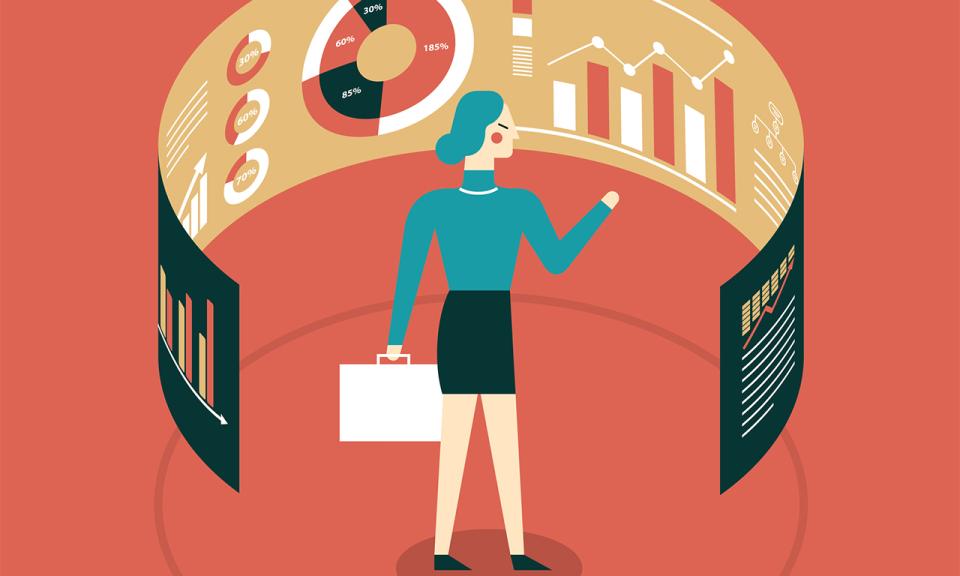
Alternative Data Ecosystem
With the growth of alternative datasets as an asset class and increased number of available datasets, a number of companies have sprung up offering a wide range of alternative data services, ranging from selling data and analysing it to helping with the challenges of running a successful alternative data function. Here are the main participants in the ever-evolving alt-data ecosystem (some participants might take multiple roles/services):
- Data Originators are entities that sell their own proprietary data to clients directly or sell it indirectly using data aggregators or data marketplaces. The datasets could either be a by-product of companies’ main functions (i.e. sales figures) or specifically sourced/collected by the companies (i.e. web-scraped data, enriched datasets, etc.). The biggest players in this category are Bloomberg, Refinitiv and S&P among others.
- Data Aggregators (or data marketplaces), as the name suggests, these collect and aggregate data from external sources. Some aggregators provide pre-processing of data, which make their use more appealing. Another advantage is having all the required data streamed through a single API. From an onboarding perspective, it is much simpler getting internal approval for a single aggregator rather than trying to bring on and implement data from several data vendors separately. Examples would be Bloomberg, Amazon and Quandl.
- Data Research firms help customers to understand what datasets are available, and assess potential uses and applications. They advise on which datasets might be the most appropriate given the problem the clients are trying to address. Usually this is done through giving clients access to web portals that contain research reports and additional information on a wide range of datasets. The leading players here are NeuData, BattleFin and EagleAlpha.
- Data Consultants provide similar services to data research entities where they act as bespoke advisors to clients on specific data questions they might have. For example, providing a general overview of alt data within specific industry, datasets used by competitors, implementing alt data infrastructure within organisations, etc. They also help companies identify, package and market in-house data that could be monetised including navigating privacy regulations.
Implementation Challenges
While there is certainly much to be gained from effective use of alternative data, it is also important to consider the sizeable challenges organisations might face and will need to address:
- Abundance of data might not sound like a problem (the more the better, right?!), however taking into account the cost and time it takes to analyse and find the suitable datasets means that it is extremely challenging to analyse more than a handful of datasets
- To fully benefit from new datasets, organisations have to have an agile in-house infrastructure in place. First of all, the dataset has to be efficiently sourced and the data provider on-boarded. Secondly, the majority of datasets come in an unstructured format and have to be pre-processed to be available for analysis. Finally, the organisation has to be able to store the data securely and in such a way as to ensure ease of experimentation and capability to plug the data into live models.
- Internal skills and resources that companies possess might not be appropriate to benefit fully from the use of alternative data. A recent survey by Lowenstein Sandler showed that a third of respondents highlighted the lack of required skills to analyse alternative datasets.
- Value assessment is also a huge barrier. In theory, you could plug the dataset (hopefully pre-processed by then) in your current models and do a back-test to see the impact of the new features. However, in practice, it’s not that easy. The majority of datasets do not have sufficient data history and/or current back-testing models are not set up to use the new types of data. Distribution shift is another big issue, where datasets could prove useful for certain economic regimes and not others.
- The lengthy search, on-boarding and analysis stages might also negatively impact potential usefulness of data. This is mainly as a result of short use-life of some datasets as once a dataset is proven to be useful, more and more market participants would implement the data in their processes and by doing that eroding overall competitive advantage the data brought in the first place.
- Finally, and perhaps the most significant barrier, is the cost of datasets. I know we have spoken about the abundance of datasets out there and in fact a large number of them are free (yes, free!). However, if you are after a dataset that has proven to generate alpha or/and you know that these datasets are successfully implemented by your competitors, in many cases you do not have a choice but to purchase the premium datasets. For these datasets, the prices could easily run in hundreds of thousand dollars, particularly in the area of equity or fixed income trading.
Conclusion
Despite the sizeable challenges outlined above, the alternative data still offers great potential, especially given the pace of current business environment. Therefore, I would strongly encourage companies to start exploring potential introduction of alternative data to their businesses process sooner rather than later.