being developed and trialled by Dr Matthew Williams. He leads the Computational Oncology Group at Imperial, which straddles the Departments of Computing, and Surgery and Cancer.
“The underlying idea is that there is a close link in the brain between location and function,” he explains. “As a brain tumour gets bigger, it is likely to affect function in different ways. Some tumours might affect speech, some might affect walking, some might affect other functions.”
Movement is measured with a wrist accelerometer gathering data in three dimensions, 100 times a second. Unlike a commercial fitness tracker, which reduces motion data to a simple measure such as the number of steps taken, the BrainWear monitor produces a huge amount of raw data for the AI to work with.
“We don’t just want to measure how much you are walking, but how you are walking,” Dr Williams says. “So we apply deep learning to that data to pick out significant features of someone’s gait, to establish what is normal and recognise changes that are down to the disease.”
Meanwhile, factoring in the patient’s treatment should make it possible to rule out changes due to ongoing therapy. “We are now at the stage of collecting data from patients and carers and looking at what that can tell us.” Initial results are expected by the end of 2019. “But the real challenge comes in integrating the different sources of data – fatigue, quality of life and activity – to provide a coherent picture of the patient over time. That is the ultimate goal.”
BrainWear is unusual in bringing together engineering and data science to solve a problem in cancer management. This is an approach championed by the Cancer Research UK Convergence Science Centre, established at Imperial and the Institute of Cancer Research. “We started our work before the Centre came along, and we are a good example of what it hopes to achieve,” says Dr Williams.
From AI to basic biology
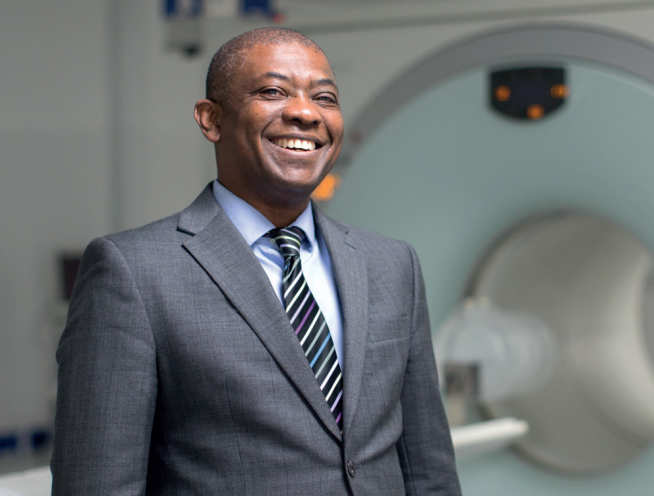
Once an AI system has proved effective at predicting outcomes for a particular disease, a fruitful avenue for research is to ask how its decisions relate to basic biology. This is something that Professor Eric Aboagyein the Department of Surgery and Cancer has been doing for ovarian cancer.
The first step was to design the AI, which looks at scans of ovarian cancer and predicts how the disease will develop. From a panel of 657 scan features, relating to factors such as shape and size, intensity and texture, four were selected that together produce a severity score for each tumour. This Radiomic Prognostic Vector (RPV) proved to be up to four times more accurate in predicting deaths than standard methods.
Then, the researchers set out to explain what the AI was seeing by matching predictions and scans with with genetic and protein data. “When we delved down into the detail we found that a high RPV was strongly correlated with the stromal phenotype, so the micro-environment of the ovarian cancer,” says Professor Aboagye. This suggests that some therapies will be more successful in these cases than others. “Going forward, this means we can begin to see how we use the AI in selecting patients for treatment.”
The next step is to test the method on broader databases, in order to make the case for its use as a routine clinical tool. But it can already be applied to research questions, both in academia and industry. “Pharmaceutical companies have huge imaging data repositories and with the associated clinical data we can go back and mine all of these.”
Consulting an AI clinician
In the area of intervention AI, Dr Aldo Faisal and his colleagues have been developing an AI Clinician. So far this has been applied to diabetes management, neurological disorders, and most recently to intensive care.
Here the AI is told to consider the monitoring data routinely collected in intensive care and to maximise the patient’s chances of survival. Conventionally, an AI would approach this kind of task by trial and error, but this is not something that can be done with a patient. So a sleight of hand is required.
“We fool our algorithm into believing that the doctors’ interventions from the past were its own interventions,” explains Dr Faisal. “Based on these real interactions, which the AI only hypothetically experienced, it learns to become better than the average doctor. Better, in fact, than 99.8% of all clinicians in intensive care.” In practice the AI would not be allowed to take decisions on its own, but only give advice to the doctor or other carers. This makes explainability of paramount importance. “The machine has to say why it is making a recommendation and convince the doctor why it is the right thing to do,” Dr Faisal says.
This also turns out to be a skill that the AI can learn, informed by input from neuroscience and psychology. “We’ve put a lot of thought into how AI deals with clinicians as people – interacting with them and making recommendations. At the same time, we’ve worked on how to make this a regulatable, acceptable way of interacting.” The system is currently being installed at St Mary’s Hospital, London, in preparation for a practical test.
