Film based radiographic inspections can be very costly and has the risk of human exposure to ionising radiation. Therefore, industry tries to utilise computational tools as much as possible for each radiographic inspection to find the test configuration which leads to the best possible results.
The interpretation of a radiographic image is difficult and needs interpretation by a human expert to identify a damage signature. This presents a challenge to simulation tools when attempting to assess the ability to detect a defect within a simulated radiograph. In particular, the qualified expert’s defect detection ability investigating an actual film based image should be predicted by a computer model treating simulated images.
Techniques able to measure the defect detectability for image assessments are developed within this project. These techniques help to find the best radiographic test setup in regard to detectability. In order to improve the realistic appearance of the simulated radiographs, a second part of this project aims to improve the visual noise appearance of the simulated images. Film noise shows specific characteristics such as graininess being a visual obstacle and so influences the defect visibility. To make the simulation results closer to reality, this major influencer of the image quality should be considered and accurately simulated.
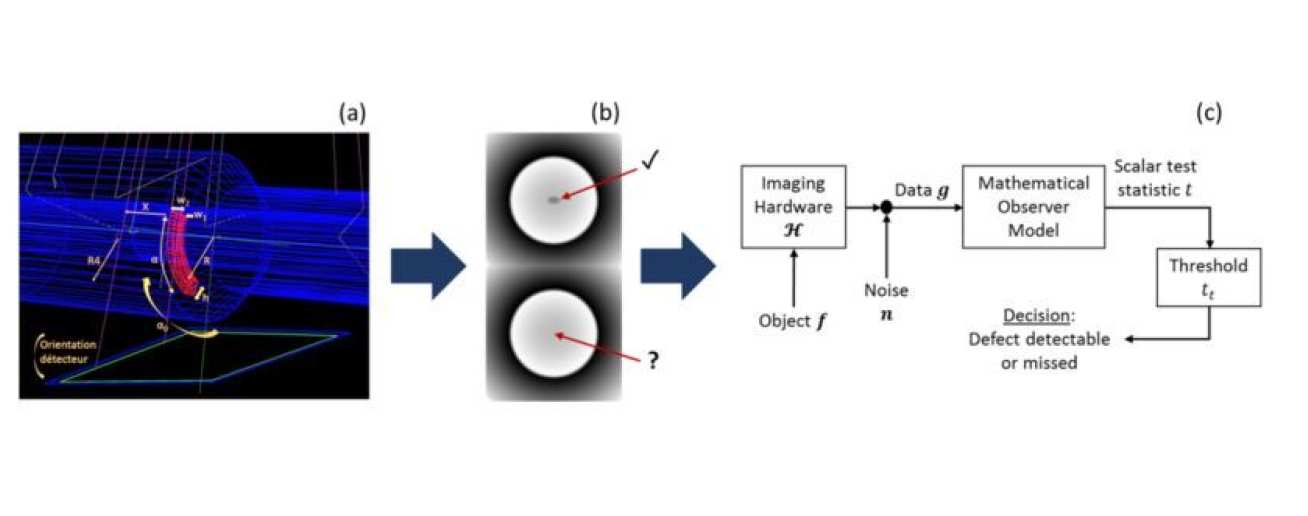
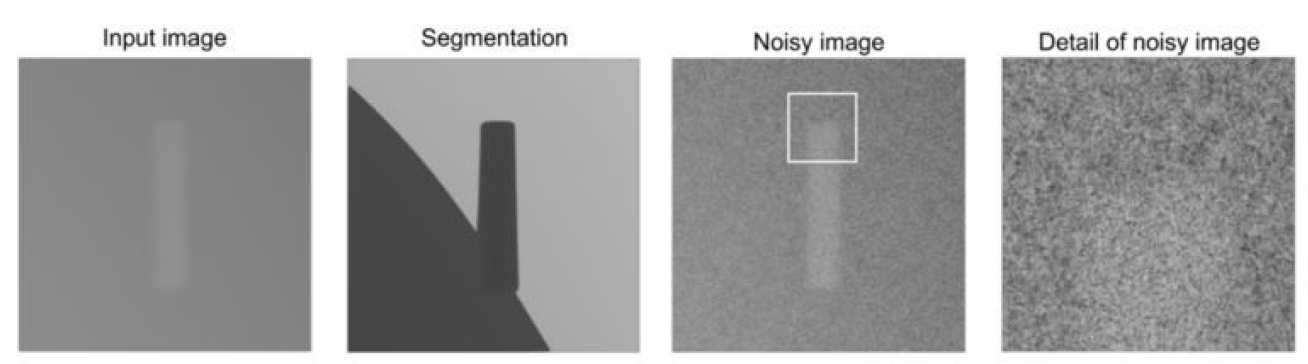