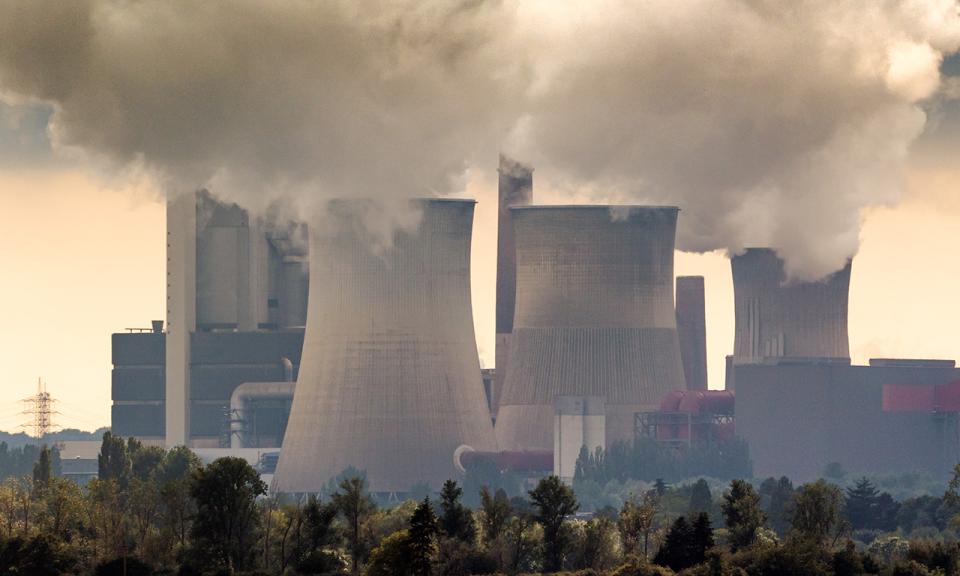
Uncertainty-aware planning and scheduling in the process industries
Led by the Department of Computing, this project examines process industries and will develop planning and scheduling tools for optimal decision-making under uncertainty while taking into account multiple time-scales. Expanding production and a growing global customer base can make planning and scheduling increasingly complex, particularly when dealing with uncertain factors such as volatile customer demand, variations in performance, and socio-economic fluctuation.
This research acknowledges the variation in the problems faced by individual firms, recognising that a single tool cannot address such specific problems, so we will develop a framework that classifies uncertainties by categories to provide optimal cutting-edge solutions. Simultaneously the research team will develop a hybrid uncertainty modelling and optimisation tool that offers synergies between various solution types. These will be tested on real-life case studies from sectors including energy systems, agro-chemicals, pharmaceuticals, consumer goods, oil & gas, and industrial gases.
The Business School will actively contribute to several work packages of this project, looking at foreseen uncertainties which existing optimisation models already account for. These include predictable outcomes such as demand for new products and changes in demand for existing products – this is known as stochastic and robust optimisation. Strategic, tactical and operational decisions affect a company’s future and all are heavily influenced by various sources of uncertainty. This research will assist firms in managing uncertainty and mitigate failure or severe under performance of entire production systems.
Key research questions:
- Can we integrate robust and stochastic policies into a single model to improve consistency? We propose a unified framework that incorporates both probabilistic and range-based uncertainties for Process Planning and Scheduling (PPS).
- How can discrete recourse decisions be modelled in robust optimisation problems? PPS problems often require additional distinct decisions, for example on investment or batch sequencing, so we consider how these can be predicted.
- What contribution can this work on decision-dependent uncertainty make to the robust optimisation literature?
Research partners
- Department of Computing
- Department of Chemical Engineering
- University College London
Funder: Engineering and Physical Sciences Research Council (EPSRC)
Duration: June 2015 – December 2019
Business school lead investigator: Dr Wolfram Wiesemann
Principal investigator: Dr Panos Parpas (Department of Computing)