Fluorescent Penetrant Inspection (FPI) is a well-established NDT method used widely in the aerospace industry. The nature of FPI inspection, particularly in new manufacture, can lead to variable results influenced by human factors. This has led to the desire for an automated inspection system which would improve reliability, however, there is a lack of available training data. This, combined with accumulating surface penetrant due to roughness, geometry, insufficient wash off, etc. makes it challenging to train an automated inspection system capable of distinguishing penetrant which has arisen from defects from other non-defective indications.
A dataset of test pieces containing cracks induced using thermal fatigue was manufactured, processed and imaged. This dataset was used to train two Machine Learning (ML) algorithms: Random Forest and the ResNet Deep Learning architecture. Both methods demonstrated good performance, comparable to a human operator.
This project demonstrated the feasibility of using ML techniques to perform automated defect detection for FPI.
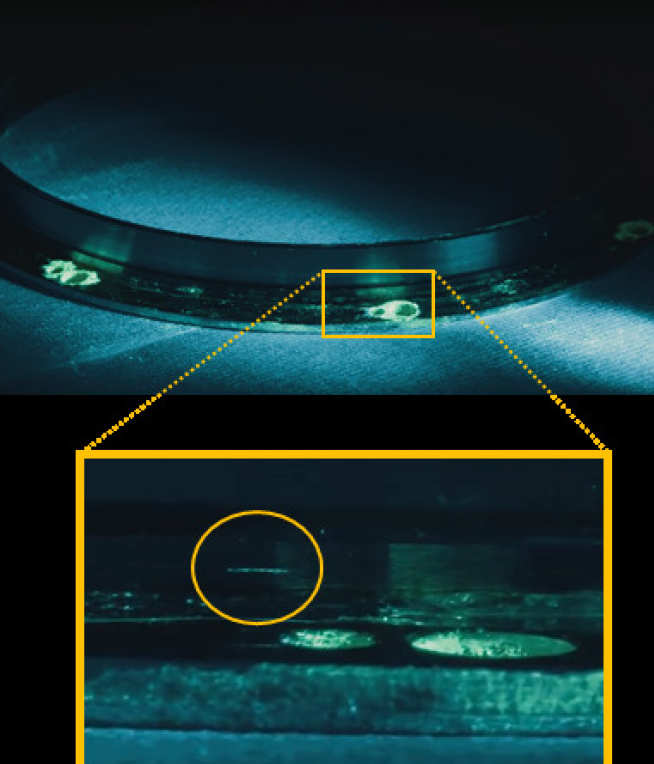