Resolving guideline conflicts
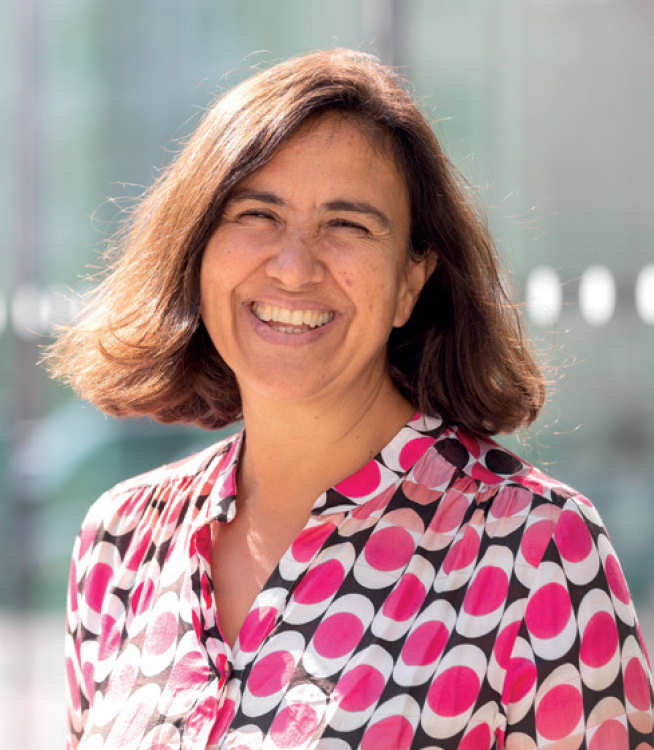
A different kind of AI has been used in the ROAD2H project, which aims to support clinical decision-making for patients with several chronic conditions. For instance, someone with chronic obstructive pulmonary disease (COPD) might also have asthma, hypertension or diabetes.
Doctors are unlikely to be familiar with the clinical guidelines in all areas relevant to their patients, and will not have the time to compare and contrast the advice they give. “The question is, how do we get machines to read the guidelines, then to understand that multiple guidelines apply to patients, and then resolve any conflicts that arise,” says Professor Francesca Toni in the Department of Computing.
Machine learning will not help in this situation, but it can be addressed with another approach to AI. “My expertise is in conflict resolution by means of a form of symbolic AI called argumentation, grounded in computational logic, which is useful where you have different, competing opinions and need to decide how to resolve this conflict.”
As well as absorbing the guidelines, the AI system Professor Toni and her colleagues designed consults electronic health records to verify which aspects of the guidelines apply to each individual patient. It also takes into account a patient’s or doctor’s preferences. For example, a patient may wish to balance treatment with any impacts it may have on their lifestyle, while a doctor may have preferences based on the availability or cost of drugs. Finally, the system explains to the doctor why it is making a particular recommendation. “The goal is to bring the clinician’s attention to points of conflict and suggest possible ways to resolve those conflicts,” Professor Toni says. “But ultimately it is for the clinician to act.”
ROAD2H is a collaboration with King’s College London and partners in China and Serbia. The next step is to trial the system on COPD patients in Serbia, to see how clinicians react.
AI for explanation
A second project within ROAD2H has taken the novel approach of asking an AI system to explain, not itself, but another mathematical system. Specifically, it has been applied to healthcare scheduling, which means managing a nursing roster or matching operations with surgeons and operating theatres.
“There are very sophisticated mathematical optimisation techniques for scheduling, but they are typically very rigid and not understandable,” Professor Toni explains. At best, the system gives you optimal solutions, with no explanation and no opportunity to ask for alternatives.
Working together with her colleagues, Dr Kristijonas Cyras, Dr Dimitrios Letsios and Dr Ruth Misener, an AI solution was found. “We looked at how we could use argumentation to explain the output of optimisation techniques to clinicians, nurses or hospital administrators, and allow for their input if things need to be changed.” The resulting interactive scheduling system is currently being evaluated in a hospital setting.
As well as addressing a healthcare need, this proved a novel application of artificial intelligence. “It’s AI for explanation rather than explainable AI.”
Breaking down barriers for healthcare AI
One of the important challenges in developing AI for healthcare is eliminating divisions between the disciplines involved. One way Imperial is doing this is with the UKRI Centre for Doctoral Training in Artificial Intelligence for Healthcare, which opened in October 2019. Each PhD project in the Centre has two supervisors, one with a background in AI, the other with a background in healthcare. The students come from science and engineering, or medicine, and will do much of their training together. At the same time, there are courses that demystify AI for the clinicians, and explain the clinic to engineers and scientists.
“In this way the engineers and scientists understand what the patient journey looks like, they meet patients and patient organisations, so that from day one students really understand what it means to deliver care,” says Dr Faisal.
Meanwhile a close understanding of medical regulation will help move PhD projects with commercial potential closer to market. “We also have a number of incubators on board that provide opportunities for students to launch a start-up during their studies.” Above all, there must be a connection across the disciplines. “The most important thing we can instil in these students is how important mutual respect is for understanding other people’s disciplines and concerns.”
The range of disciplines involved in healthcare AI is clear from Professor Aboagye’s initiative on ovarian cancer, which included oncologists, radiologists, geneticists, bioinformaticians, pharmacologists and computer scientists. All were vital to debate the questions thrown up by the research. “Imperial has all these people,” he says. “If you have the right questions, then you can bring these diverse researchers together, in an environment that supports and encourages this kind of research.”
Ian Mundell is a journalist who specialises in research and higher education. He divides his time between London and Brussels.