Cyber, Cyber-Physical and Information Security
For further information on Imperial's work in cyber security, including people, please visit the dedicated cyber security section.
Example projects from across Imperial College London
Example projetcs from across Imperial College London
Projects 2
projects 3
Projects
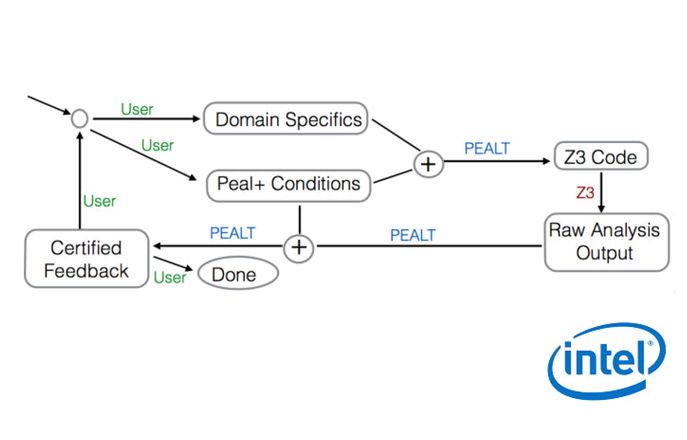
Trust Evidence From Programmers' Intent
This project explores how trust evidence for running software -- but also for more general systems -- can be specified and aggregated in order to support security-relevant decision making. Particular emphasis is being placed on the fact that such evidence may be qualitative as well as quantitative, and that is may cover or interact with a range of modalities such as risk, security, cost, and impact.
PI: Professor Michael Huth
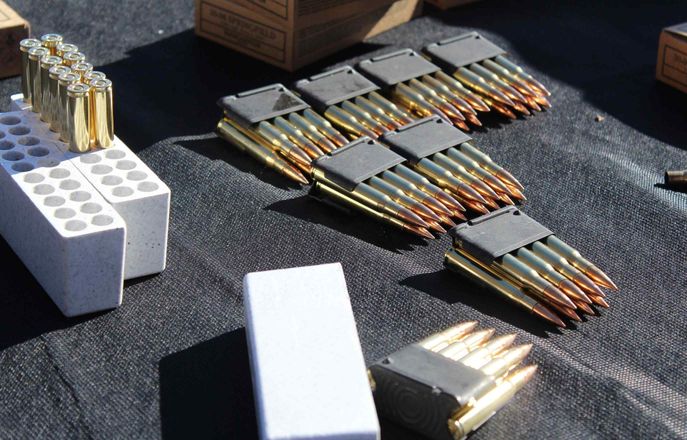
Formal Verification of Treaty Processes
This project, in collaboration with a UK defence company, extends and combines mathematical modelling and verification approaches to make them cope with the inherent lack of available data in the domain of arms control treaty design and implementation. New modelling and analysis methods developed have allowed for a much more sophisticated approach to modelling arms control, and enable a user to optimise treaty-relevant decisions - made during the design or execution phase of a treaty - given various modelling parameters and perhaps even multiple objectives.
PI: Professor Micahel Huth
Projects
Projects
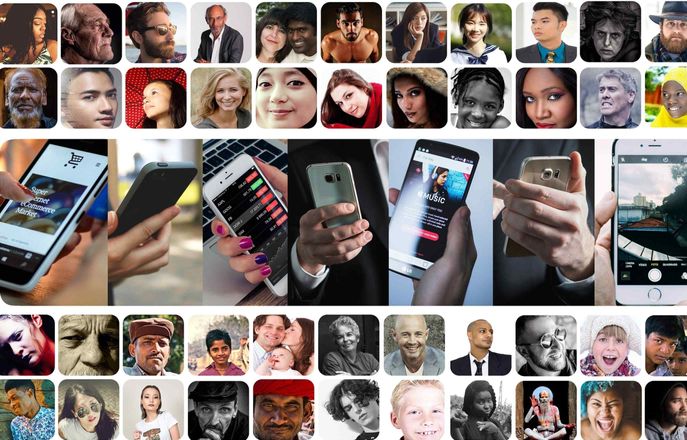
Privacy Dynamics: Learning from the Wisdom of Groups
In recent years, social psychologists have made a core distinction between personal identity and social identity, the formation of which are both dynamic. Understanding the identity process is key to assessing the impact that privacy and security policies have on people’s behaviours. This project studies privacy management by investigating how individuals learn and benefit from their membership of social or functional groups, and how such learning can be automated and incorporated into modern mobile and ubiquitous technologies that increasingly pervade society.
PI: Dr A Russo
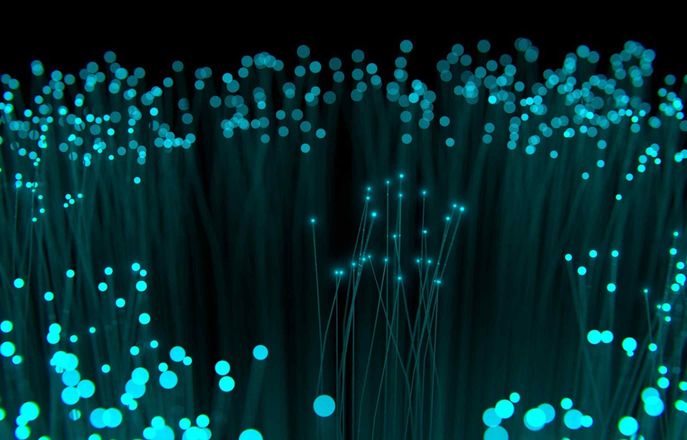
Joint modelling of edge evolution in dynamic graphs
The aim of this project is to develop a general and scalable framework for monitoring and unsupervised anomaly detection of large dynamic graphs. Understanding normal behaviour of communication patterns between pairs of individuals or IP addresses is complex, due to the inherent seasonality and burstiness in human behaviour. The challenge of anomaly detection is to find departures beyond such normal behaviour on the vast collection of edges. It is within this context of dynamic graphs that many cyber security problems arise.
PI: Dr N Heard